Artificial Intelligence
RigNeRF: A New Deepfakes Method That Uses Neural Radiance Fields
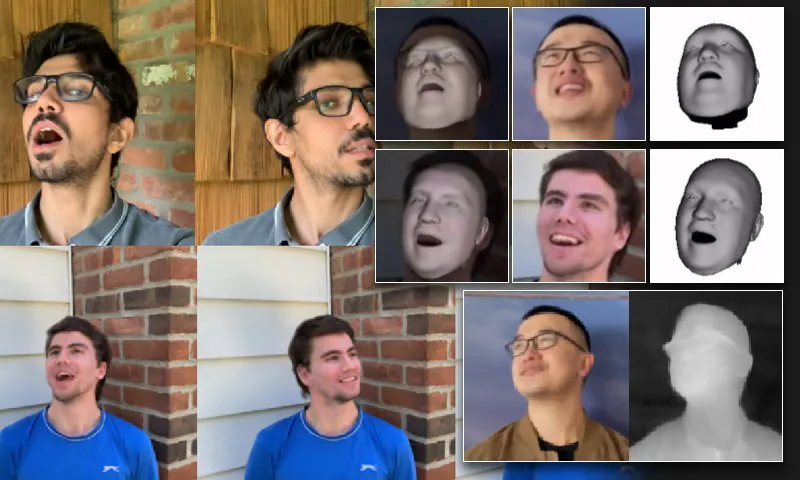
New research developed at Adobe is offering the first viable and effective deepfakes method based on Neural Radiance Fields (NeRF) – perhaps the first real innovation in architecture or approach in the five years since the emergence of deepfakes in 2017.
The method, titled RigNeRF, uses 3D morphable face models (3DMMs) as an interstitial layer of instrumentality between the desired input (i.e. the identity to be imposed into the NeRF render) and the neural space, a method that has been widely adopted in recent years by Generative Adversarial Network (GAN) face synthesis approaches, none of which have yet produced functional and useful face-replacement frameworks for video.
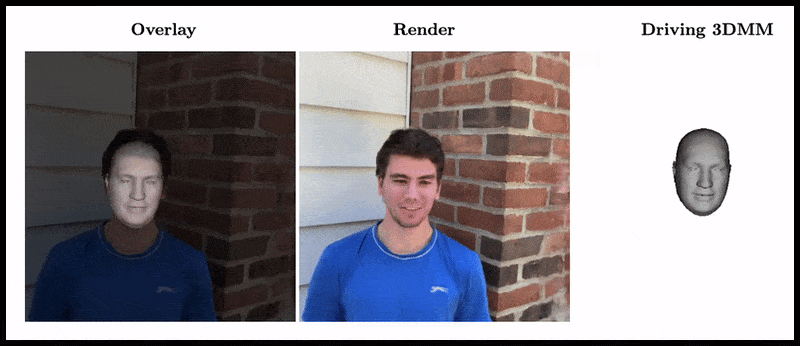
Unlike traditional deepfake videos, absolutely none of the moving content pictured here is ‘real', but rather is an explorable neural space that was trained on brief footage. On the right we see the 3D morphable face model (3DMM) acting as an interface between the desired manipulations (‘smile', ‘look left', ‘look up', etc.) and the usually-intractable parameters of a Neural Radiance Field visualization. For a high-resolution version of this clip, along with other examples, see the project page, or the embedded videos at the end of this article. Source: https://shahrukhathar.github.io/2022/06/06/RigNeRF.html
3DMMs are effectively CGI models of faces, the parameters of which can be adapted to more abstract image synthesis systems, such as NeRF and GAN, which are otherwise difficult to control.
What you're seeing in the image above (middle image, man in blue shirt), as well as the image directly below (left image, man in blue shirt), is not a ‘real' video into which a small patch of ‘fake' face has been superimposed, but an entirely synthesized scene that exists solely as a volumetric neural rendering – including the body and background:
In the example directly above, the real-life video on the right (woman in red dress) is used to ‘puppet' the captured identity (man in blue shirt) on the left via RigNeRF, which (the authors claim) is the first NeRF-based system to achieve separation of pose and expression while being able to perform novel view syntheses.
