- Terminology (A to D)
- AI Capability Control
- AIOps
- Albumentations
- Asset Performance
- Autoencoder
- Backpropagation
- Bayes Theorem
- Big Data
- Chatbot: A Beginner’s Guide
- Computational Thinking
- Computer Vision
- Confusion Matrix
- Convolutional Neural Networks
- Cybersecurity
- Data Fabric
- Data Storytelling
- Data Science
- Data Warehousing
- Decision Tree
- Deepfakes
- Deep Learning
- Deep Reinforcement Learning
- Devops
- DevSecOps
- Diffusion Models
- Digital Twin
- Dimensionality Reduction
- Terminology (E to K)
- Edge AI
- Emotion AI
- Ensemble Learning
- Ethical Hacking
- ETL
- Explainable AI
- Federated Learning
- FinOps
- Generative AI
- Generative Adversarial Network
- Generative vs. Discriminative
- Gradient Boosting
- Gradient Descent
- Few-Shot Learning
- Image Classification
- IT Operations (ITOps)
- Incident Automation
- Influence Engineering
- K-Means Clustering
- K-Nearest Neighbors
- Terminology (L to Q)
- Terminology (R to Z)
AI 101
What is Deep Learning?
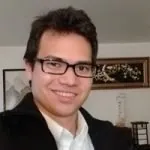
Table Of Contents
Deep learning is one of the most influential and fastest growing fields in artificial intelligence. However, getting an intuitive understanding of deep learning can be difficult because the term deep learning covers a variety of different algorithms and techniques. Deep learning is also a subdiscipline of machine learning in general, so it's important to understand what machine learning is in order to understand deep learning.
What is Machine Learning?
Deep learning is an extension of some of the concepts originating from machine learning, so for that reason, let’s take a minute to explain what machine learning is.
Put simply, machine learning is a method of enabling computers to carry out specific tasks without explicitly coding every line of the algorithms used to accomplish those tasks. There are many different machine learning algorithms, but one of the most commonly used algorithms is a multilayer perceptron. A multilayer perceptron is also referred to as a neural network, and it is comprised of a series of nodes/neurons linked together. There are three different layers in a multilayer perceptron: the input layer, the hidden layer, and the output layer.
The input layer takes the data into the network, where it is manipulated by the nodes in the middle/hidden layer. The nodes in the hidden layer are mathematical functions that can manipulate the data coming from the input layer, extracting relevant patterns from the input data. This is how the neural network “learns”. Neural networks get their name from the fact that they are inspired by the structure and function of the human brain.
The connections between nodes in the network have values called weights. These values are essentially assumptions about how the data in one layer is related to the data in the next layer. As the network trains the weights are adjusted, and the goal is that the weights/assumptions about the data will eventually converge on values that accurately represent the meaningful patterns within the data.
Activation functions are present in the nodes of the network, and these activation functions transform the data in a non-linear fashion, enabling the network to learn complex representations of the data. Activation functions multiply the input values by the weight values and add a bias term.
What is Deep Learning?
Deep learning is the term given to machine learning architectures that join many multilayer perceptrons together, so that there isn’t just one hidden layer but many hidden layers. The “deeper” that the deep neural network is, the more sophisticated patterns the network can learn.
The deep layer networks comprised of neurons are sometimes referred to as fully connected networks or fully connected layers, referencing the fact that a given neuron maintains a connection to all the neurons surrounding it. Fully connected networks can be combined with other machine learning functions to create different deep learning architectures.
Different Types of Deep Learning
There are a variety of deep learning architectures used by researchers and engineers, and each of the different architectures has its own specialty use case.
Convolutional Neural Networks
Convolutional neural networks, or CNNs, are the neural network architecture commonly used in the creation of computer vision systems. The structure of convolutional neural networks enables them to interpret image data, converting them into numbers that a fully connected network can interpret. A CNN has four major components:
- Convolutional layers
- Subsampling/pooling layers
- Activation functions
- Fully connected layers
The convolutional layers are what takes in the images as inputs into the network, analyzing the images and getting the values of the pixels. Subsampling or pooling is where the image values are converted/reduced to simplify the representation of the images and reduce the sensitivity of the image filters to noise. The activation functions control how the data flows from one layer to the next layer, and the fully connected layers are what analyze the values that represent the image and learn the patterns held in those values.
