- Terminology (A to D)
- AI Capability Control
- AIOps
- Albumentations
- Asset Performance
- Autoencoder
- Backpropagation
- Bayes Theorem
- Big Data
- Chatbot: A Beginner’s Guide
- Computational Thinking
- Computer Vision
- Confusion Matrix
- Convolutional Neural Networks
- Cybersecurity
- Data Fabric
- Data Storytelling
- Data Science
- Data Warehousing
- Decision Tree
- Deepfakes
- Deep Learning
- Deep Reinforcement Learning
- Devops
- DevSecOps
- Diffusion Models
- Digital Twin
- Dimensionality Reduction
- Terminology (E to K)
- Edge AI
- Emotion AI
- Ensemble Learning
- Ethical Hacking
- ETL
- Explainable AI
- Federated Learning
- FinOps
- Generative AI
- Generative Adversarial Network
- Generative vs. Discriminative
- Gradient Boosting
- Gradient Descent
- Few-Shot Learning
- Image Classification
- IT Operations (ITOps)
- Incident Automation
- Influence Engineering
- K-Means Clustering
- K-Nearest Neighbors
- Terminology (L to Q)
- Terminology (R to Z)
AI 101
What is Edge AI & Edge Computing?
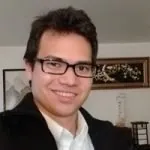
Table Of Contents
Edge AI is one of the most notable new sectors of artificial intelligence, and it aims to let people run AI processes without having to be concerned about privacy or slowdowns due to data transmission. Edge AI is enabling greater, more widespread use of AI, letting smart devices react quickly to inputs without access to a cloud. While that’s a quick definition of Edge AI, let’s take a moment to better understand Edge AI by exploring the technologies that make it possible and seeing some use cases for Edge AI.
What is Edge Computing?
In order to truly understand Edge AI, we need to first understand Edge computing, and the best way to understand Edge computing is to contrast it with cloud computing. Cloud computing is the delivery of computing services over the internet. In contrast, Edge computing systems are not connected to a cloud, instead of operating on local devices. These local devices can be a dedicated edge computing server, a local device, or an Internet of Things (IoT). There are a number of advantages to using Edge computing. For instance, internet/cloud-based computation is limited by latency and bandwidth, while Edge computing is not limited by these parameters.
What is Edge AI?
Now that we understand Edge computing we can take a look at Edge AI. Edge AI combines Artificial Intelligence and edge computing. The AI algorithms are run on devices capable of edge computing. The advantage of this is that the data can be processed in real-time, without having to connect to a cloud.
Most cutting edge AI processes are carried out in a cloud as they mandate a large amount of computing power. The result is that these AI processes can be vulnerable to downtime. Because Edge AI systems operate on an edge computing device, the necessary data operations can occur locally, being sent when an internet connection is established, which saves time. The deep learning algorithms can operate on the device itself, the origin point of the data.
Edge AI is becoming increasingly important due to the fact that more and more devices need to employ AI in situations where they cannot access the cloud. Consider how many factory robots or how many cars these days come with computer vision algorithms. A lag time in the transmission of data in these situations could be catastrophic. Self-driving cars cannot suffer from latency while detecting objects on the street. Since a quick response time is so important, the device itself must have an Edge AI system that allows it to analyze and classify images without relying on a cloud connection.
When edge computers are entrusted with the information processing tasks usually carried out on the cloud, the result is real-time low latency, real-time processing. Additionally, by restricting the transmission of data to just the most vital information, the data volume itself can be reduced and communication interruptions can be minimized.
Edge AI and the Internet of Things
Edge AI meshes with other digital technologies like 5G and the Internet of Things (IoT). IoT can generate data for Edge AI systems to make use of, while 5G technology is essential for the continued advancement of both Edge AI and IoT.
The Internet of Things refers to a variety of smart devices connected to one another through the internet. All of these devices generate data, which can be fed into the Edge AI device, which can also act as a temporary storage unit for the data until it is synced with the cloud. The method of data processing allows for greater flexibility.
The fifth generation of the mobile network, 5G, is critical for the development of both Edge AI and the Internet of Things. 5G is capable of transferring data at much higher speeds, up to 20Gbps, whereas 4G is capable of delivering data at only 1Gbps. 5G also supports far more simultaneous connections than 4G (1,000,000 per square kilometer vs. 100,000) and a better latency speed (1ms vs. 10ms). These advantages over 4G are important because as the IoT grows, data volume grows as well and transfer speed is impacted. 5G enables more interactions between a wider range of devices, many of which can be equipped with Edge AI.
Use Cases For Edge AI
Use cases for Edge AI include just about any instance where data processing would be done more efficiently on a local device than when done through a cloud. However, some of the most common use cases for Edge AI include self-driving cars, autonomous drones, facial recognition, and digital assistants.
Self-driving cars are one of the most relevant use cases for Edge AI. Self-driving cars must constantly be scanning the surrounding environment and assessing the situation, making corrections to its trajectory based on nearby events. Real-time data processing is critical for these cases, and as a result, their onboard Edge AI systems are in charge of the data storage, manipulation, and analysis. The edge AI systems are necessary to bring level 3 and level 4 (fully autonomous) vehicles to the market.
Because autonomous drones are not piloted by human operators, they have very similar requirements for autonomous cars. If a drone loses control or malfunctions while flying, it can crash and damage property or life. Drones may fly far out of range of an internet access point, and they must have Edge AI capabilities. Edge AI systems will be indispensable for services like Amazon Prime Air, which aims to deliver packages via drone.