The male figure on the left in the image above was ‘captured' from a 70-second smartphone video, and the input data (including the entire scene information) subsequently trained across 4 V100 GPUs to obtain the scene.
Since 3DMM-style parametric rigs are also available as entire-body parametric CGI proxies (rather than just face rigs), RigNeRF potentially opens up the possibility of full-body deepfakes where real human movement, texture and expression is passed to the CGI-based parametric layer, which would then translate action and expression into rendered NeRF environments and videos.
As for RigNeRF – does it qualify as a deepfake method in the current sense that the headlines understand the term? Or is it just another semi-hobbled also-ran to DeepFaceLab and other labor-intensive, 2017-era autoencoder deepfake systems?
The new paper's researchers are unambiguous on this point:
‘Being a method that is capable of reanimating faces, RigNeRF is prone to misuse by bad actors to generate deep-fakes.'
The new paper is titled RigNeRF: Fully Controllable Neural 3D Portraits, and comes from ShahRukh Atha of Stonybrook University, an intern at Adobe during RigNeRF's development, and four other authors from Adobe Research.
Beyond Autoencoder-Based Deepfakes
The majority of viral deepfakes that have captured headlines over the last few years are produced by autoencoder-based systems, derived from the code that was published at the promptly-banned r/deepfakes subreddit in 2017 – though not before being copied over to GitHub, where it has currently been forked over a thousand times, not least into the popular (if controversial) DeepFaceLab distribution, and also the FaceSwap project.
Besides GAN and NeRF, autoencoder frameworks have also experimented with 3DMMs as ‘guidelines' for improved facial synthesis frameworks. An example of this is the HifiFace project from July of 2021. However, no usable or popular initiatives seem to have developed from this approach to date.
Data for RigNeRF scenes are obtained by capturing short smartphone videos. For the project, RigNeRF's researchers used an iPhone XR or an iPhone 12 for all experiments. For the first half of the capture, the subject is asked to perform a wide range of facial expressions and speech while keeping their head still as the camera is moved around them.
For the second half of the capture, the camera maintains a fixed position while the subject must move their head around whilst evincing a wide range of expressions. The resultant 40-70 seconds of footage (around 1200-2100 frames) represent the entire dataset that will be used to train the model.
Cutting Down on Data-Gathering
By contrast, autoencoder systems such as DeepFaceLab require the relatively laborious gathering and curation of thousands of diverse photos, often taken from YouTube videos and other social media channels, as well as from movies (in the case of celebrity deepfakes).
The resultant trained autoencoder models are often intended to be used in a variety of situations. However, the most fastidious ‘celebrity' deepfakers may train entire models from scratch for a single video, despite the fact that training can take a week or more.
Despite the warning note from the new paper's researchers, the ‘patchwork' and broadly-assembled datasets that power AI porn as well as popular YouTube/TikTok ‘deepfake recastings' seem unlikely to produce acceptable and consistent results in a deepfake system such as RigNeRF, which has a scene-specific methodology. Given the restrictions on data capture outlined in the new work, this could prove, to some extent, an additional safeguard against casual misappropriation of identity by malicious deepfakers.
