Thought Leaders
New Frontiers in Generative AI — Far From the Cloud
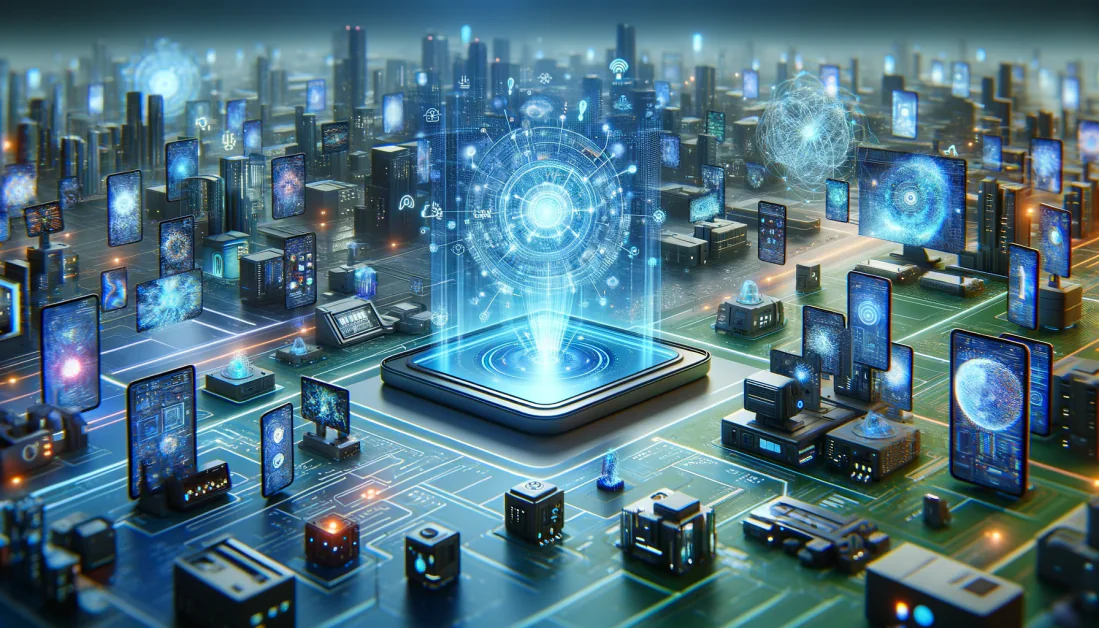
In the beginning, there was the internet, which changed our lives forever — the way we communicate, shop, conduct business. And then for reasons of latency, privacy, and cost-efficiency, the internet moved to the network edge, giving rise to the “internet of things.”
Now there’s artificial intelligence, which makes everything we do on the internet easier, more personalized, more intelligent. To use it, however, large servers are needed, and high compute capacity, so it’s confined to the cloud. But the same motivations — latency, privacy, cost efficiency — have driven companies like Hailo to develop technologies that enable AI on the edge.
Undoubtedly, the next big thing is generative AI. Generative AI presents enormous potential across industries. It can be used to streamline work and increase the efficiency of various creators — lawyers, content writers, graphic designers, musicians, and more. It can help discover new therapeutic drugs or aid in medical procedures. Generative AI can improve industrial automation, develop new software code, and enhance transportation security through the automated synthesis of video, audio, imagery, and more.
However, generative AI as it exists today is limited by the technology that enables it. That’s because generative AI happens in the cloud — large data centers of costly, energy-consuming computer processors far removed from actual users. When someone issues a prompt to a generative AI tool like ChatGPT or some new AI-based videoconferencing solution, the request is transmitted via the internet to the cloud, where it’s processed by servers before the results are returned over the network.
As companies develop new applications for generative AI and deploy them on different types of devices — video cameras and security systems, industrial and personal robots, laptops and even cars — the cloud is a bottleneck in terms of bandwidth, cost, and connectivity.
And for applications like driver assist, personal computer software, videoconferencing and security, constantly moving data over a network can be a privacy risk.
The solution is to enable these devices to process generative AI at the edge. In fact, edge-based generative AI stands to benefit many emerging applications.
