Artificial Intelligence
New Technique Lets AI Intuitively Understand Some Physics
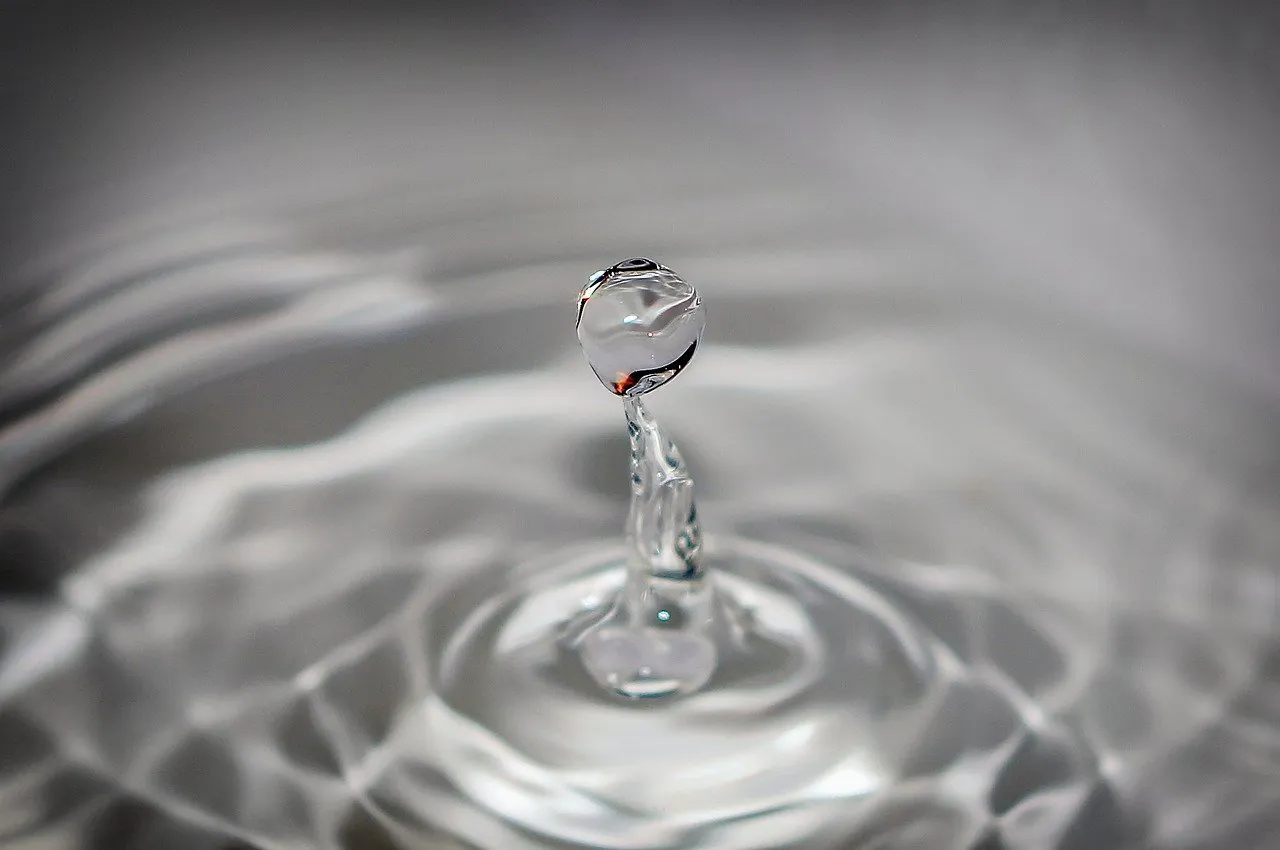
Artificial intelligence has been able to develop an understanding of physics through reinforcement learning for some time now, but a new technique developed by researchers at MIT could help engineers design models that demonstrate an intuitive understanding of physics.
Psychological research has shown that, to some extent, humans have an intuitive understanding of the laws of physics. Infants have expectations of how objects should interact and move, and violations of these expectations will have the infants react with surprise. The research conducted by the MIT team has the potential to not only drive new applications of artificial intelligence, but help psychologists understand how infants perceive and learn about the world.
The model designed by the MIT team is called ADEPT, and it functions by making predictions about how objects should behave in a physical space. The model observes objects and keeps track of a “surprise” metric as it does so. If something unexpected happens, the model responds by increasing its surprise value. Unexpected and seemingly impossible actions such as an object teleporting or vanishing all together will see a dramatic rise in surprise.
The goal of the research team was to get their model to register the same levels of surprise that humans register when they see objects behaving in implausible ways.
ADEPT has two major components to it, a physics engine and an inverse graphics module. The physics engine is responsible for predicting how an object will move, predicting a future representation of an object, from a range of possible states. Meanwhile, the inverse graphics module is responsible for creating the representations of objects that will be fed into the physics engine.
The inverse graphics module tracks several different attributes such as velocity, shape, and orientation of an object, extracting this information from frames of videos. The inverse graphical module only focuses on the most salient details, ignoring details that won’t help the physics engine interpret the object and predict new states. By focusing on only the most important details, the model is better able to generalize to new objects. The physics engine then takes these object descriptions and simulates more complex physical behavior, like fluidity or rigidity, in order to make predictions about how the object should behave.
After this intake process occurs, the model observes the actual next frame in the video, which it uses to recalculate its probability distribution with respect to possible object behaviors. The surprise is inversely proportional to the probability than an event should occur, only registering great surprise when there is a major mismatch between what the model believes should happen next and what actually happens next.
