AI 101
What Are Deepfakes?
As deepfakes become easier to make and more prolific, more attention is paid to them. Deepfakes have become the focal point of discussions involving AI ethics, misinformation, openness of information and the internet, and regulation. It pays to be informed regarding deepfakes, and to have an intuitive understanding of what deepfakes are. This article will clarify the definition of a deepfake, examine their use cases, discuss how deepfakes can be detected, and examine the implications of deepfakes for society.
What are Deepfakes?
Before going on to discuss deepfakes further, it would be helpful to take some time and clarify what “deepfakes” actually are. There is a substantial amount of confusion regarding the term Deepfake, and often the term is misapplied to any falsified media, regardless of whether or not it is a genuine deepfake. In order to qualify as a Deepfake, the faked media in question must be generated with a machine-learning system, specifically a deep neural network.
The key ingredient of deepfakes is machine learning. Machine learning has made it possible for computers to automatically generate video and audio relatively quickly and easily. Deep neural networks are trained on footage of a real person in order for the network to learn how people look and move under the target environmental conditions. The trained network is then used on images of another individual and augmented with additional computer graphics techniques in order to combine the new person with the original footage. An encoder algorithm is used to determine the similarities between the original face and the target face. Once the common features of the faces have been isolated, a second AI algorithm called a decoder is used. The decoder examines the encoded (compressed) images and reconstructs them based off on the features in the original images. Two decoders are used, one on the original subject’s face and the second on the target person’s face. In order for the swap to be made, the decoder trained on images of person X is fed images of person Y. The result is that person Y’s face is reconstruction over Person X’s facial expressions and orientation.
Currently, it still takes a fair amount of time for a deepfake to be made. The creator of the fake has to spend a long time manually adjusting parameters of the model, as suboptimal parameters will lead to noticeable imperfections and image glitches that give away the fake’s true nature.
Although it’s frequently assumed that most deepfakes are made with a type of neural network called a generative adversarial network (GAN), many (perhaps most) deepfakes created these days do not rely on GANs. While GANs did play a prominent role in the creation of early deepfakes, most deepfake videos are created through alternative methods, according to Siwei Lyu from SUNY Buffalo.
It takes a disproportionately large amount of training data in order to train a GAN, and GANs often take much longer to render an image compared to other image generation techniques. GANs are also better for generating static images than video, as GANs have difficulties maintaining consistencies from frame to frame. It’s much more common to use an encoder and multiple decoders to create deepfakes.
What Are Deepfakes Used For?
Many of the deepfakes found online are pornographic in nature. According to research done by Deeptrace, an AI firm, out of a sample of approximately 15,000 deepfake videos taken in September of 2019, approximately 95% of them were pornographic in nature. A troubling implication of this fact is that as the technology becomes easier to use, incidents of fake revenge porn could rise.
However, not all deep fakes are pornographic in nature. There are more legitimate uses for deepfake technology. Audio deepfake technology could help people broadcast their regular voices after they are damaged or lost due to illness or injury. Deepfakes can also be used for hiding the faces of people who are in sensitive, potentially dangerous situations, while still allowing their lips and expressions to be read. Deepfake technology can potentially be used to improve the dubbing on foreign-language films, aid in the repair of old and damaged media, and even create new styles of art.
Non-Video Deepfakes
While most people think of fake videos when they hear the term “deepfake”, fake videos are by no means the only kind of fake media produced with deepfake technology. Deepfake technology is used to create photo and audio fakes as well. As previously mentioned, GANs are frequently used to generate fake images. It’s thought that there have been many cases of fake LinkedIn and Facebook profiles that have profile images generated with deepfake algorithms.
It’s possible to create audio deepfakes as well. Deep neural networks are trained to produce voice clones/voice skins of different people, including celebrities and politicians. One famous example of an audio Deepfake is when the AI company Dessa made use of an AI model, supported by non-AI algorithms, to recreate the voice of the podcast host Joe Rogan.
