Artificial Intelligence
Newly Developed Artificial Neural Network Set To Quickly Solve A Physics Problem
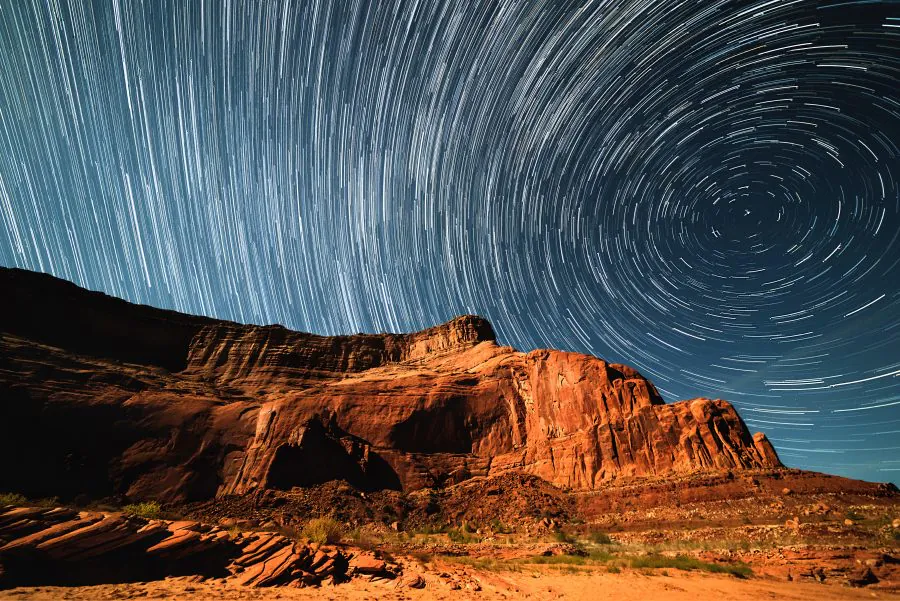
From Sir Isaac Newton on, the so-called three-body problem has confounded mathematicians and physics researchers. As ScienceAlert explains, “the three-body problem involves calculating the movement of three gravitationally interacting bodies – such as the Earth, the Moon, and the Sun, for example – given their initial positions and velocities.”
On the surface, this problem seems a simple one, but in reality, it is an extremely hard one to tackle. One of the results was the introduction of say, marine chronometers to calculate positions at sea, rather than resolving the three-body problem calculating such a position by the Moon and the stars.
With the fast advancement of the study of the Universe, the three-body problem became an important part for researchers when they attempt to figure out “how black hole binaries might interact with single black holes, and from there how some of the most fundamental objects of the Universe interact with each other.”
To make these calculations feasible in a reasonable time, scientists and researchers resorted to the use of ANN, deep artificial neural networks. The new system was developed by a team comprised of researchers from the University of Edinburgh and the University of Cambridge in the UK, the University of Aveiro in Portugal, and Leiden University in the Netherlands.
The ANN this team developed was trained on a database of existing three-body problems as wells as a selection of solutions that scientists have previously figured out.
The results were more than promising. The trained ANN promises to be capable of finding solutions “100 million times faster than existing techniques.”
The resulting research paper, “Newton vs the machine: solving the chaotic three-body problem using deep neural networks” states that, “A trained ANN can replace existing numerical solvers, enabling fast and scalable simulations of many-body systems to shed light on outstanding phenomena such as the formation of black-hole binary systems or the origin of the core-collapse in dense star clusters.”
ScienceAlert notes that “the researchers simplified the process to only include three equal-mass particles in a plane, all starting with zero velocity, and then ran an existing three-body problem solver called Brutus 10,000 times over (9,900 for training and 100 for validation).”
After training, the new ANN came up with impressive results. It was given 5,000 new scenarios to work with and it matched the results Brutus achieved almost completely.
While the study has yet to be peer-reviewed, by scientists with the know and experience in the field and is still more of a proof-of-concept at this stage, it certainly shows that trained neural networks “might be able to work alongside Brutus and similar systems, jumping in when three-body calculations become too complex for our current models to cope with.”
As this team of researchers concludes in their paper, “Eventually, we envision, that network may be trained on richer chaotic problems, such as the 4 and 5-body problem, reducing the computational burden even more.”
