Artificial Intelligence
Researchers Develop AI-Backed Method of Seed Analysis
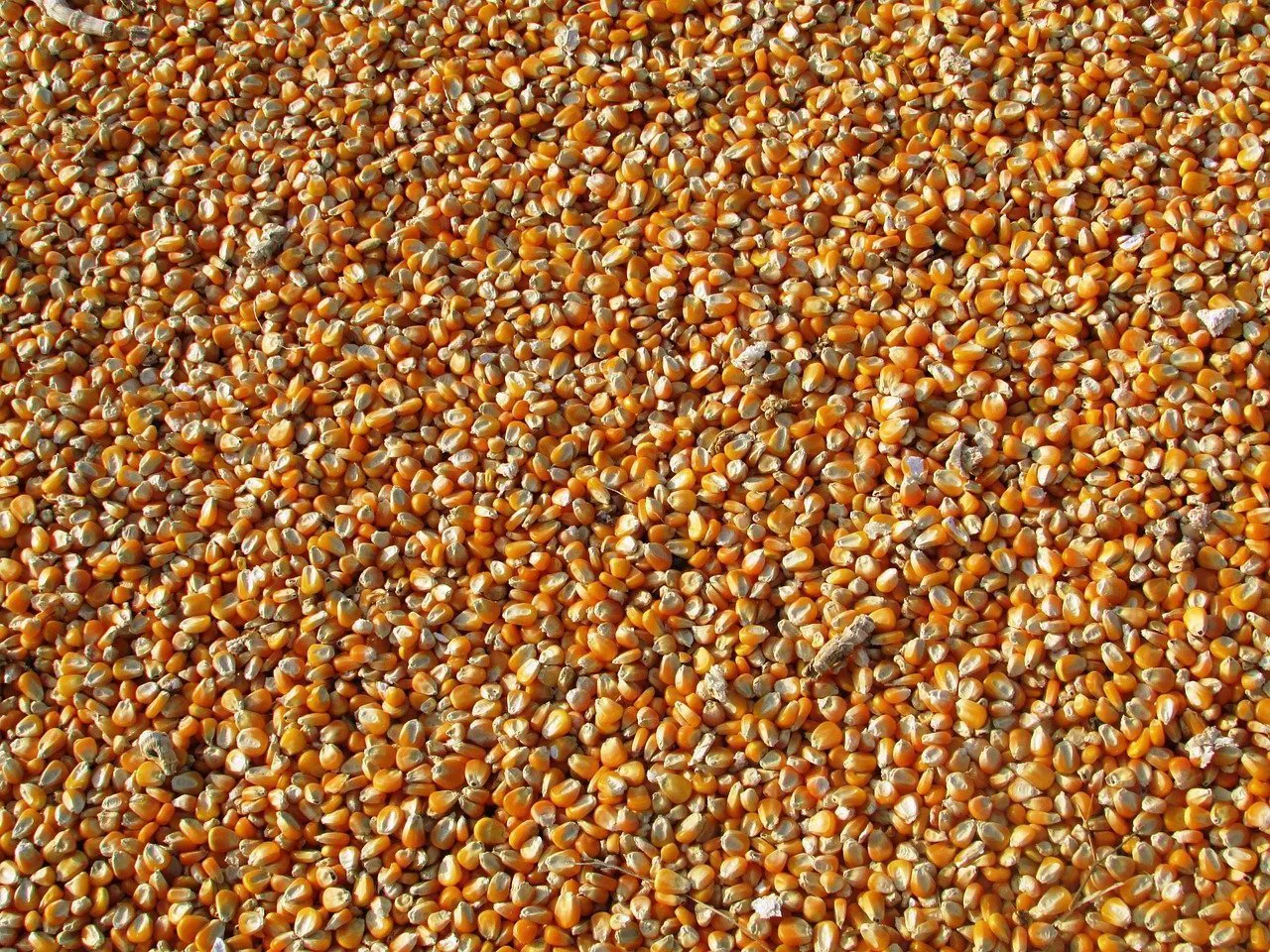
A team of researchers from Brazil’s Center for Nuclear Energy in Agriculture (CENA) and the Luiz de Quieroz College of Agriculture (ESALQ) have created an AI-driven method of seed quality analysis, dramatically reducing the time needed to determine the quality of agricultural seeds.