How To Spot Deepfakes
As deepfakes become more and more sophisticated, distinguishing them from genuine media will become tougher and tougher. Currently, there are a few telltale signs people can look for to ascertain if a video is potentially a deepfake, like poor lip-syncing, unnatural movement, flickering around the edge of the face, and warping of fine details like hair, teeth, or reflections. Other potential signs of a deepfake include lower-quality parts of the same video, and irregular blinking of the eyes.
While these signs may help one spot a deepfake at the moment, as deepfake technology improves the only option for reliable deepfake detection might be other types of AI trained to distinguish fakes from real media.
Artificial intelligence companies, including many of the large tech companies, are researching methods of detecting deepfakes. Last December, a deepfake detection challenge was started, supported by three tech giants: Amazon, Facebook, and Microsoft. Research teams from around the world worked on methods of detecting deepfakes, competing to develop the best detection methods. Other groups of researchers, like a group of combined researchers from Google and Jigsaw, are working on a type of “face forensics” that can detect videos that have been altered, making their datasets open source and encouraging others to develop deepfake detection methods. The aforementioned Dessa has worked on refining deepfake detection techniques, trying to ensure that the detection models work on deepfake videos found in the wild (out on the internet) rather than just on pre-composed training and testing datasets, like the open-source dataset Google provided.
There are also other strategies that are being investigated to deal with the proliferation of deepfakes. For instance, checking videos for concordance with other sources of information is one strategy. Searches can be done for video of events potentially taken from other angles, or background details of the video (like weather patterns and locations) can be checked for incongruities. Beyond this, a Blockchain online ledger system could register videos when they are initially created, holding their original audio and images so that derivative videos can always be checked for manipulation.
Ultimately, it’s important that reliable methods of detecting deepfakes are created and that these detection methods keep up with the newest advances in deepfake technology. While it is hard to know exactly what the effects of deepfakes will be, if there are not reliable methods of detecting deepfakes (and other forms of fake media), misinformation could potentially run rampant and degrade people’s trust in society and institutions.
Implications of Deepfakes
What are the dangers of allowing deep fake to proliferate unchecked?
One of the biggest problems that deepfakes create currently is nonconsensual pornography, engineered by combining people’s faces with pornographic videos and images. AI ethicists are worried that deepfakes will see more use in the creation of fake revenge porn. Beyond this, deepfakes could be used to bully and damage the reputation of just about anyone, as they could be used to place people into controversial and compromising scenarios.
Companies and cybersecurity specialists have expressed concern about the use of deepfakes to facilitate scams, fraud, and extortion. Allegedly, deepfake audio has been used to convince employees of a company to transfer money to scammers
It’s possible that deepfakes could have harmful effects even beyond those listed above. Deepfakes could potentially erode people’s trust in media generally, and make it difficult for people to distinguish between real news and fake news. If many videos on the web are fake, it becomes easier for governments, companies, and other entities to cast doubt on legitimate controversies and unethical practices.
When it comes to governments, deepfakes may even pose threats to the operation of democracy. Democracy requires that citizens are able to make informed decisions about politicians based on reliable information. Misinformation undermines democratic processes. For example, the president of Gabon, Ali Bongo, appeared in a video attempting to reassure the Gabon citizenry. The president was assumed to be unwell for long a long period of time, and his sudden appearance in a likely fake video kicked off an attempted coup. President Donald Trump claimed that an audio recording of him bragging about grabbing women by the genitals was fake, despite also describing it as “locker room talk”. Prince Andrew also claimed that an image provided by Emily Maitilis’ attorney was fake, though the attorney insisted on its authenticity.
Ultimately, while there are legitimate uses for deepfake technology, there are many potential harms that can arise from the misuse of that technology. For that reason, it’s extremely important that methods to determine the authenticity of media be created and maintained.
