- Terminology (A to D)
- AI Capability Control
- AIOps
- Albumentations
- Asset Performance
- Autoencoder
- Backpropagation
- Bayes Theorem
- Big Data
- Chatbot: A Beginner’s Guide
- Computational Thinking
- Computer Vision
- Confusion Matrix
- Convolutional Neural Networks
- Cybersecurity
- Data Fabric
- Data Storytelling
- Data Science
- Data Warehousing
- Decision Tree
- Deepfakes
- Deep Learning
- Deep Reinforcement Learning
- Devops
- DevSecOps
- Diffusion Models
- Digital Twin
- Dimensionality Reduction
- Terminology (E to K)
- Edge AI
- Emotion AI
- Ensemble Learning
- Ethical Hacking
- ETL
- Explainable AI
- Federated Learning
- FinOps
- Generative AI
- Generative Adversarial Network
- Generative vs. Discriminative
- Gradient Boosting
- Gradient Descent
- Few-Shot Learning
- Image Classification
- IT Operations (ITOps)
- Incident Automation
- Influence Engineering
- K-Means Clustering
- K-Nearest Neighbors
- Terminology (L to Q)
- Terminology (R to Z)
AI 101
What is Meta-Learning?
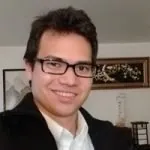
Table Of Contents
What is Meta-Learning?
One of the fastest-growing areas of research in machine learning is the area of meta-learning. Meta-learning, in the machine learning context, is the use of machine learning algorithms to assist in the training and optimization of other machine learning models. As meta-learning is becoming more and more popular and more meta-learning techniques are being developed, it’s beneficial to have an understanding of what meta-learning is and to have a sense of the various ways it can be applied. Let’s examine the ideas behind meta-learning, types of meta-learning, as well as some of the ways meta-learning can be used.
The term meta-learning was coined by Donald Maudsley to describe a process by which people begin to shape what they learn, becoming “increasingly in control of habits of perception, inquiry, learning, and growth that they have internalized”. Later, cognitive scientists and psychologists would describe meta-learning as “learning how to learn”.
For the machine learning version of meta-learning, the general idea of “learning how to learn” is applied to AI systems. In the AI sense, meta-learning is the ability of an artificially intelligent machine to learn how to carry out various complex tasks, taking the principles it used to learn one task and applying it to other tasks. AI systems typically have to be trained to accomplish a task through the mastering of many small subtasks. This training can take a long time and AI agents don’t easily transfer the knowledge learned during one task to another task. Creating meta-learning models and techniques can help AI learn to generalize learning methods and acquire new skills quicker.
Types of Meta-Learning
Optimizer Meta-Learning
Meta-learning is often employed to optimize the performance of an already existing neural network. Optimizer meta-learning methods typically function by tweaking the hyperparameters of a different neural network in order to improve the performance of the base neural network. The result is that the target network should become better at performing the task it is being trained on. One example of a meta-learning optimizer is the use of a network to improve gradient descent results.
Few-Shots Meta-Learning
A few-shots meta-learning approach is one where a deep neural network is engineered which is capable of generalizing from the training datasets to unseen datasets. An instance of few-shot classification is similar to a normal classification task, but instead, the data samples are entire datasets. The model is trained on many different learning tasks/datasets and then it’s optimized for peak performance on the multitude of training tasks and unseen data. In this approach, a single training sample is split up into multiple classes. This means that each training sample/dataset could potentially be made up of two classes, for a total of 4-shots. In this case, the total training task could be described as a 4-shot 2-class classification task.
In few-shot learning, the idea is that the individual training samples are minimalistic and that the network can learn to identify objects after having seen just a few pictures. This is much like how a child learns to distinguish objects after seeing just a couple of pictures. This approach has been used to create techniques like one-shot generative models and memory augmented neural networks.
Metric Meta-Learning
Metric based meta-learning is the utilization of neural networks to determine if a metric is being used effectively and if the network or networks are hitting the target metric. Metric meta-learning is similar to few-shot learning in that just a few examples are used to train the network and have it learn the metric space. The same metric is used across the diverse domain and if the networks diverge from the metric they are considered to be failing.
Recurrent Model Meta-Learning
Recurrent model meta-learning is the application of meta-learning techniques to Recurrent Neural Networks and the similar Long Short-Term Memory networks. This technique operates by training the RNN/LSTM model to sequentially learn a dataset and then using this trained model as a basis for another learner. The meta-learner takes on board the specific optimization algorithm that was used to train the initial model. The inherited parameterization of the meta-learner enables it to quickly initialize and converge, but still be able to update for new scenarios.
How Does Meta-Learning Work?
The exact way that meta-learning is conducted varies depending on the model and the nature of the task at hand. However, in general, a meta-learning task involves copying over the parameters of the first network into the parameters of the second network/the optimizer.
There are two training processes in meta-learning. The meta-learning model is typically trained after several steps of training on the base model have been carried out. After the forward, backward, and optimization steps that train the base model, the forward training pass is carried out for the optimization model. For example, after three or four steps of training on the base model, a meta-loss is computed. After the meta-loss is computed, the gradients are computed for each meta-parameter. After this occurs, the meta-parameters in the optimizer are updated.
