Artificial Intelligence
Artificial Intelligence Enhances Speed of Discoveries For Particle Physics
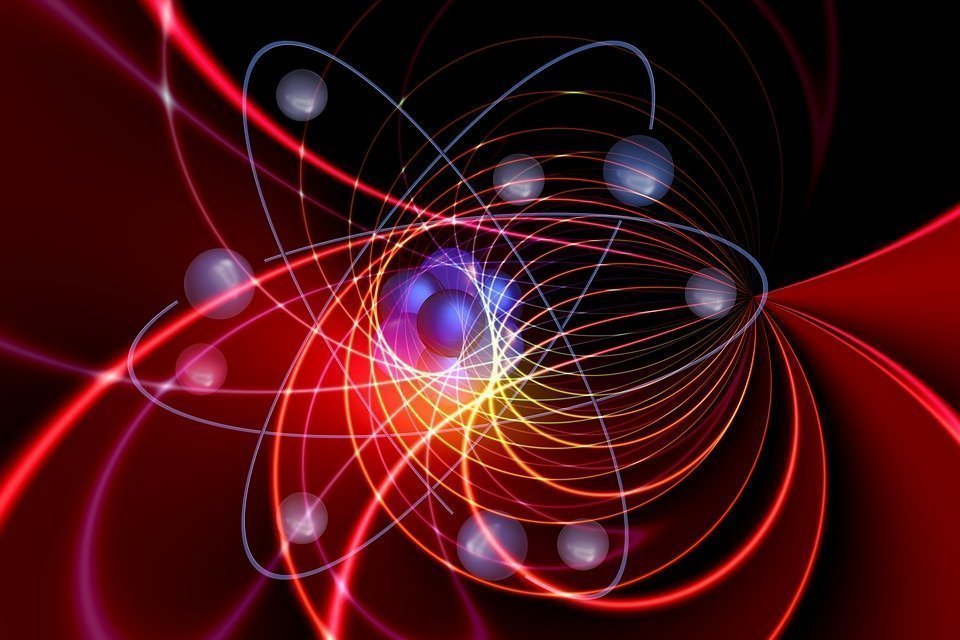
Researchers at MIT have recently demonstrated that utilizing artificial intelligence to simulate aspects of particles and nuclear physics theories can lead to faster algorithms, and therefore faster discoveries when it comes to theoretical physics. The MIT research team combined theoretical physics with AI models to accelerate the creation of samples that simulate interactions between neutrons, protons, and nuclei.
There are four fundamental forces that govern the universe: gravity, electromagnetism, the weak force, and the strong force. The strong, weak, and electromagnetic forces are studied through particle physics. The traditional method of studying particle interactions requires running numerical simulations of these interactions between particles, typically taking place at 1/10th or 1/100th the size of a proton. These studies can take a long time to complete due to limited computing power, and there are many problems that physicists know how to tackle in theory yet cannot address to said computational limitations.
MIT Physics professor Phiala Shanahan is the head of a research group that uses machine learning models to create new algorithms that can speed up particle physics studies. The symmetries found within physics theories (features of the physical system that stay constant even as conditions change) can be incorporated into machine learning algorithms to produce algorithms more suited to particle physics studies. Shanahan explained that the machine learning models aren’t being used to process large amounts of data, rather they are being used to integrate particle symmetries, and the inclusion of these attributes within a model means that computations can be done more quickly.
The research project was lead by Shanahan and it includes several members of the Theoretical Physics team at NYU, as well as machine-learning researchers from Google DeepMind. The recent study is just one of a series of ongoing and recently completed studies aimed at leveraging the power of machine learning to solve theoretical physics problems that are currently impossible with modern computation schemas. According to MIT graduate student Gurtej Kanwar, the problems that the machine-learning boosted algorithms are trying to solve will help scientists understand more about particle physics, and they are useful in making comparisons against results derived by large-scale particle physics experiments (like those conducted at CERN’s Large Hadron Collider). By comparing the results of the large-scale experiments with the AI algorithms, scientists can get a better idea of how their physics models should be constrained, and when those models break down.
Currently, the only method that scientists can reliably use to investigate the Standard Model of particle physics is one where samples/snapshots are taken of fluctuations occurring in a vacuum. Researchers can gain insight into the properties of the particles and what happens when those particles collide. However, taking samples like this is expensive and it is hoped that AI techniques can make taking samples a cheaper, more efficient process. The snapshots taken of the vacuum can be used much like image training data in a computer vision AI model. The quantum snapshots are used to train a model that can create samples in a much more efficient manner, accomplished by taking samples in an easy-to-sample space and running the samples through the trained model.
The research has created a framework intended to streamline the process of creating machine-learning models based on physics symmetries. The framework has already been applied to simpler physics problems and the research team is now attempting to scale up their approach to work with cutting edge calculations. As Kanwar explained via Phys.org:
“I think we have shown over the past year that there is a lot of promise in combining physics knowledge with machine learning techniques. We are actively thinking about how to tackle the remaining barriers in the way of performing full-scale simulations using our approach. I hope to see the first application of these methods to calculations at scale in the next couple of years.”
