- Terminology (A to D)
- AI Capability Control
- AIOps
- Albumentations
- Asset Performance
- Autoencoder
- Backpropagation
- Bayes Theorem
- Big Data
- Chatbot: A Beginner’s Guide
- Computational Thinking
- Computer Vision
- Confusion Matrix
- Convolutional Neural Networks
- Cybersecurity
- Data Fabric
- Data Storytelling
- Data Science
- Data Warehousing
- Decision Tree
- Deepfakes
- Deep Learning
- Deep Reinforcement Learning
- Devops
- DevSecOps
- Diffusion Models
- Digital Twin
- Dimensionality Reduction
- Terminology (E to K)
- Edge AI
- Emotion AI
- Ensemble Learning
- Ethical Hacking
- ETL
- Explainable AI
- Federated Learning
- FinOps
- Generative AI
- Generative Adversarial Network
- Generative vs. Discriminative
- Gradient Boosting
- Gradient Descent
- Few-Shot Learning
- Image Classification
- IT Operations (ITOps)
- Incident Automation
- Influence Engineering
- K-Means Clustering
- K-Nearest Neighbors
- Terminology (L to Q)
- Terminology (R to Z)
AI 101
How Does Text Classification Work?
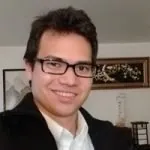
Table Of Contents
Text classification is the process of analyzing text sequences and assigning them a label, putting them in a group based on their content. Text classification underlies almost any AI or machine learning task involving Natural Language Processing (NLP). With text classification, a computer program can carry out a wide variety of different tasks like spam recognition, sentiment analysis, and chatbot functions. How does text classification work exactly? What are the different methods of carrying out text classification? We’ll explore the answers to these questions below.
Defining Text Classification
It’s important to take some time and make sure that we understand what text classification is, in general, before delving into the different methods of doing text classification. Text classification is one of those terms that is applied to many different tasks and algorithms, so it's useful to make sure that we understand the basic concept of text classification before moving on to explore the different ways that it can be carried out.
Anything that involves creating different categories for text, and then labeling different text samples as these categories, can be considered text classification. As long as a system carries out these basic steps it can be considered a text classifier, regardless of the exact method used to classify the text and regardless of how the text classifier is eventually applied. Detecting email spam, organizing documents by topic or title, and recognizing the sentiment of a review for a product are all examples of text classification because they are accomplished by taking text as an input and outputting a class label for that piece of text.
How Does Text Classification Work?
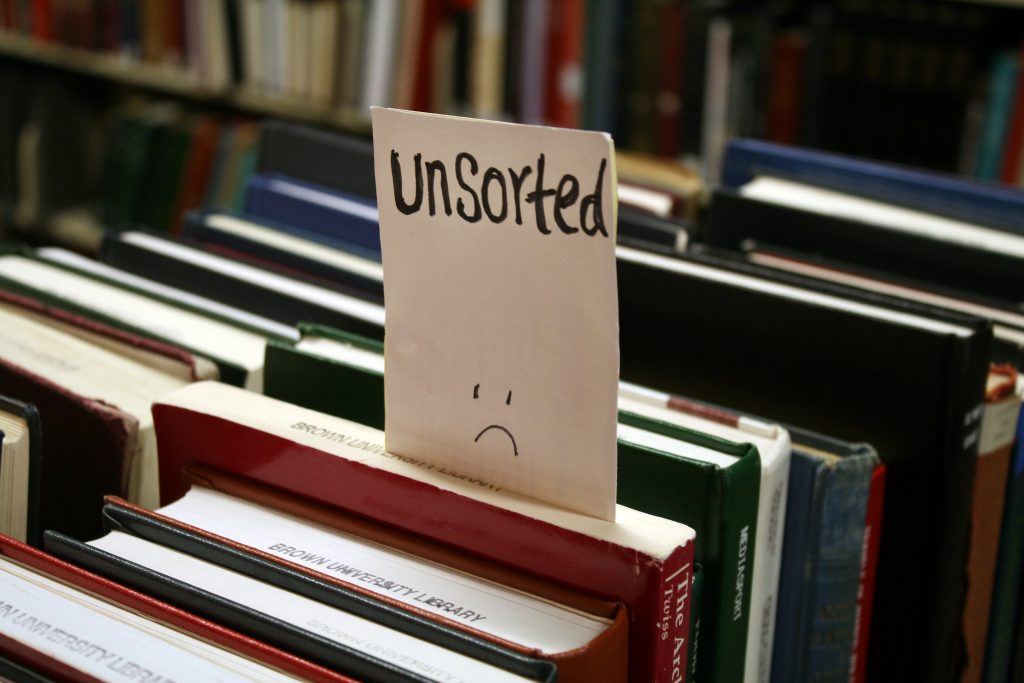
Photo: Quinn Dombrowski via Flickr, CC BY SA 2.0 , (https://www.flickr.com/photos/quinnanya/4714794045)
Most text classification methods can be placed into one of three different categories: rule-based methods or machine learning methods.