One possibility for calculating the meta-loss is to finish the forward training pass of the initial model and then combine the losses that have already been computed. The meta-optimizer could even be another meta-learner, though at a certain point a discrete optimizer like ADAM or SGD must be used.
Many deep learning models can have hundreds of thousands or even millions of parameters. Creating a meta-learner that has an entirely new set of parameters would be computationally expensive, and for this reason, a tactic called coordinate-sharing is typically used. Coordinate-sharing involves engineering the meta-learner/optimizer so that it learns a single parameter from the base model and then just clones that parameter in place of all of the other parameters. The result is that the parameters the optimizer possesses don’t depend on the parameters of the model.
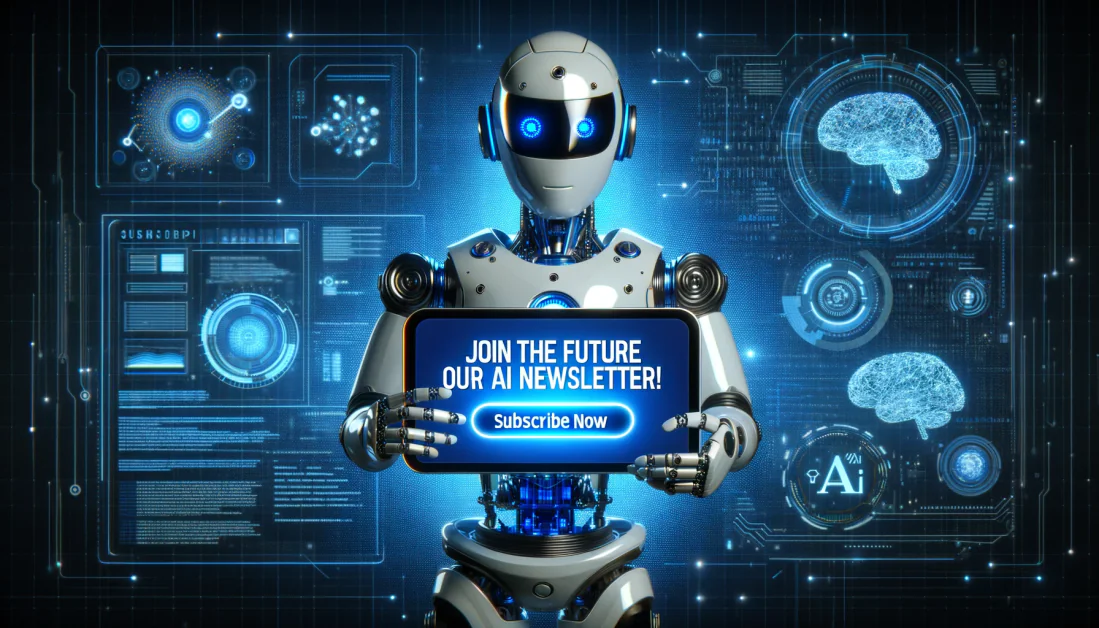
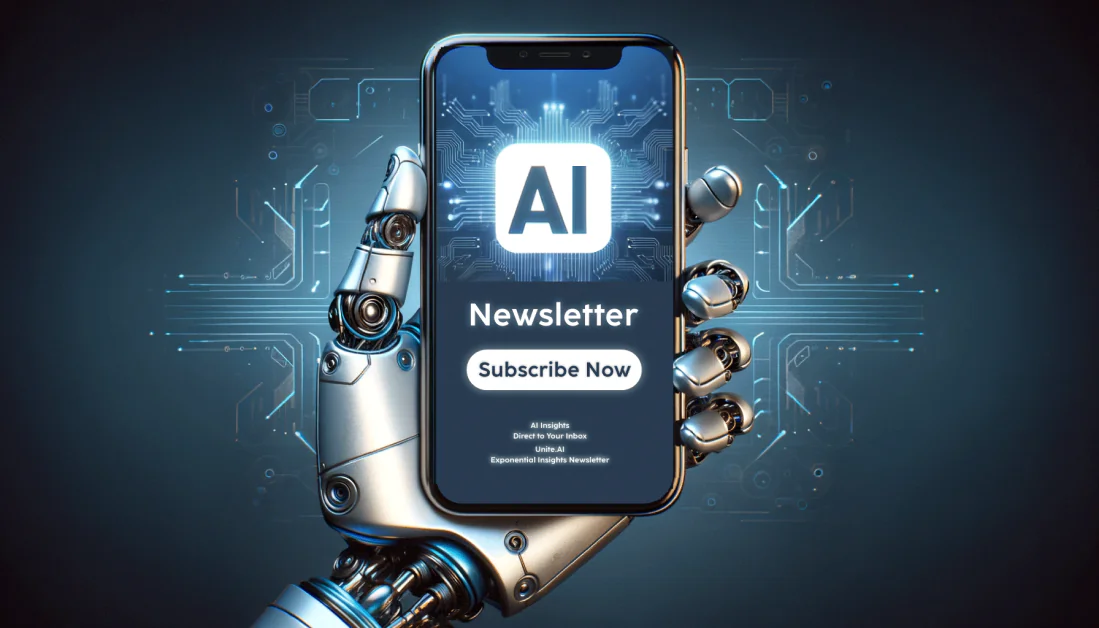
Blogger and programmer with specialties in Machine Learning and Deep Learning topics. Daniel hopes to help others use the power of AI for social good.