The research team needed some way to compare the surprise of their model to the surprise of people observing the same object behavior. In developmental psychology, researchers often test infants by showing them two different videos. In one video, an object is presented that behaves as you would expect objects to in the real world, not spontaneous play vanishing or teleporting. In the other video and object violates the laws of physics in some fashion. The research team took these same basic concepts and had 60 adults watch 64 different videos of both expected and unexpected physical behavior. The participants were then asked to rate their surprise at various moments in the video on a scale of 1 to 100.
Analysis of the model’s performance demonstrated that it performed quite well on videos where an object was moved behind a wall and disappeared when the wall was removed, typically matching humans surprise levels in these cases. The model also appeared to be surprised by videos where humans didn't demonstrate surprise but arguably should have. As an example, in order for an object to move behind a wall at a given speed and immediately come out on the other side of the wall, it must have either teleported or experienced a dramatic increase in speed.
When compared to the performance of traditional neural networks that are capable of learning from observation but do not explicitly log the representation of an object, researchers found that the ADEPT network was much more accurate at discriminating between surprising and unsurprising scenes and that ADEPT’s performance aligned with human reactions more closely.
The MIT research team is aiming to do more research and gain deeper insight into how infants observe the world around them and learn from these observations, incorporating their findings into new versions of the ADEPT model.
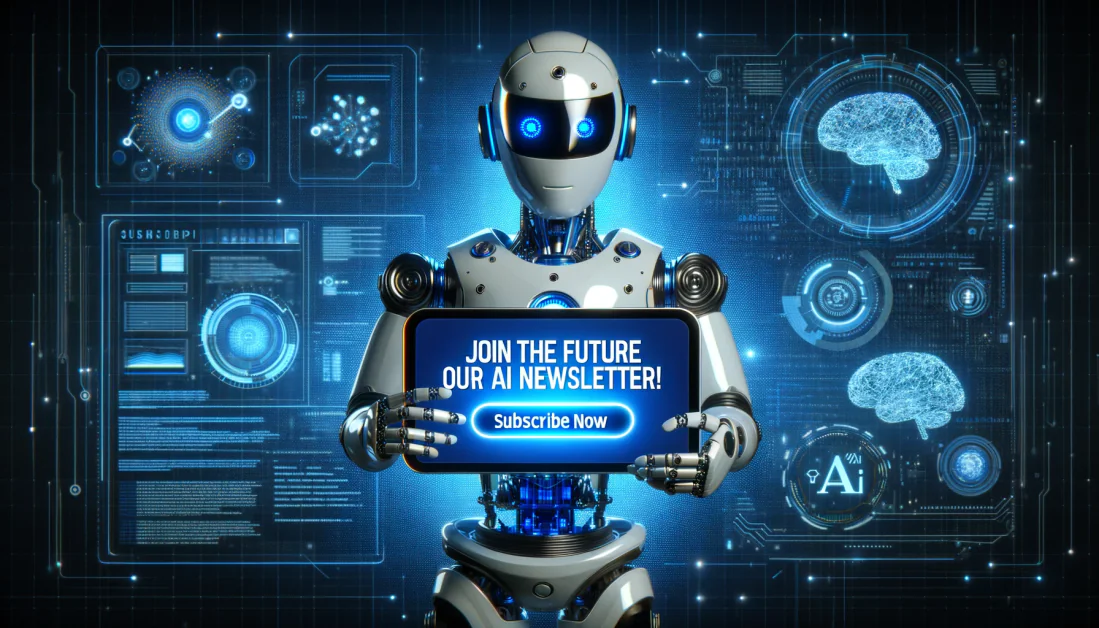
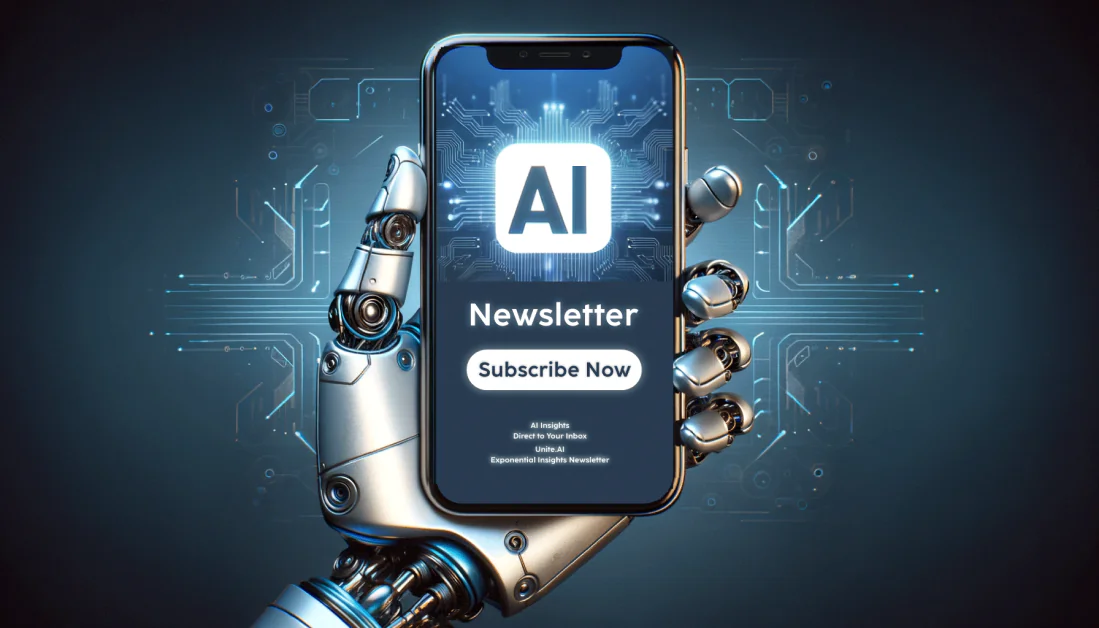