Rule-Based Classification Methods
Rule-based text classification methods operate through the use of explicitly engineered linguistic rules. The system uses the rules created by the engineer to determine which class a given piece of text should belong to, looking for clues in the form of semantically relevant text elements. Every rule has a pattern that the text must match to be placed into the corresponding category.
To be more concrete, let’s say you wanted to design a text classifier capable of distinguishing common topics of conversation, like the weather, movies, or food. In order to enable your text classifier to recognize discussion of the weather, you might tell it to look for weather-related words in the body of the text samples it is being fed. You’d have a list of keywords, phrases, and other relevant patterns that could be used to distinguish the topic. For instance, you might instruct the classifier to look for words like “wind”, “rain”, “sun”, “snow”, or “cloud”. You could then have the classifier look through input text and count the number of times that these words appear in the body of the text and if they appear more commonly than words related to movies, you would classify the text as belonging to the weather class.
The advantage of rules-based systems is that their inputs and outputs are predictable and interpretable by humans, and they can be improved through manual intervention by the engineer. However, rules-based classification methods are also somewhat brittle, and they often have a difficult time generalizing because they can only adhere to the predefined patterns that have been programmed in. As an example, the word “cloud” could refer to moisture in the sky, or it could be referring to a digital cloud where data is stored. It’s difficult for rules-based systems to handle these nuances without the engineers spending a fair amount of time trying to manually anticipate and adjust for these subtleties.
Machine Learning Systems
As mentioned above, rules-based systems have limitations, as their functions and rules must be pre-programmed. By contrast, machine learning-based classification systems operate by applying algorithms that analyze datasets for patterns that are associated with a particular class.
Machine learning algorithms are fed pre-labeled/pre-classified instances that are analyzed for relevant features. These pre-labeled instances are the training data.
The machine learning classifier analyzes the training data and learns patterns that are associated with the different classes. After this, unseen instances are stripped of their labels and fed to the classification algorithm which assigns the instances a label. The assigned labels are then compared to the original labels to see how accurate the machine learning classifier was, gauging how well the model learned what patterns predict which classes.
Machine learning algorithms operate by analyzing numerical data. This means that in order to use a machine learning algorithm on text data, the text needs to be converted into a numerical format. There are various methods of encoding text data as numerical data and creating machine learning methods around this data. We’ll cover some of the different ways to represent text data below.
Bag-of-Words
Bag-of-words is one of the most commonly used approaches for encoding and representing text data. The term “bag-of-words” comes from the fact that you essentially take all the words in the documents and put them all into one “bag” without paying attention to word order or grammar, paying attention only to the frequency of words in the bag. This results in a long array, or vector, containing a single representation of all the words in the input documents. So if there are 10000 unique words total in the input documents, the feature vectors will be 10000 words long. This is how the size of the word bag/feature vector is calculated.
