Healthcare
Researchers Aim To Boost Drug Discovery Speed By Calculating Binding Efficiencies With AI
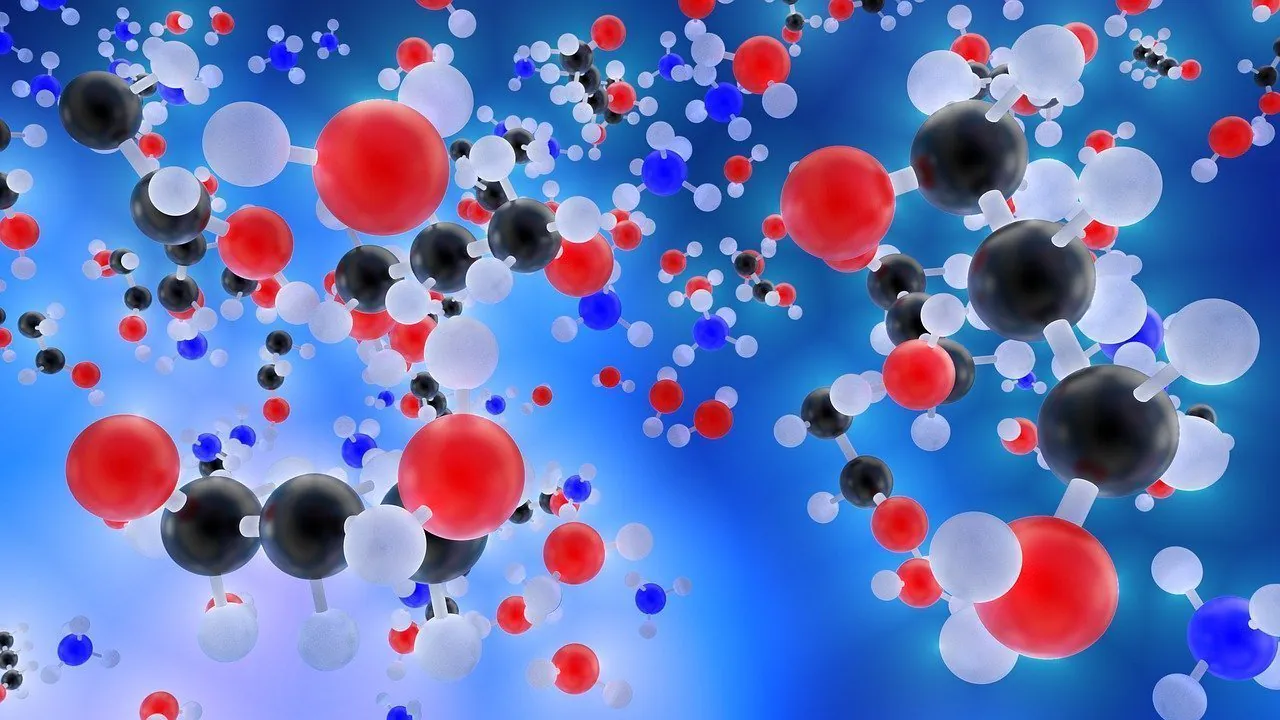
Researchers from MIT have recently developed a new AI-driven technique to speed up the discovery of drugs by increasing the speed of calculations used to assess the molecular binding affinity of a drug.
A drug must be able to stick to proteins in order to carry out the task it was designed for. Assessing the ability of a drug to stick to proteins is a major part of the drug discovery and screening process, and machine learning techniques could reduce the amount of time spent assessing this important drug attribute.
The MIT research team responsible for developing the new drug assessment technique calls it DeepBAR. DeepBAR combines machine learning algorithms with traditional chemistry calculations. DeepBAR calculates the binding potential of a given candidate drug and that drugās target proteins. The new analysis technique delivers estimates of a drugās binding capability substantially faster than traditional methods used to assess binding affinities, and itās hoped that the technique can enhance the speed of drug discovery.
The binding potential of a drug is quantified through a metric called binding free energy, where a smaller number indicates greater binding potential. A low binding free energy score means that a drug has a great ability to compete with other molecules, filling the roles of those molecules and disrupting the normal function of a protein. Thereās a high correlation between the binding free energy of a drug candidate and the effectiveness of that drug. However, measuring binding free energy can be quite difficult.
There are two typical techniques used to measure free binding energies. One method is calculating the exact quantity of binding free energy, while the other is estimating the quantity of binding free energy. Estimates are less computationally expensive than exact measurements, but they obviously come with a tradeoff inaccuracy.
The DeepBAR method uses a fraction of the computational power of exact measuring methods but delivers highly accurate estimates of binding energies. DeepBAR employs the āBennett acceptance ratioā, which is the algorithm typically used to calculate binding free energy. The Bennet acceptance ratio requires the use of two baseline/endpoint states and a variety of intermediates states (which are states of partial binding). The DeepBAR approach attempts to reduce the number of calculations needed to estimate binding energies by utilizing the Bennett acceptance ratio alongside machine-learning frameworks and deep generative models. The machine learning models generate a reference state for each endpoint and these endpoints are accurate enough to the real endpoints that a Bennett acceptance ratio can be deployed.
The deep generative model designed by the MIT research team is based on computer vision techniques. Essentially, DeepBAR treats every molecular structure it analyzes an as image, analyzing the features of the āimageā to learn for them. The research team had to make slight changes to the algorithm to accommodate analysis of the 3D structures, as computer vision algorithms typically operate on 2D images.
In initial tests, DeepBAR was able to calculate binding free energy approximately 50 times faster than traditional techniques. There is still work to be done on the model. It has to be validated against more complex, experimental data than the rather simple data it was initially tested on, which involved fairly simple data. The MIT research team aims to improve DeepBarās ability to calculate binding free energies for large proteins by refining the model using recent advances in computer science.
DeepBAR is far from the first attempt to apply AI to the drug discovery pipeline with the goal of increasing the speed of drug discovery. Many other research projects have also used AI to automate aspect of the drug discovery pipeline and improve their efficiency. However, there could be a natural bottleneck that limits the effectiveness of these strategies.
As Derek Lowe recently argued in a blog on ScienceMag.org, if the goal is to enhance the speed of drug discovery, itās important to āattack the right problemsā. The evaluation of the clinical effectiveness and safety of drugs takes a substantial amount of time and finding ways to use AI to reduce clinical failure rates is difficult. Ultimately, there could be a lower bound on the amount of time AI methods can save in terms of drug discovery, at least until AI can be meaningfully integrated into the clinical evaluation process. Nonetheless, improvements are improvements and the more research like DeepBAR is done, the more time scientists will have to consider ways to use AI in other areas of the drug discovery pipeline.