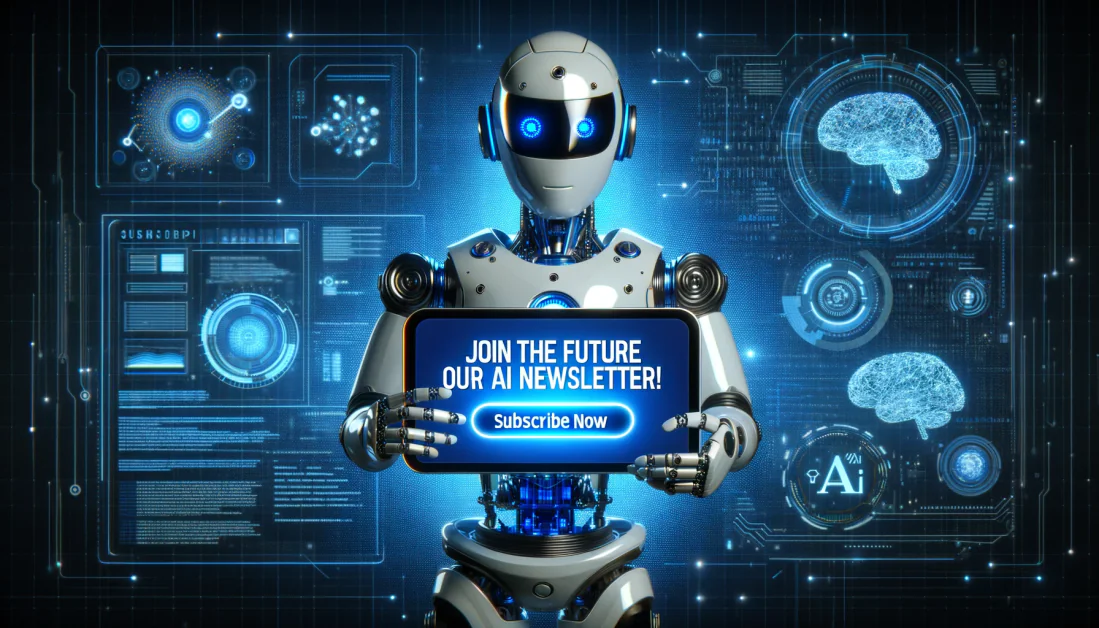
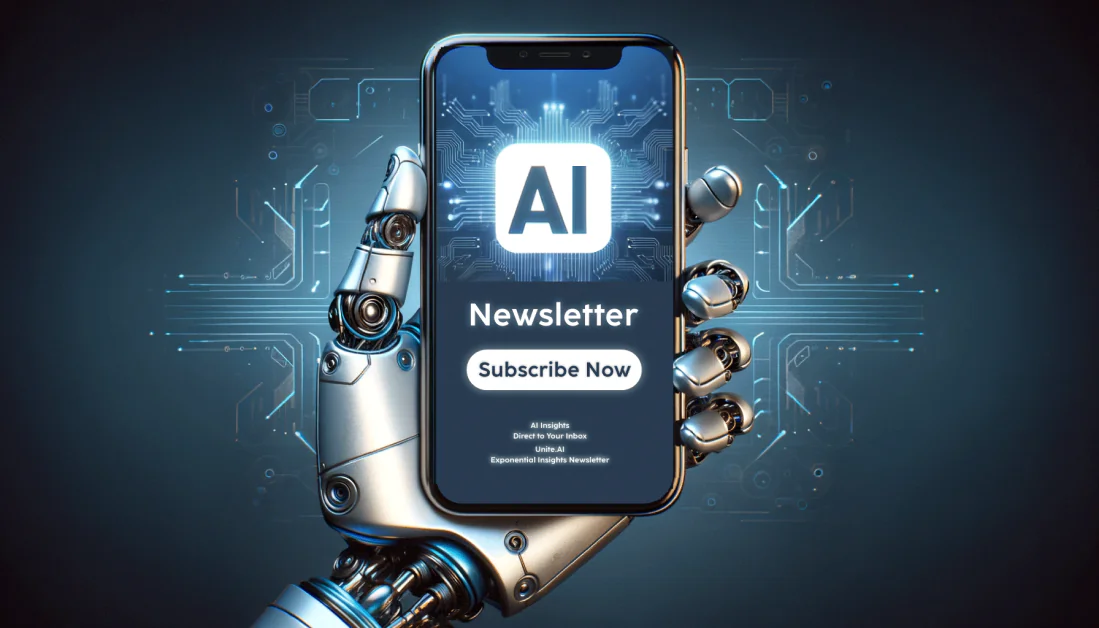