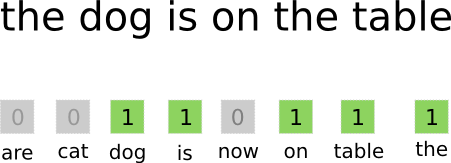
Photo: gk_ via Machinelearning.co, (https://machinelearnings.co/text-classification-using-neural-networks-f5cd7b8765c6)
After the feature vector size has been determined, every document in the list of total documents is assigned its own vector filled with numbers that indicate how many times the word in question appears in the current document. This means that if the word “food” appears eight times within one text document, that corresponding feature vector/feature array will have an eight in the corresponding position.
Put another way, all the unique words that appear in the input documents are all piled into one bag and then each document gets a word vector of the same size, which is then filled in with the number of times the different words appear in the document.
Text datasets will often contain a large number of unique words, but most of them aren’t used very frequently. For this reason, the number of words used to create the word vector is typically capped at a chosen value (N) and then the feature vector dimension will be Nx1.
Term Frequency-Inverse Document Frequency (TF-IDF)
Another way to represent a document based on the words in it is dubbed Term Frequency-Inverse Document Frequency (TF-IDF). A TF-IDF approach also creates a vector that represents the document based on the words in it, but unlike Bag-of-words these words are weighted by more than just their frequency. TF-IDF considers the importance of the words in the documents, attempting to quantify how relevant that word is to the subject of the document. In other words, TF-IDF analyzes relevance instead of frequency and the word counts in a feature vector are replaced by a TF-IDF score that is calculated with regard to the whole dataset.
A TF-IDF approach operates by first calculating the term frequency, the number of times that the unique terms appear within a specific document. However, TF-IDF also takes care to limit the influence that extremely common words like “the”, “or”, and “and”, as these “stopwords” are very common yet convey very little information about the content of the document. These words need to be discounted, which is what the “inverse-document frequency” part of TF-IDF refers to. This is done is because the more documents that a specific words shows up in, the less useful that word is in distinguishing it from the other documents in the list of all documents. The formula that TF-IDF uses to calculate the importance of a word is designed to preserve the words that are the most frequent and the most semantically rich.
The feature vectors created by the TF-IDF approach contain normalized values that sum to one, assigning each word a weighted value as calculated by the TF-IDF formula.
Word Embeddings
Word embeddings are methods of representing text that ensure that words with similar meanings have similar numerical representations.
Word embeddings operate by “vectorizing” words, meaning that they represent words as real-valued-vectors in a vector space. The vectors exist in a grid or matrix, and they have a direction and length (or magnitude). When representing words as vectors, the words are converted into vectors comprised of real values. Every word is mapped to one vector, and words that are similar in meaning have similar direction and magnitude. This type of encoding makes it possible for a machine learning algorithm to learn complicated relationships between words.
The embeddings that represent different words are created with regard to how the words in question are used. Because words that are used in similar ways will have similar vectors, the process of creating word embeddings automatically translates some of the meaning the words have. A bag of words approach, by contrast, creates brittle representations where different words will have dissimilar representations even if they are used in highly similar contexts.
As a result, word embeddings are better at capturing the context of words within a sentence.
There are different algorithms and approaches used to create word embeddings. Some of the most common and reliable word embedding methods include: embedding layers, word2vec, and GloVe.
Embedding Layers
One potential way to use word embeddings alongside a machine learning/deep learning system is to use an embedding layer. Embedding layers are deep learning layers that convert words into embeddings which is then fed into the rest of the deep learning system. The word embeddings are learned as the network trains for a specific text-based task.
