- Terminology (A to D)
- AI Capability Control
- AIOps
- Albumentations
- Asset Performance
- Autoencoder
- Backpropagation
- Bayes Theorem
- Big Data
- Chatbot: A Beginner’s Guide
- Computational Thinking
- Computer Vision
- Confusion Matrix
- Convolutional Neural Networks
- Cybersecurity
- Data Fabric
- Data Storytelling
- Data Science
- Data Warehousing
- Decision Tree
- Deepfakes
- Deep Learning
- Deep Reinforcement Learning
- Devops
- DevSecOps
- Diffusion Models
- Digital Twin
- Dimensionality Reduction
- Terminology (E to K)
- Edge AI
- Emotion AI
- Ensemble Learning
- Ethical Hacking
- ETL
- Explainable AI
- Federated Learning
- FinOps
- Generative AI
- Generative Adversarial Network
- Generative vs. Discriminative
- Gradient Boosting
- Gradient Descent
- Few-Shot Learning
- Image Classification
- IT Operations (ITOps)
- Incident Automation
- Influence Engineering
- K-Means Clustering
- K-Nearest Neighbors
- Terminology (L to Q)
- Terminology (R to Z)
AI 101
What is Reinforcement Learning From Human Feedback (RLHF)
Published
1 year agoon
Table Of Contents
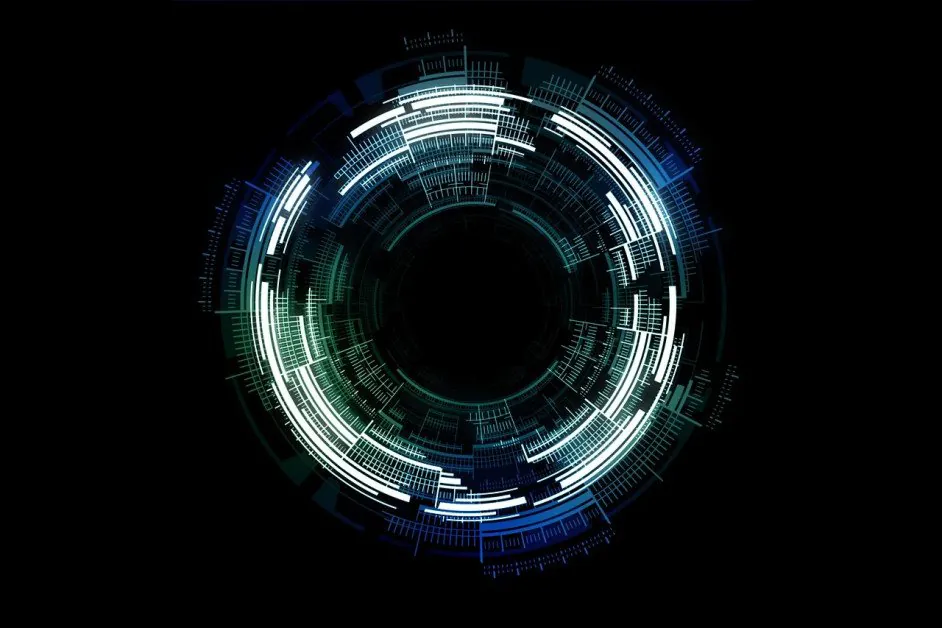
In the constantly evolving world of artificial intelligence (AI), Reinforcement Learning From Human Feedback (RLHF) is a groundbreaking technique that has been used to develop advanced language models like ChatGPT and GPT-4. In this blog post, we will dive into the intricacies of RLHF, explore its applications, and understand its role in shaping the AI systems that power the tools we interact with daily.
Reinforcement Learning From Human Feedback (RLHF) is an advanced approach to training AI systems that combines reinforcement learning with human feedback. It is a way to create a more robust learning process by incorporating the wisdom and experience of human trainers in the model training process. The technique involves using human feedback to create a reward signal, which is then used to improve the model's behavior through reinforcement learning.
Reinforcement learning, in simple terms, is a process where an AI agent learns to make decisions by interacting with an environment and receiving feedback in the form of rewards or penalties. The agent's goal is to maximize the cumulative reward over time. RLHF enhances this process by replacing, or supplementing, the predefined reward functions with human-generated feedback, thus allowing the model to better capture complex human preferences and understandings.
How RLHF Works
The process of RLHF can be broken down into several steps:
- Initial model training: In the beginning, the AI model is trained using supervised learning, where human trainers provide labeled examples of correct behavior. The model learns to predict the correct action or output based on the given inputs.