According to Phys.org, the research team collected images of seeds with the use of light-based imaging technology. The techniques used by the research team included multispectral imaging and chlorophyll fluorescence. The research team selected carrots and tomatoes to serve as their experimental models, choosing different variants to produce in different countries and under different conditions. The seeds they selected were commercial tomato varieties produced in the US and in Brazil, as well as commercial carrot varieties produced in Italy, Chile, and Brazil.
Demand for these crops is rising around the globe, but collecting the seeds for these crops can be difficult. Both carrots and tomatoes have ripening processes that aren’t uniform. Seed production for these crops is also non-synchronous, meaning that seeds lots extracted from these tomatoes and carrots can contain both mature and immature seeds. It isn’t easy to distinguish between mature and immature seeds with the eye, but computer vision systems can make this process easier.
Traditionally, seeds are assessed by either germination and vigor tests. Germination tests involve the sowing and germination of seeds, while vigor tests aim to assess how seeds respond to stress. It can two weeks or more to obtain results from these tests, meaning that machine learning techniques are dramatically faster than these traditional seed analysis techniques.
After collecting the training images, the researchers used a random forest classifier to automate the interpretation of the seed images. This optical imaging system has many advantages over traditional methods of analyzing seeds, one of which is the fact that the optical imaging technology can be used on entire batches of seeds instead of just small samples of those batches. Another advantage the method has over traditional seed assessment techniques is that the computer vision technique is non-invasive, so it doesn’t destroy any products analyzed.
One method of analyzing seed quality the researchers used was chlorophyll fluorescence. Algorithms developed by the research team made use of the presence of chlorophyll within seeds. Chlorophyll supplies the energy seeds need for development, and if the seed still has large volumes of residual chlorophyll within it, this implies the seed isn’t fully mature. This residual chlorophyll can be detected with multispectral imaging, with red light exciting the chlorophyll and special devices capturing its fluorescence and converting it into an electrical signal.
Multispectral imaging involves the use of LEDs to emit light at varying points on the light spectrum. The researchers split the emitted light into 19 different wavelengths and analyzed seed quality based on reflectance for these different wavelengths. They then compared the results they obtained with quality data obtained through typical seed analysis methods. The researchers found that using near-infrared light works best for the assessment of carrot seeds while UV light worked best for the assessment of UV tomato seeds.
Seeds contain proteins, sugars, and lipids that absorb certain wavelengths of light while reflecting the rest of the light. A multispectral camera is used to capture the reflective light, and the resulting image data is used to find the seeds within the entire captured image. The more of a given nutrient that a seed contains the more light wavelengths corresponding to that is absorbed. A series of algorithms is used to identify which wavelength does the best at localizing the seeds. This process can be used to provide information about the chemical composition of the seeds being studies, allowing their quality to be inferred. The research team then employed chemometrics, which are mathematical and statistical models used to classify materials, to create the classes that described seed quality.
Finally, the researchers were able to use machine learning models to assess the accuracy of the chemometrics models they created. In the case of tomato seeds, the quality classification accuracy ranged from 86% to 95%. In the case of carrot seeds, the accuracy ranged from 88% to 97%.
Both the chlorophyll fluorescence technique and the multispectral imaging technique proved reliable and much faster than traditional methods of assessing seed quality. If the method proves reliable, it has the potential to bring higher quality seeds to growers around the world.
