Artificial Intelligence
AI Teaches Itself Laws of Physics
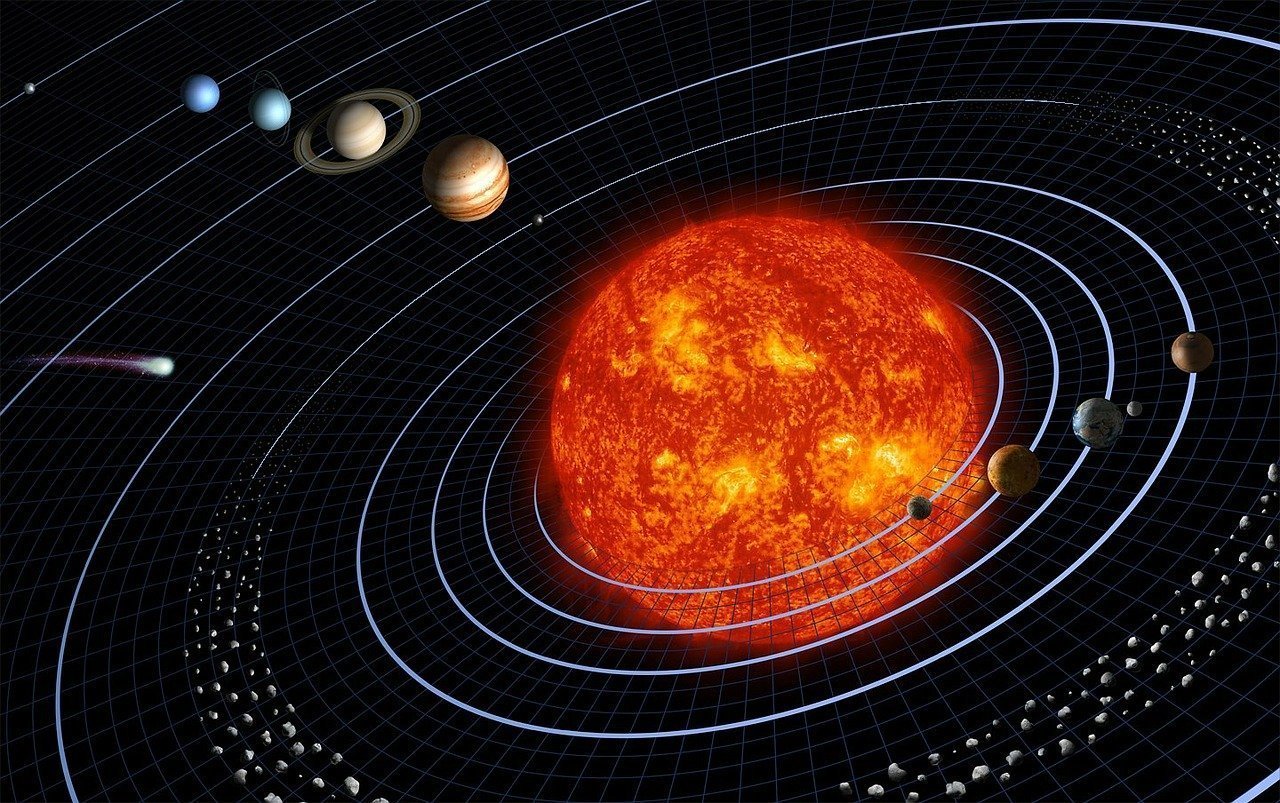
In what is a monumental moment in both AI and physics, a neural network has “rediscovered” that Earth orbits the Sun. The new development could be critical in solving quantum-mechanics problems, and the researchers hope that it can be used to discover new laws of physics by identifying patterns within large data sets.
The neural network, named SciNet, was fed measurements showing how the Sun and Mars appear from Earth. Scientists at the Swiss Federal Institute of Technology then tasked SciNet with predicting where the Sun and Mars would be at different times in the future.
The research will be published in Physical Review Letters.
Designing the Algorithm
The team, including Physicist Renato Renner, set out to make the algorithm capable of distilling large data sets into basic formulae. This is the same system used by physicists when coming up with equations. In order to do this, the researchers had to base the neural network on the human brain.
The formulas that were generated by SciNet placed the Sun at the center of our solar system. One of the remarkable aspects of this research was that SciNet did this similarly to how astronomer Nicolaus Copernicus discovered heliocentricity.