Generative AI on the Rise
Consider that in June, Mercedes-Benz said it would introduce ChatGPT to its cars. In a ChatGPT-enhanced Mercedes, for example, a driver could ask the car — hands free — for a dinner recipe based on ingredients they already have at home. That is, if the car is connected to the internet. In a parking garage or remote location, all bets are off.
In the last couple of years, videoconferencing has become second nature to most of us. Already, software companies are integrating forms of AI into videoconferencing solutions. Maybe it’s to optimize audio and video quality on the fly, or to “place” people in the same virtual space. Now, generative AI-powered videoconferences can automatically create meeting minutes or pull in relevant information from company sources in real-time as different topics are discussed.
However, if a smart car, videoconferencing system, or any other edge device can’t reach back to the cloud, then the generative AI experience can’t happen. But what if they didn’t have to? It sounds like a daunting task considering the enormous processing of cloud AI, but it is now becoming possible.
Generative AI at the Edge
Already, there are generative AI tools, for example, that can automatically create rich, engaging PowerPoint presentations. But the user needs the system to work from anywhere, even without an internet connection.
Similarly, we’re already seeing a new class of generative AI-based “copilot” assistants that will fundamentally change how we interact with our computing devices by automating many routine tasks, like creating reports or visualizing data. Imagine flipping open a laptop, the laptop recognizing you through its camera, then automatically generating a course of action for the day/week/month based on your most used tools, like Outlook, Teams, Slack, Trello, etc. But to maintain data privacy and a good user experience, you must have the option of running generative AI locally.
In addition to meeting the challenges of unreliable connections and data privacy, edge AI can help reduce bandwidth demands and enhance application performance. For instance, if a generative AI application is creating data-rich content, like a virtual conference space, via the cloud, the process could lag depending on available (and costly) bandwidth. And certain types of generative AI applications, like security, robotics, or healthcare, require high-performance, low-latency responses that cloud connections can’t handle.
In video security, the ability to re-identify people as they move among many cameras — some placed where networks can’t reach — requires data models and AI processing in the actual cameras. In this case, generative AI can be applied to automated descriptions of what the cameras see through simple queries like, “Find the 8-year-old child with the red T-shirt and baseball cap.”
That’s generative AI at the edge.
Developments in Edge AI
Through the adoption of a new class of AI processors and the development of leaner, more efficient, though no-less-powerful generative AI data models, edge devices can be designed to operate intelligently where cloud connectivity is impossible or undesirable.
Of course, cloud processing will remain a critical component of generative AI. For example, training AI models will remain in the cloud. But the act of applying user inputs to those models, called inferencing, can — and in many cases should — happen at the edge.
The industry is already developing leaner, smaller, more efficient AI models that can be loaded onto edge devices. Companies like Hailo manufacture AI processors purpose-designed to perform neural network processing. Such neural-network processors not only handle AI models incredibly rapidly, but they also do so with less power, making them energy efficient and apt to a variety of edge devices, from smartphones to cameras.
Processing generative AI at the edge can also effectively load-balance growing workloads, allow applications to scale more stably, relieve cloud data centers of costly processing, and help them reduce their carbon footprint.
Generative AI is poised to change computing again. In the future, the LLM on your laptop may auto-update the same way your OS does today — and function in much the same way. But to get there, we’ll need to enable generative AI processing at the network’s edge. The result promises to be greater performance, energy efficiency, and privacy and security. All of which leads to AI applications that change the world as much as generative AI itself.