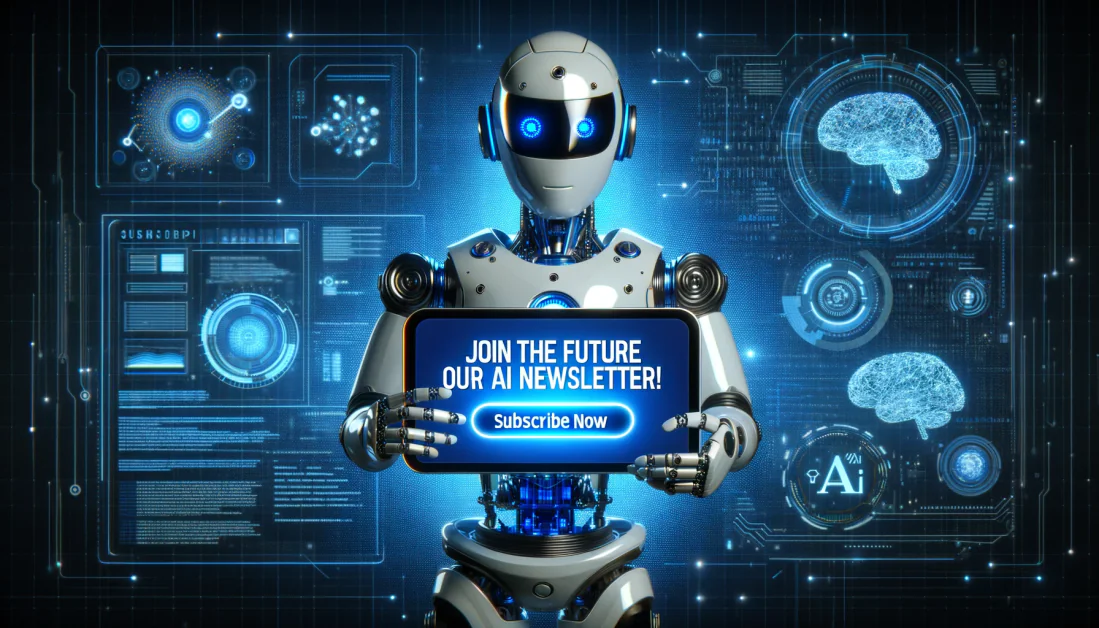
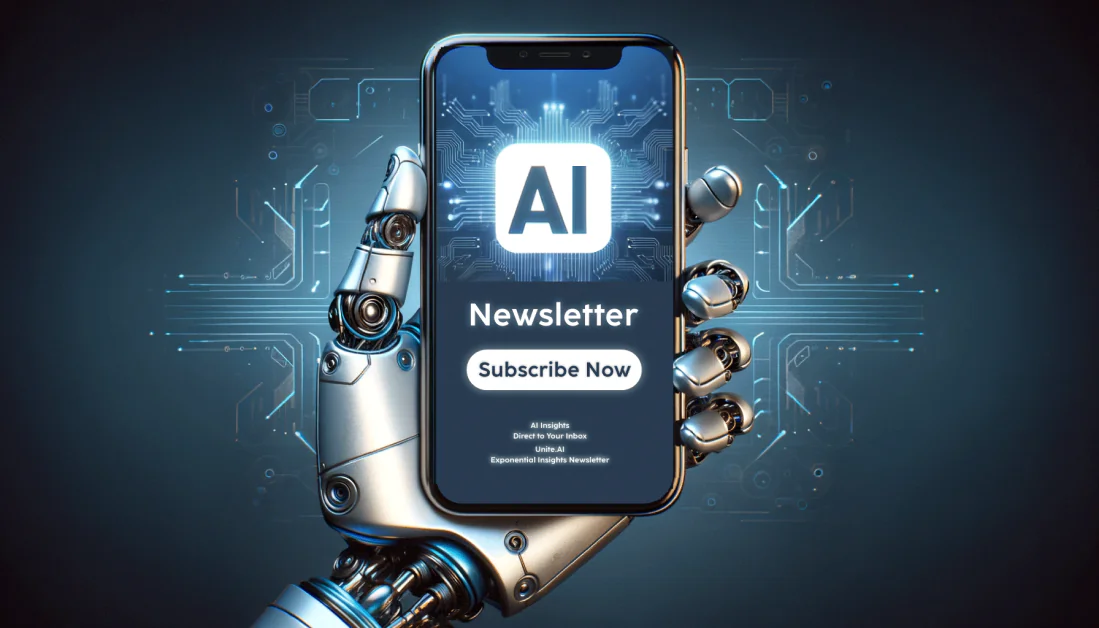