Artificial Intelligence
New Deepfake Method Solves the ‘Face Host’ Problem
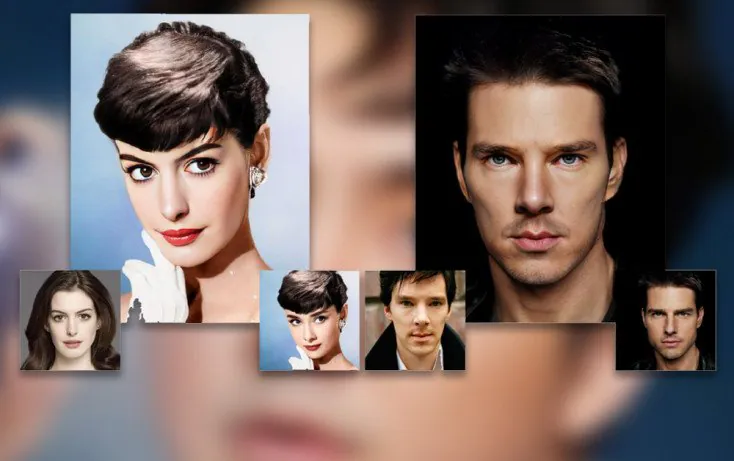
Despite several years of media hyperbole about the potential for deepfake images to undermine our long-held faith in the authenticity of video footage, all currently popular methods rely on finding ‘face hosts' that are broadly similar in shape to the target face.
Where the original footage features a wide face, but the target subject has a narrow face, results have always been problematic, because such a transfer involves cutting away part of the original face and reconstructing the now-exposed background. Current packages such as DeepFaceLab and FaceSwap are able to produce limited results when the configuration is reversed (narrow>wide), but have no facility to convincingly tackle this scenario.
Now, a collaboration between Tencent and China's Xiamen University has developed a new approach, titled HifiFace, designed to redress this shortfall.
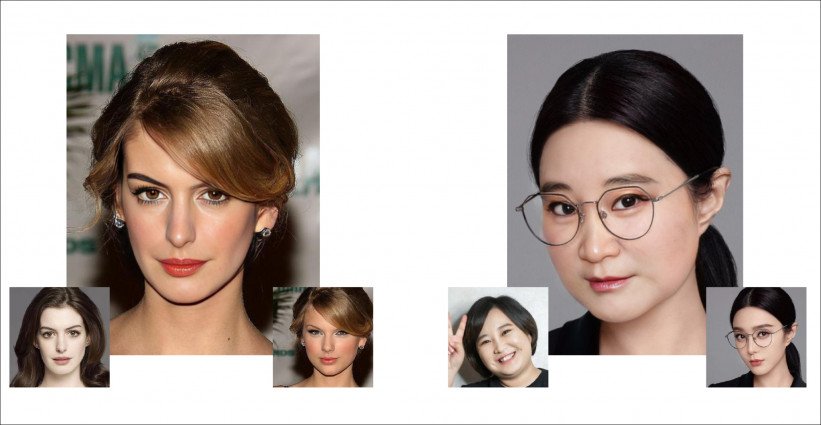
Two HifiFace deepfakes, the first of Anne Hathaway, where a good likeness is obtained in spite of incompatible host face shape. HifiFace also performs well on targets with glasses, traditionally a stumbling block in deepfakes. Source: https://arxiv.org/pdf/2106.09965.pdf
Remodeling a Deepfake Face
Previous approaches, such as 2019's Subject Agnostic Face Swapping and Reenactment (FSGAN), have depended on 3DMM fitting (3D Morphable Models) or other methodologies based around facial landmark recognition or transformation, where the facial lineaments of the face to be ‘overwritten' pretty much dictate the bounds of the swap:
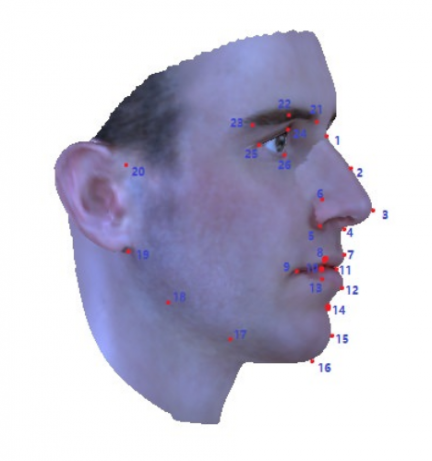
3DMM facial landmark detection. Source: https://github.com/Yinghao-Li/3DMM-fitting
Though competing methods have drawn on features derived from face recognition networks, these are primarily aimed at reconstituting texture rather than structure, and similarly produce a ‘mask-like' effect in cases where the host face is not entirely compatible (i.e. the limits and shape of hairline, jawline and cheekbones).