Adapting NeRF to Deepfake Video
NeRF is a photogrammetry-based method in which a small number of source pictures taken from various viewpoints are assembled into an explorable 3D neural space. This approach came to prominence earlier this year when NVIDIA unveiled its Instant NeRF system, capable of cutting the exorbitant training times for NeRF down to minutes, or even seconds:
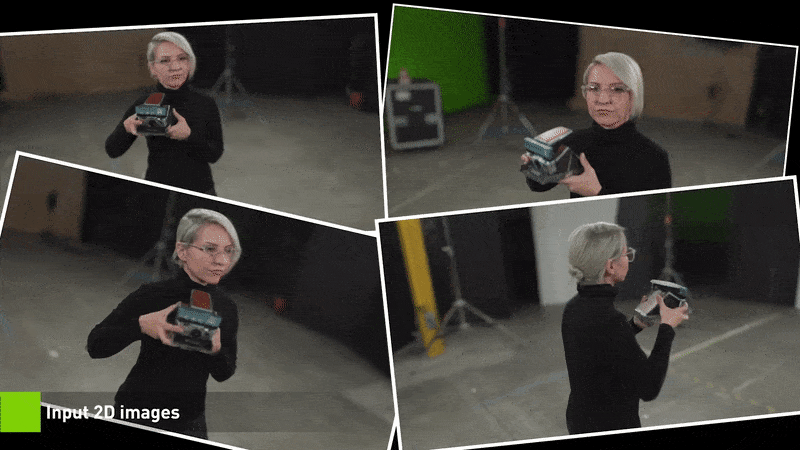
Instant NeRF. Source: https://www.youtube.com/watch?v=DJ2hcC1orc4
The resulting Neural Radiance Field scene is essentially a static environment which can be explored, but which is difficult to edit. The researchers note that two prior NeRF-based initiatives – HyperNeRF + E/P and NerFACE – have taken a stab at facial video synthesis, and (apparently for the sake of completeness and diligence) have set RigNeRF against these two frameworks in a testing round:
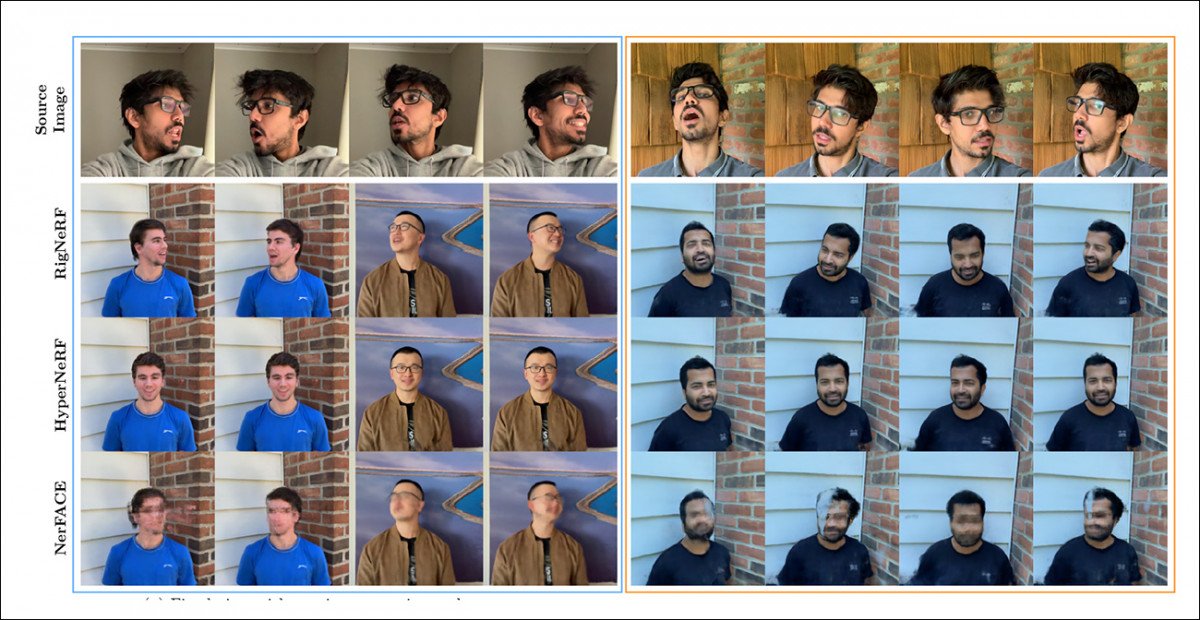
A qualitative comparison between RigNeRF, HyperNeRF, and NerFACE. See the linked source videos and PDF for higher-quality versions. Static image source: https://arxiv.org/pdf/2012.03065.pdf
However, in this case the results, which favor RigNeRF, are fairly anomalous, for two reasons: firstly, the authors observe that ‘there is no existing work for an apple-to-apple comparison'; secondly, this has necessitated the limiting of RigNeRF's capabilities to at least partially match the more restricted functionality of the prior systems.