RNNs/LSTMs
Recurrent neural networks, or RNNs, are popular for tasks where the order of the data matters, where the network must learn about a sequence of data. RNNs are commonly applied to problems like natural language processing, as the order of words matters when decoding the meaning of a sentence. The “recurrent” part of the term Recurrent Neural Network comes from the fact that the output for a given element in a sequence in dependant on the previous computation as well as the current computation. Unlike other forms of deep neural networks, RNNs have “memories”, and the information calculated at the different time steps in the sequence is used to calculate the final values.
There are multiple types of RNNs, including bidirectional RNNs, which take future items in the sequence into account, in addition to the previous items, when calculating an item’s value. Another type of RNN is a Long Short-Term Memory, or LSTM, network. LSTMs are types of RNN that can handle long chains of data. Regular RNNs may fall victim to something called the “exploding gradient problem”. This issue occurs when the chain of input data becomes extremely long, but LSTMs have techniques to combat this problem.
Autoencoders
Most of the deep learning architectures mentioned so far are applied to supervised learning problems, rather than unsupervised learning tasks. Autoencoders are able to transform unsupervised data into a supervised format, allowing neural networks to be used on the problem.
Autoencoders are frequently used to detect anomalies in datasets, an example of unsupervised learning as the nature of the anomaly isn’t known. Such examples of anomaly detection include fraud detection for financial institutions. In this context, the purpose of an autoencoder is to determine a baseline of regular patterns in the data and identify anomalies or outliers.
The structure of an autoencoder is often symmetrical, with hidden layers arrayed such that the output of the network resembles the input. The four types of autoencoders that see frequent use are:
- Regular/plain autoencoders
- Multilayer encoders
- Convolutional encoders
- Regularized encoders
Regular/plain autoencoders are just neural nets with a single hidden layer, while multilayer autoencoders are deep networks with more than one hidden layer. Convolutional autoencoders use convolutional layers instead of, or in addition to, fully-connected layers. Regularized autoencoders use a specific kind of loss function that lets the neural network carry out more complex functions, functions other than just copying inputs to outputs.
Generative Adversarial Networks
Generative Adversarial Networks (GANs) are actually multiple deep neural networks instead of just one network. Two deep learning models are trained at the same time, and their outputs are fed to the other network. The networks are in competition with each other, and since they get access to each other's output data, they both learn from this data and improve. The two networks are essentially playing a game of counterfeit and detection, where the generative model tries to create new instances that will fool the detective model/the discriminator. GANs have become popular in the field of computer vision.
Deep Learning Summary
Deep learning extends the principles of neural networks to create sophisticated models that can learn complex patterns and generalize those patterns to future datasets. Convolutional neural networks are used to interpret images, while RNNs/LSTMs are used to interpret sequential data. Autoencoders can transform unsupervised learning tasks into supervised learning tasks. Finally, GANs are multiple networks pitted against each other that are especially useful for computer vision tasks.