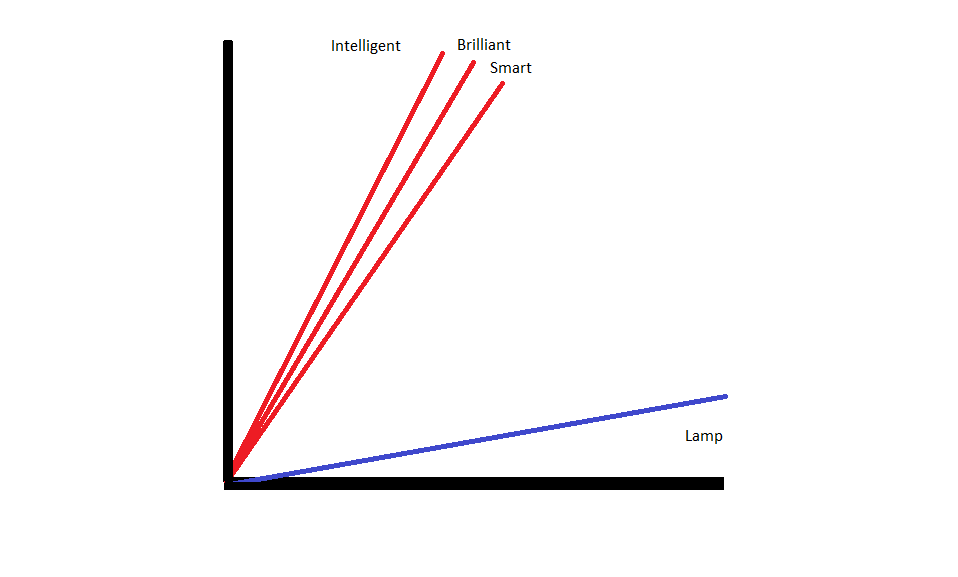
In a word embedding approach, similar words will have similar representations and be closer to each other than to dissimilar words.
In order to use embedding layers, the text needs to be preprocessed first. The text in the document has to be one-hot encoded, and the vector size needs to be specified in advance. The one-hot text is then converted to word vectors and the vectors are passed into the machine learning model.
Word2Vec
Word2Vec is another common method of embedding words. Word2Vec uses statistical methods to convert words to embeddings and it is optimized for use with neural network based models. Word2Vec was developed by Google researchers and it is one of the most commonly used embedding methods, as it reliably yields useful, rich embeddings. Word2Vec representations are useful for identifying semantic and syntactic commonalities in language. This means that Word2Vec representations capture relationships between similar concepts, being able to distinguish that the commonality between “King” and “Queen” is royalty and that “King” implies “man-ness” while Queen implies “Woman-ness”.
GloVe