Another use case for Edge AI is facial recognition systems. Facial recognition systems rely on computer vision algorithms, analyzing data collected by the camera. Facial recognition apps that operate for the purposes of tasks like security need to operate reliably even if they are not connected to a cloud.
Digital assistants are another common use case for Edge AI. Digital assistants like Google Assistant, Alexa, and Siri must be able to operate on smartphones and other digital devices even when they are not connected to the internet. When data is processed on the device there's no need to deliver it to the cloud, which helps reduce traffic and ensure privacy.
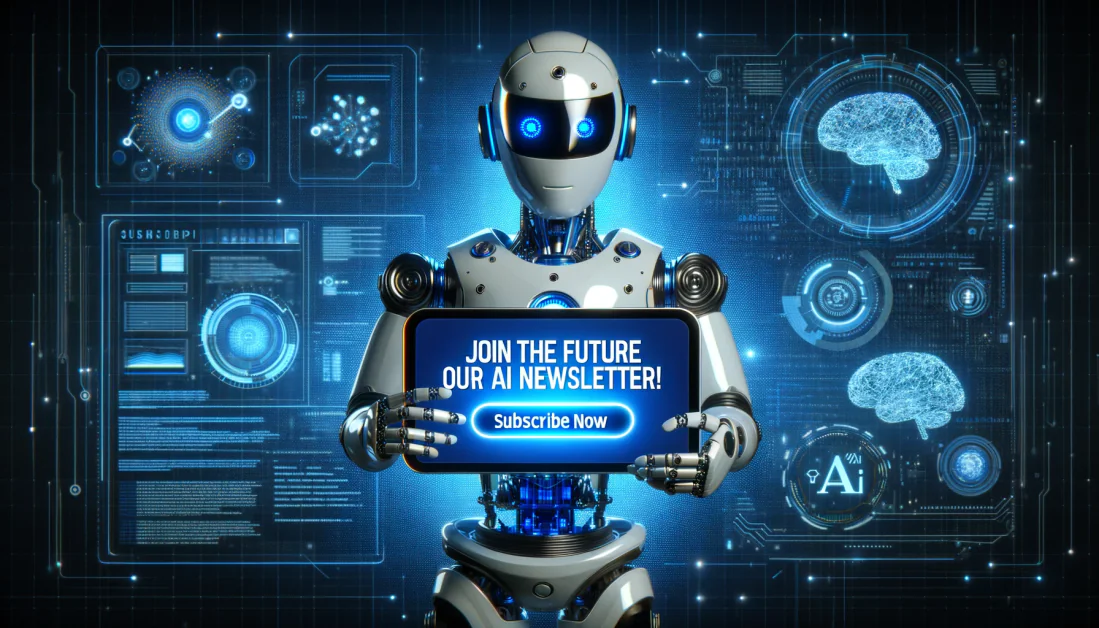
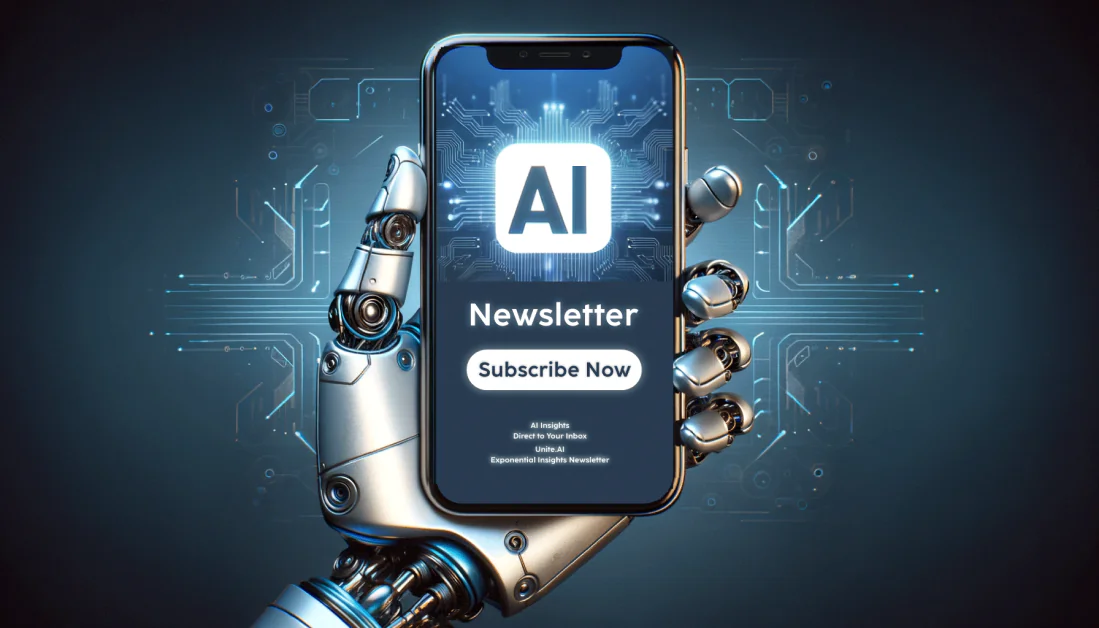
Blogger and programmer with specialties in Machine Learning and Deep Learning topics. Daniel hopes to help others use the power of AI for social good.
You may like
New Frontiers in Generative AI — Far From the Cloud
The Future of Generative AI Is the Edge
How AI, IoT & The Cloud are Keeping Fleet Vehicle Drivers Safer Today
How AI, Edge Computing, IoT & The Cloud are Drastically Reshaping Vehicle Fleet Management
Edge Computing vs. Cloud Computing: Major Differences
Advance in Microchips Brings Us Closer to AI Edge Computing