Since the results are not an incremental improvement on prior work, but rather represent a ‘breakthrough' in NeRF editability and utility, we'll leave the testing round aside, and instead see what RigNeRF is doing differently from its predecessors.
Combined Strengths
The primary limitation of NerFACE, which can create pose/expression control in a NeRF environment, is that it assumes that source footage will be captured with a static camera. This effectively means that it cannot produce novel views that extend beyond its capture limitations. This produces a system that can create ‘moving portraits', but which is unsuitable for deepfake-style video.
HyperNeRF, on the other hand, while able to generate novel and hyper-real views, has no instrumentality that allows it to change head poses or facial expressions, which again does not result in any kind of competitor for autoencoder-based deepfakes.
RigNeRF is able to combine these two isolated functionalities by creating a ‘canonical space', a default baseline from which deviations and deformations can be enacted via input from the 3DMM module.
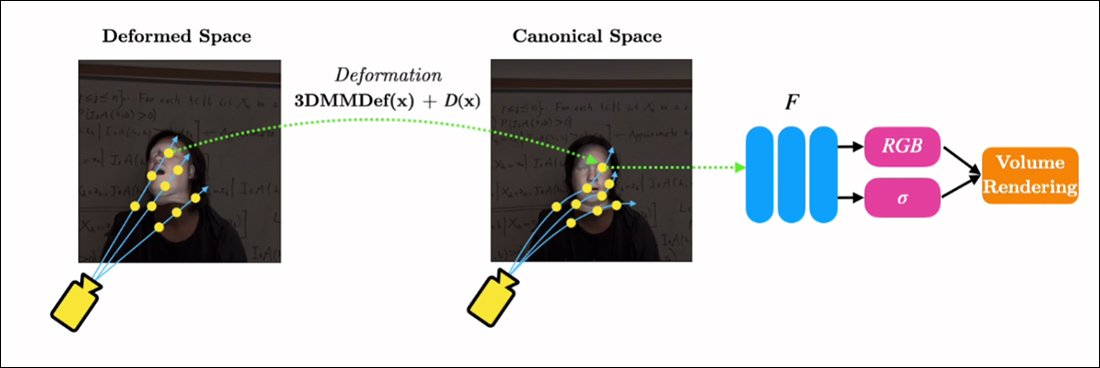
Creating a ‘canonical space' (no pose, no expression), on which the deformations (i.e. poses and expressions) produced via the 3DMM can act.
Since the 3DMM system will not be exactly matched to the captured subject, it's important to compensate for this in the process. RigNeRF accomplishes this with a deformation field prior that's calculated from a Multilayer Perceptron (MLP) derived from the source footage.
The camera parameters necessary to calculate deformations are obtained via COLMAP, while the expression and shape parameters for each frame are obtained from DECA.
The positioning is further optimized through landmark fitting and COLMAP's camera parameters, and, due to computing resource restrictions, the video output is downsampled to 256×256 resolution for training (a hardware-constrained shrinking process that also plagues the autoencoder deepfaking scene).
After this, the deformation network is trained on the four V100s – formidable hardware that's not likely to be within the reach of casual enthusiasts (however, where machine learning training is concerned, it's often possible to trade heft for time, and simply accept that model training will be a matter of days or even weeks).
In conclusion, the researchers state:
‘In contrast to other methods, RigNeRF, thanks to the use of a 3DMM-guided deformation module, is able to model head-pose, facial expressions and the full 3D portrait scene with high fidelity, thus giving better reconstructions with sharp details.'
See the embedded videos below for further details and results footage.
First published 15th June 2022.