To address these issues, the Chinese researchers, based in the Media Analytics and Computing Lab at the university's Department of Artificial Intelligence, developed an end-to-end network that regresses the coefficients of the target and the source face using a 3D reconstruction model, which is then re-combined as shape information, and concatenated with identity vector information from a face recognition network.
This geometric data is then fed into an encoder-decoder model as structural information, blending with the target face's expression and disposition, which are leveraged as auxiliary sources for accurate transfer.
Semantic Facial Fusion
Additionally, HifiFace includes a Semantic Facial Fusion (SFF) component, which uses a low-level feature in the encoder to preserve spatial and texture information, without sacrificing the identity of the target image. Features from the encoder and decoder are integrated into a learned adaptive mask, and the background information blended into the output by means of the learned face mask.
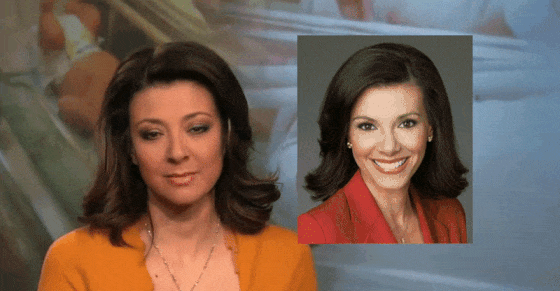
HifiFace in action. Source: https://johann.wang/HifiFace/
In this way, HifiFace departs from the use of original-material face boundaries as a hard limit, by using dilated face semantic segmentation, wherein the model can perform better adaptive fusion on the edge boundaries of the face.
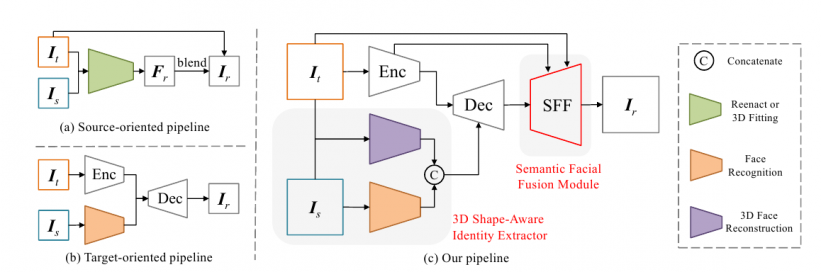
Two prior approaches (top and bottom left), and the new HifiFace architecture, which consists of an encoder, decoder, 3D shape-aware identity extractor, and SFF module.
In a comparison with former methods FSGAN, SimSwap and FaceShifter, HifiFace demonstrates a superior reconstruction of face shape, since it is not approximating ‘ghost' elements where the facial delimitations confound the identity>identity mapping, but definitively reconstructing them.
Testing
The researchers implemented the system using the VGGFace2 and the DeepGlint Asian-Celeb datasets. Faces were aligned via 5 outward landmarks and re-cropped to 256×256 pixels. A portrait enhancement network was also used to generate a 512×512 pixels version, for an additional higher-res model. The model was trained under Adam.
Though FaceShifter preserves identity well, it cannot address issues such expression, color and occlusion as effectively as HifiFace, and has a more complex network structure. FSGAN has issues in transferring the lighting from source to target.
The researchers use FaceForensics++ for quantitative comparisons, sampling ten frames each in a batch of converted videos across the competing methods, and finding that HifiFace achieved a superior ID retrieval score. In testing a range of other factors, such as image quality, the researchers also found that their method outperformed the rival methodologies.
The work represents a further move towards abstracting the source material so that it is only a rough template into which accurate identities can be transferred. Some of the current FOSS packages, including DeepFaceLab, feature nascent functionality for full-head replacement, but, like HifiFace, these do not account for hair, and they are more effective at ‘building out' a face than in chiseling it away to match a desired target source.