- Collection of human feedback: After the initial model has been trained, human trainers are involved in providing feedback on the model's performance. They rank different model-generated outputs or actions based on their quality or correctness. This feedback is used to create a reward signal for reinforcement learning.
- Reinforcement learning: The model is then fine-tuned using Proximal Policy Optimization (PPO) or similar algorithms that incorporate the human-generated reward signals. The model continues to improve its performance by learning from the feedback provided by the human trainers.
- Iterative process: The process of collecting human feedback and refining the model through reinforcement learning is repeated iteratively, leading to continuous improvement in the model's performance.
RLHF in ChatGPT and GPT-4
ChatGPT and GPT-4 are state-of-the-art language models developed by OpenAI that have been trained using RLHF. This technique has played a crucial role in enhancing the performance of these models and making them more capable of generating human-like responses.
In the case of ChatGPT, the initial model is trained using supervised fine-tuning. Human AI trainers engage in conversations, playing both the user and AI assistant roles, to generate a dataset that represents diverse conversational scenarios. The model then learns from this dataset by predicting the next appropriate response in the conversation.
Next, the process of collecting human feedback begins. AI trainers rank multiple model-generated responses based on their relevance, coherence, and quality. This feedback is converted into a reward signal, and the model is fine-tuned using reinforcement learning algorithms.
GPT-4, an advanced version of its predecessor GPT-3, follows a similar process. The initial model is trained using a vast dataset containing text from diverse sources. Human feedback is then incorporated during the reinforcement learning phase, helping the model capture subtle nuances and preferences that are not easily encoded in predefined reward functions.
Benefits of RLHF in AI Systems
RLHF offers several advantages in the development of AI systems like ChatGPT and GPT-4:
- Improved performance: By incorporating human feedback into the learning process, RLHF helps AI systems better understand complex human preferences and produce more accurate, coherent, and contextually relevant responses.
- Adaptability: RLHF enables AI models to adapt to different tasks and scenarios by learning from human trainers' diverse experiences and expertise. This flexibility allows the models to perform well in various applications, from conversational AI to content generation and beyond.
- Reduced biases: The iterative process of collecting feedback and refining the model helps address and mitigate biases present in the initial training data. As human trainers evaluate and rank the model-generated outputs, they can identify and address undesirable behavior, ensuring that the AI system is more aligned with human values.
- Continuous improvement: The RLHF process allows for continuous improvement in model performance. As human trainers provide more feedback and the model undergoes reinforcement learning, it becomes increasingly adept at generating high-quality outputs.
- Enhanced safety: RLHF contributes to the development of safer AI systems by allowing human trainers to steer the model away from generating harmful or unwanted content. This feedback loop helps ensure that AI systems are more reliable and trustworthy in their interactions with users.
Challenges and Future Perspectives
While RLHF has proven effective in improving AI systems like ChatGPT and GPT-4, there are still challenges to overcome and areas for future research:
- Scalability: As the process relies on human feedback, scaling it to train larger and more complex models can be resource-intensive and time-consuming. Developing methods to automate or semi-automate the feedback process could help address this issue.
- Ambiguity and subjectivity: Human feedback can be subjective and may vary between trainers. This can lead to inconsistencies in the reward signals and potentially impact model performance. Developing clearer guidelines and consensus-building mechanisms for human trainers may help alleviate this problem.
- Long-term value alignment: Ensuring that AI systems remain aligned with human values in the long term is a challenge that needs to be addressed. Continuous research in areas like reward modeling and AI safety will be crucial in maintaining value alignment as AI systems evolve.
RLHF is a transformative approach in AI training that has been pivotal in the development of advanced language models like ChatGPT and GPT-4. By combining reinforcement learning with human feedback, RLHF enables AI systems to better understand and adapt to complex human preferences, leading to improved performance and safety. As the field of AI continues to progress, it is crucial to invest in further research and development of techniques like RLHF to ensure the creation of AI systems that are not only powerful but also aligned with human values and expectations.