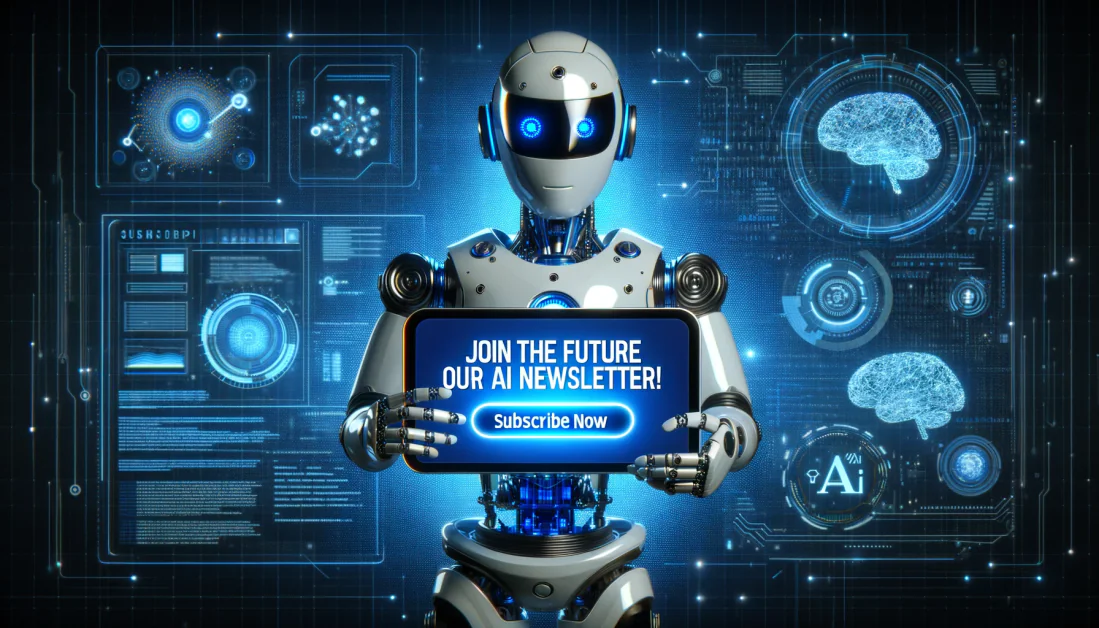
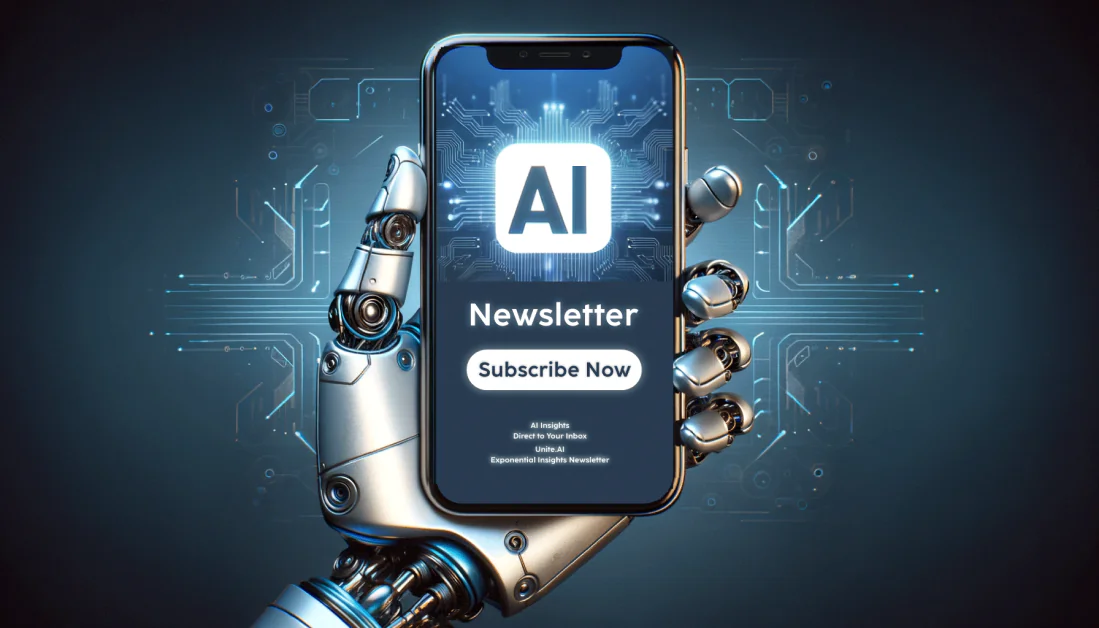