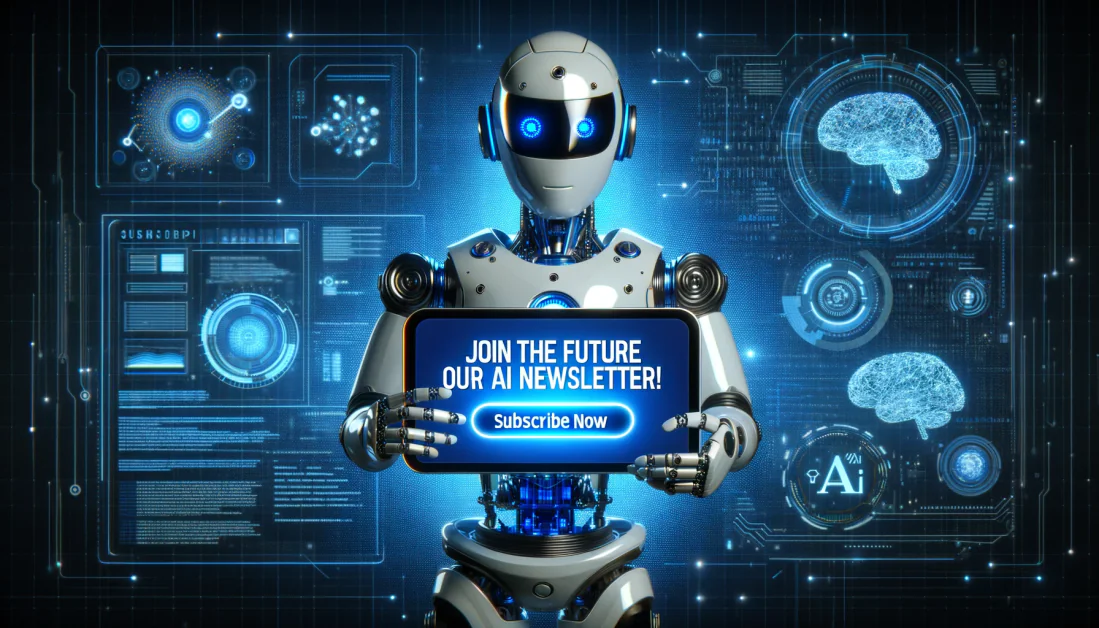
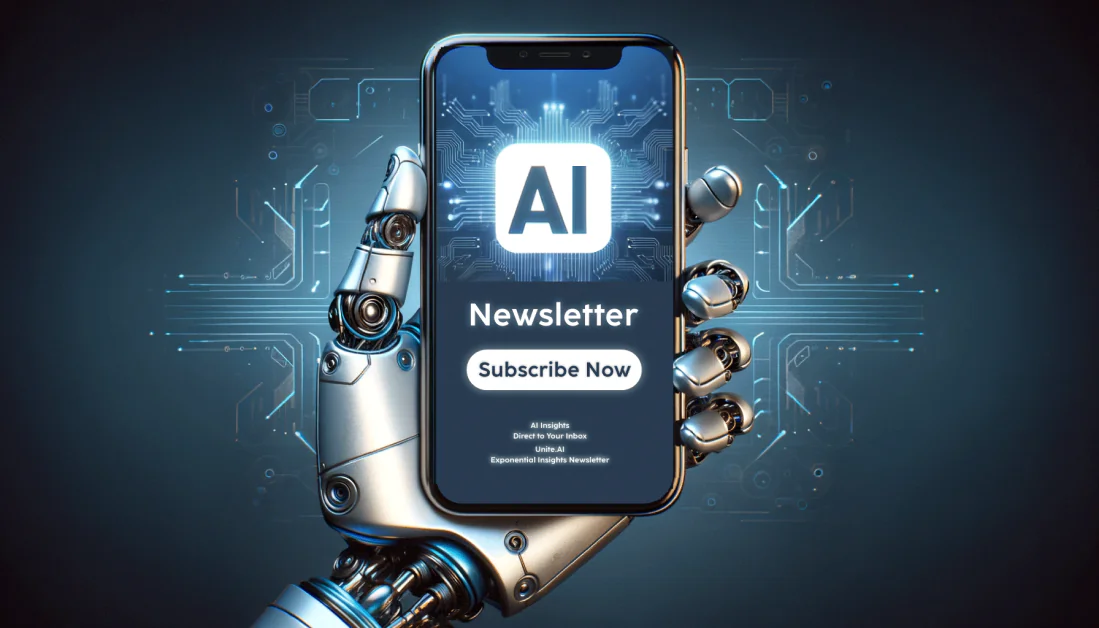
Blogger and programmer with specialties in Machine Learning and Deep Learning topics. Daniel hopes to help others use the power of AI for social good.
You may like
Multilingual AI on Google Cloud: The Global Reach of Meta’s Llama 3.1 Models
Scientists Develop ‘Material Fingerprinting’ Method Using AI and X-ray Technology
Top 5 AI Hallucination Detection Solutions
Beyond Scripts: The Future of Video Game NPCs with Generative AI
Pioneering Open Models: Nvidia, Alibaba, and Stability AI Transforming the AI Landscape
Camera System Mimics Human Eye for Enhanced Robotic Vision