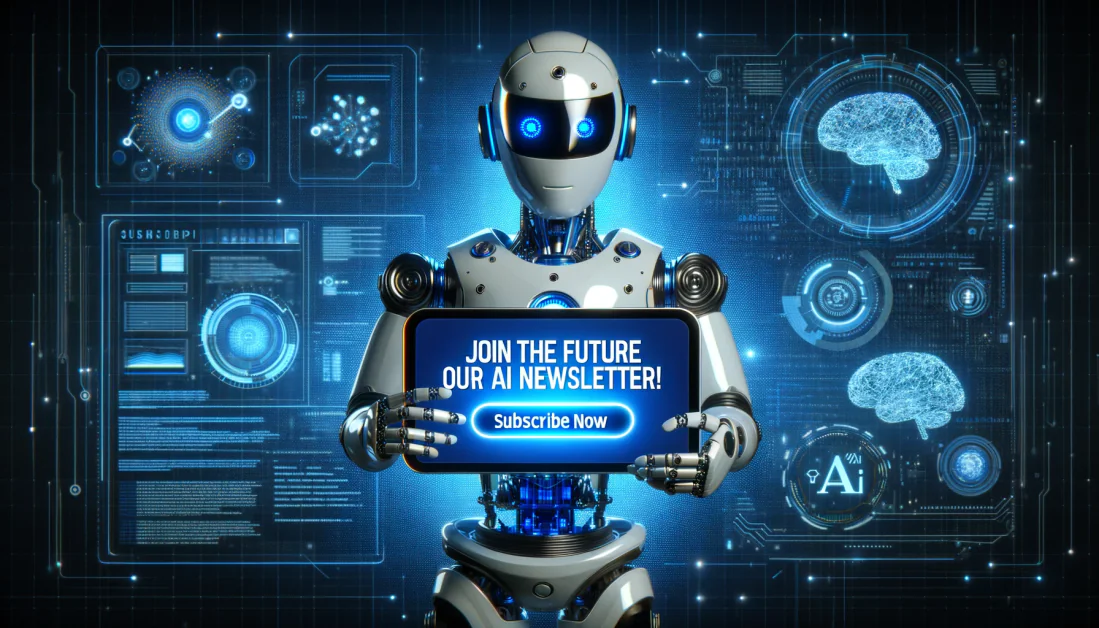
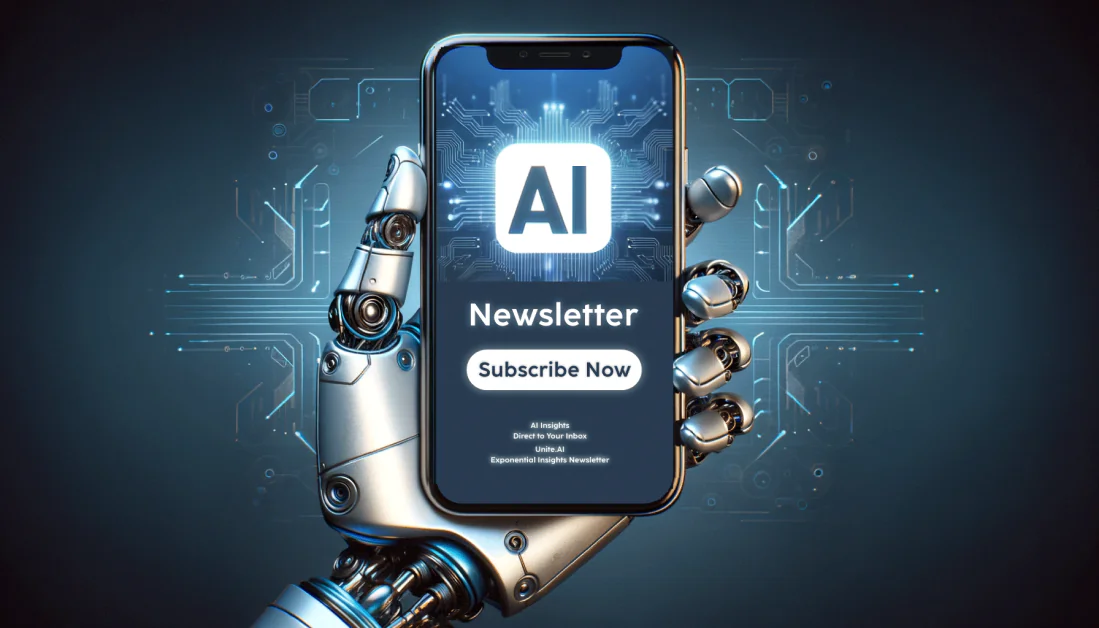
Alex McFarland is an AI journalist and writer exploring the latest developments in artificial intelligence. He has collaborated with numerous AI startups and publications worldwide.