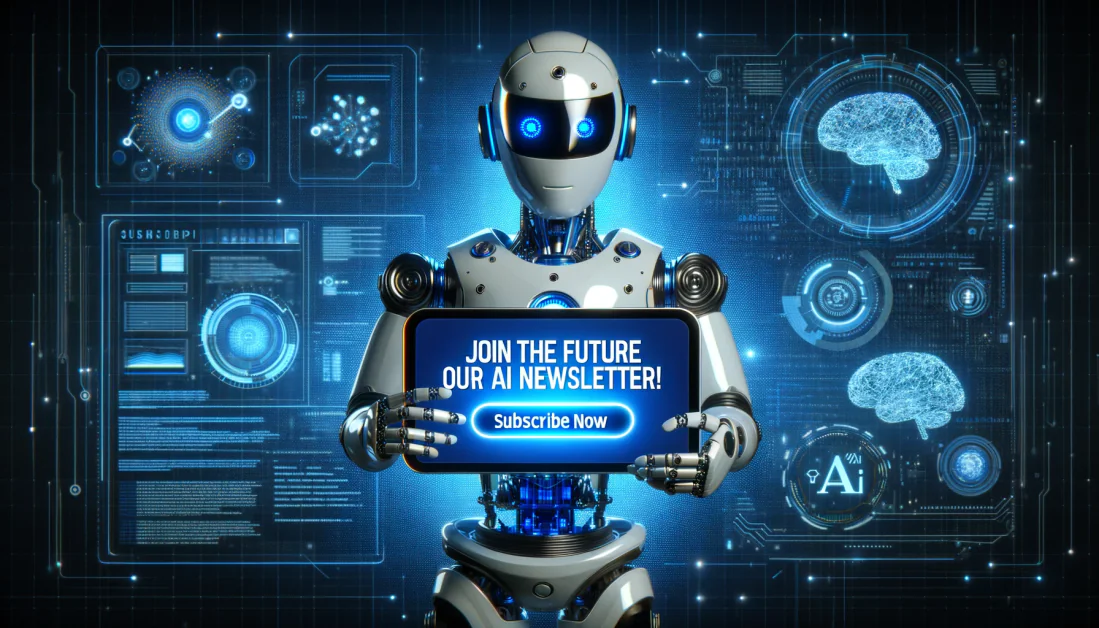
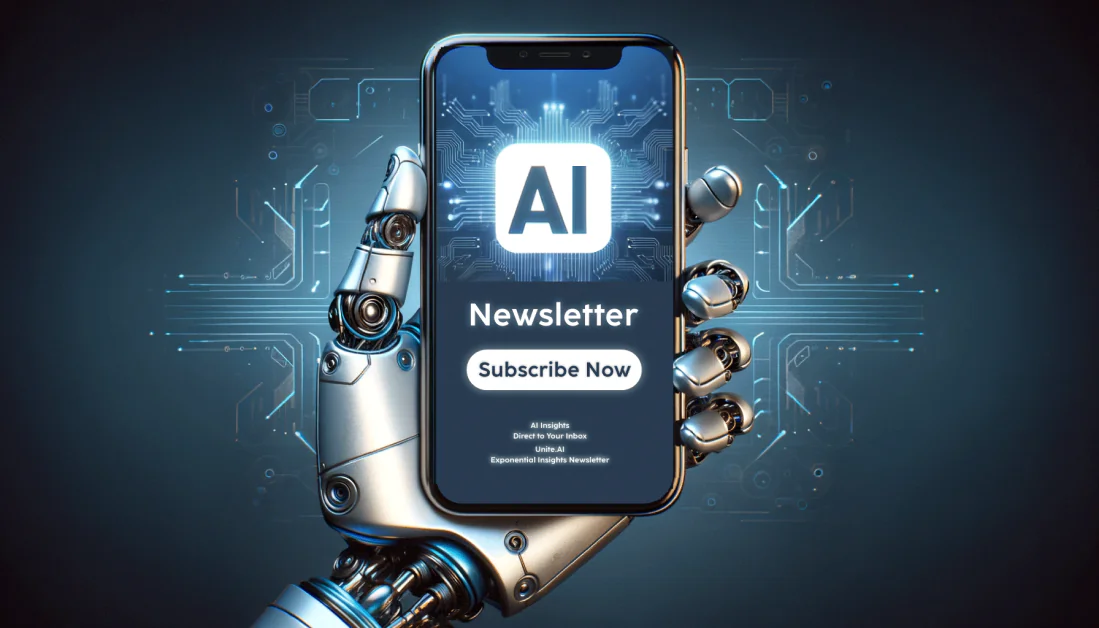