GloVE, or Global Vector for Word Representation, builds upon the embedding algorithms used by Word2Vec. GloVe embedding methods combine aspects of both Word2Vec and matrix factorization techniques like Latent Semantic Analysis. The advantage of Word2Vec is that it can capture context, but as a tradeoff it poorly captures global text statistics. Conversely, traditional vector representations are good at determining global text statistics but they aren’t useful for determining the context of words and phrases. GloVE draws from the best of both approaches, creating word-context based on global text statistics.
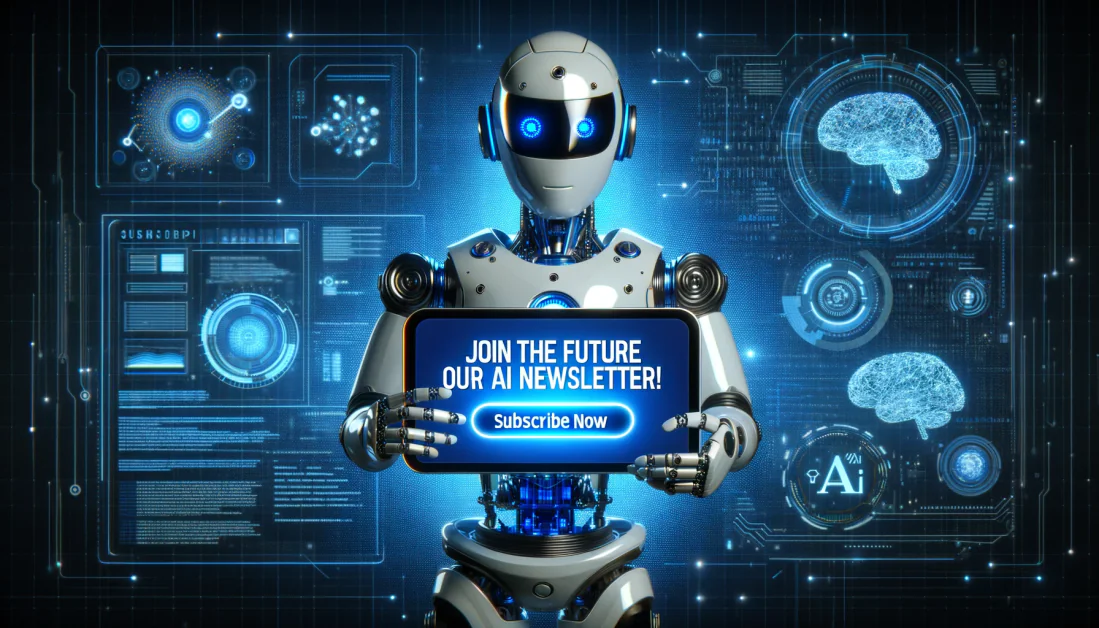
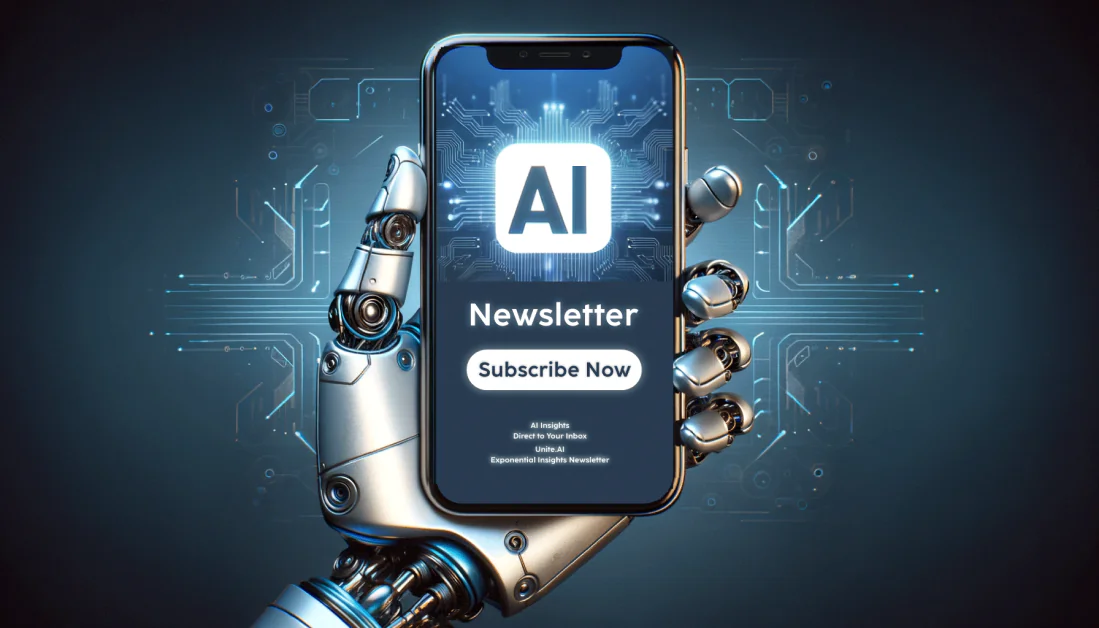
Blogger and programmer with specialties in Machine Learning and Deep Learning topics. Daniel hopes to help others use the power of AI for social good.
You may like
The Rise of AI Software Engineers: SWE-Agent, Devin AI and the Future of Coding
InstructIR: High-Quality Image Restoration Following Human Instructions
NLP Rise with Transformer Models | A Comprehensive Analysis of T5, BERT, and GPT
Analogical & Step-Back Prompting: A Dive into Recent Advancements by Google DeepMind
LlamaIndex: Augment your LLM Applications with Custom Data Easily
The Role of Vector Databases in Modern Generative AI Applications