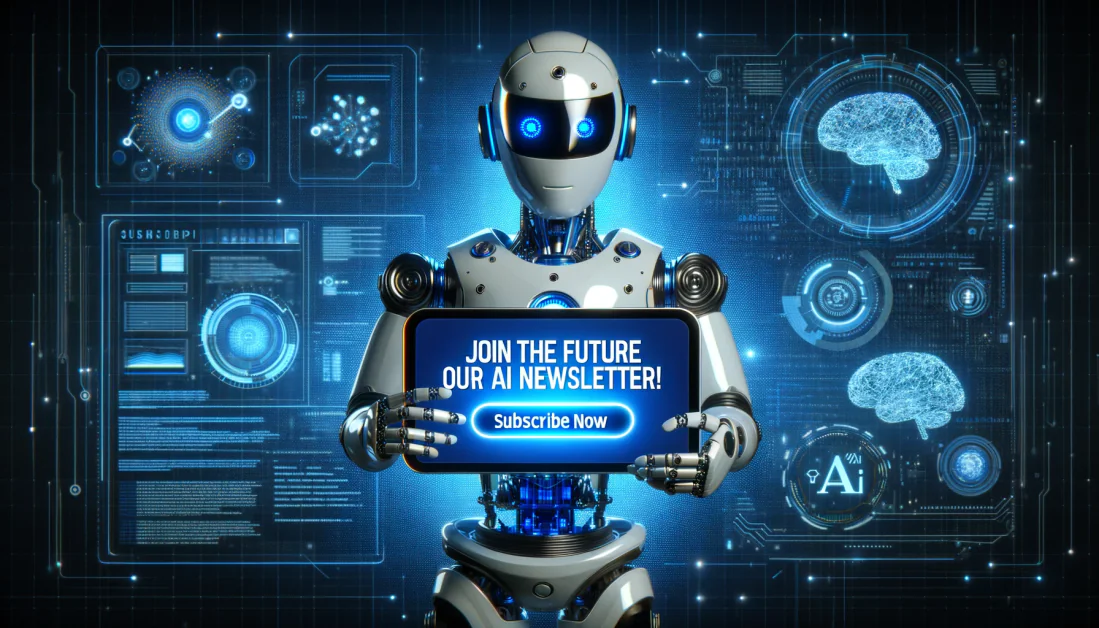
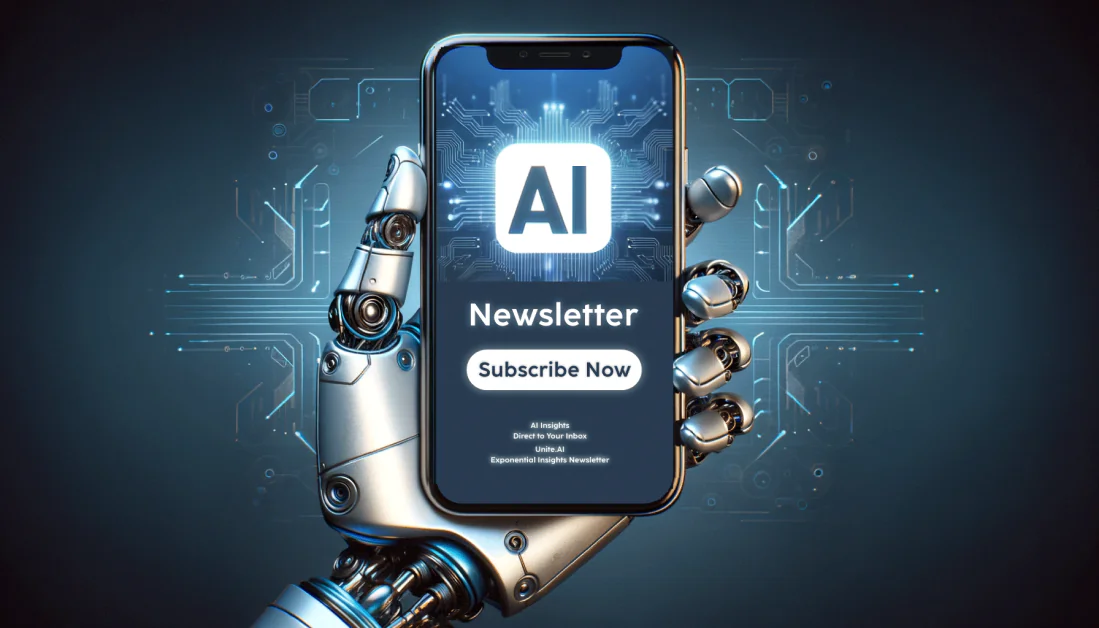