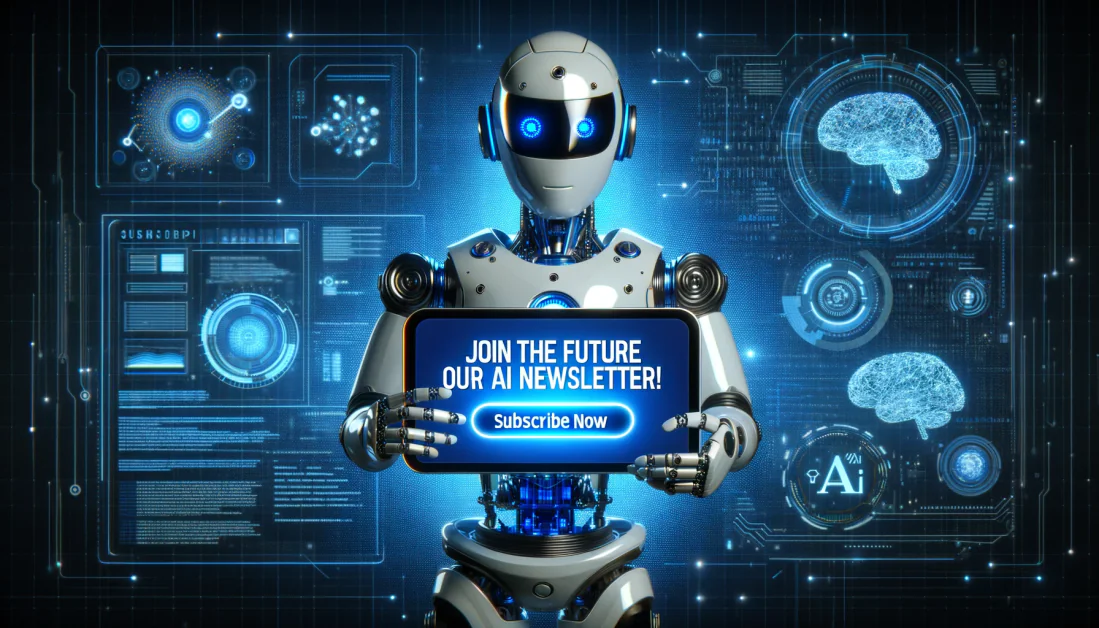
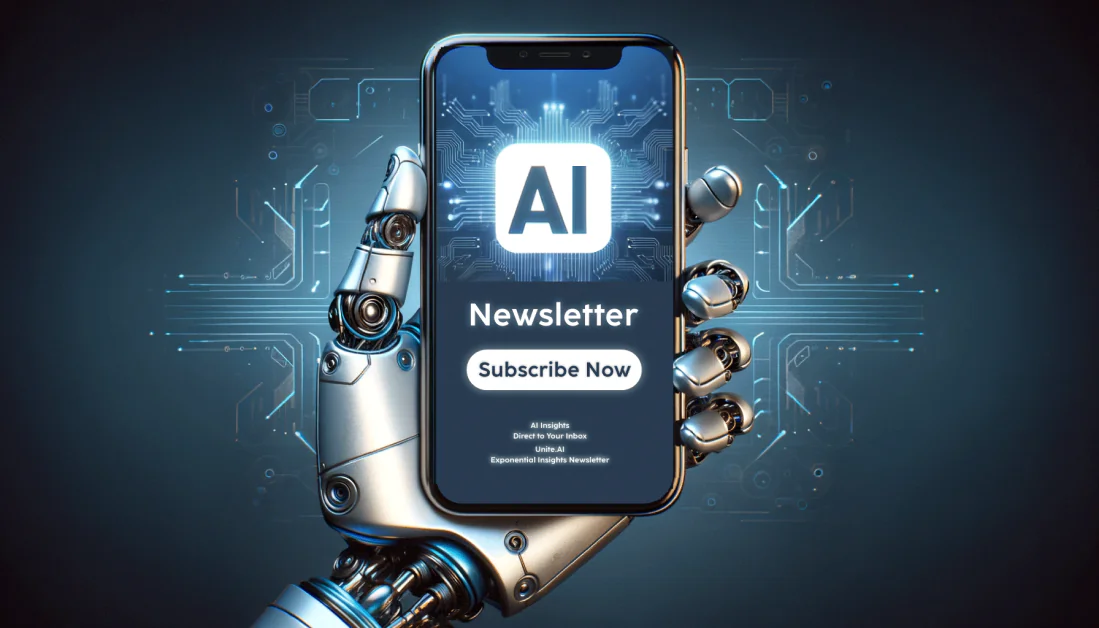