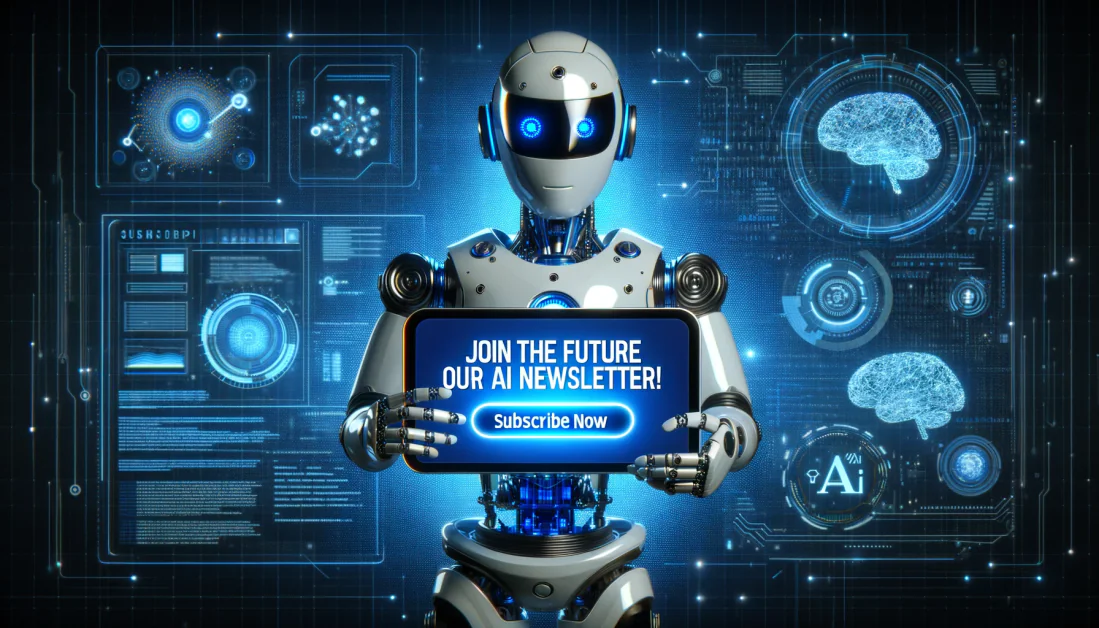
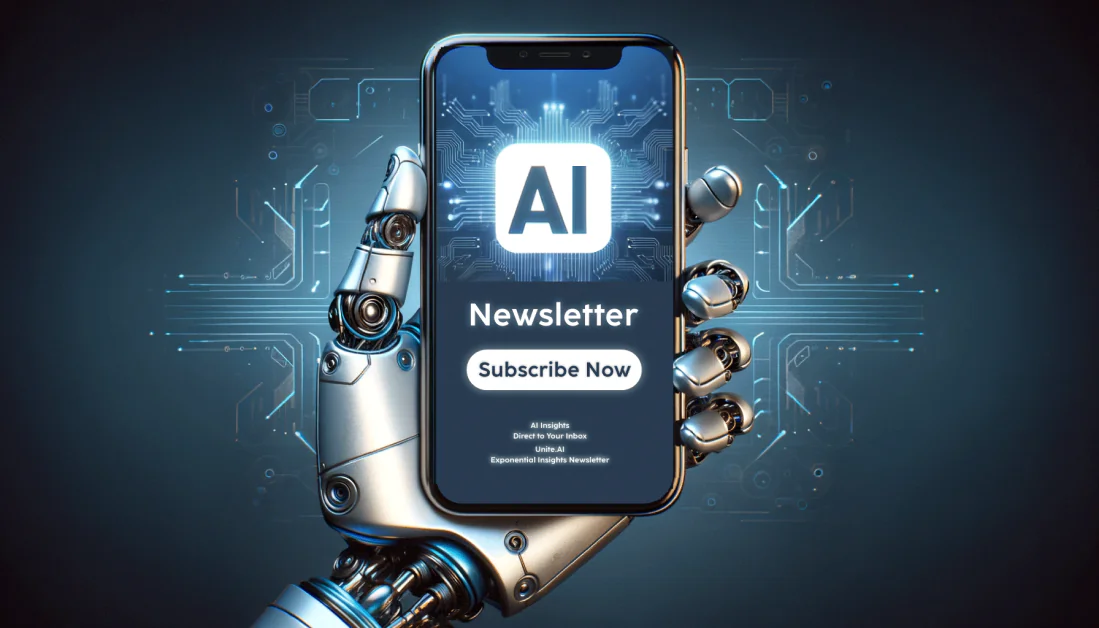