The team highlighted this in a paper published on the preprint repository arXiv.
“In the 16th century, Copernicus measured the angles between a distant fixed star and several planets and celestial bodies and hypothesized that the Sun, and not the Earth, is in the centre of our solar system and that the planets move around the Sun on simple orbits,” the team wrote. “This explains the complicated orbits as seen from Earth.”
The team tried to get SciNet to predict the movements of the Sun and Mars in the simplest way possible, so SciNet uses two sub-networks to send information back and forth. One of the networks analyzes the data and learns from it, and the other one makes predictions and tests accuracy based on that knowledge. Because these networks are connected together by just a few links, information is compressed and communication is simpler.
Conventional neural networks learn to identify and recognize objects through huge data sets, and they generate features. Those are then encoded in mathematical ‘nodes,’ which are considered the artificial equivalent of neurons. Unlike physicists, neural networks are more unpredictable and difficult to interpret.
Artificial Intelligence and Scientific Discoveries
One of the tests involved giving the network simulated data about the movements of Mars and the Sun, as seen from Earth. The orbit of Mars around the Sun appears unpredictable and often reverses its course. It was in the 1500s when Nicolaus Copernicus discovered that simpler formulas could be used to predict the movements of the planets orbiting the Sun.
When the neural network “discovered” similar formulas for Mar’s trajectory, it rediscovered one of the most important pieces of knowledge in history.
Mario Krenn is a physicist at the University of Toronto in Canada, and he works on using artificial intelligence to make scientific discoveries.
SciNet rediscovered “one of the most important shifts of paradigms in the history of science,” he said.
According to Renner, humans are still needed to interpret the equations and determine how they are connected to the movement of the planets around the Sun.
Hod Lipson is a roboticist at Columbia University in New York City.
“This work is important because it is able to single out the crucial parameters that describe a physical system,” he says. “I think that these kinds of techniques are our only hope of understanding and keeping pace with increasingly complex phenomena, in physics and beyond.”
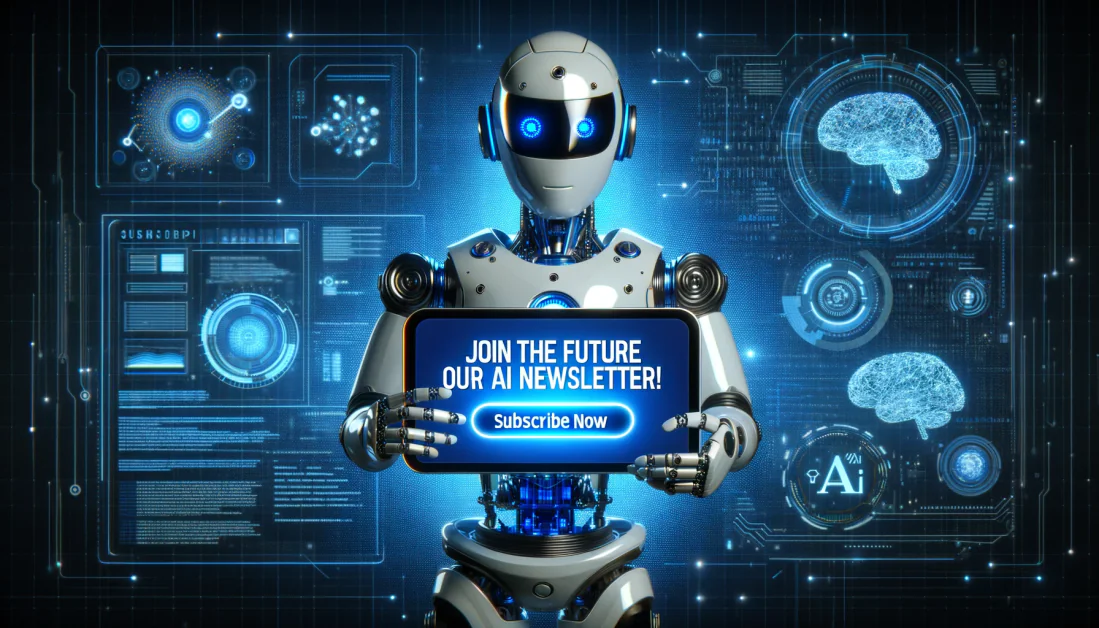
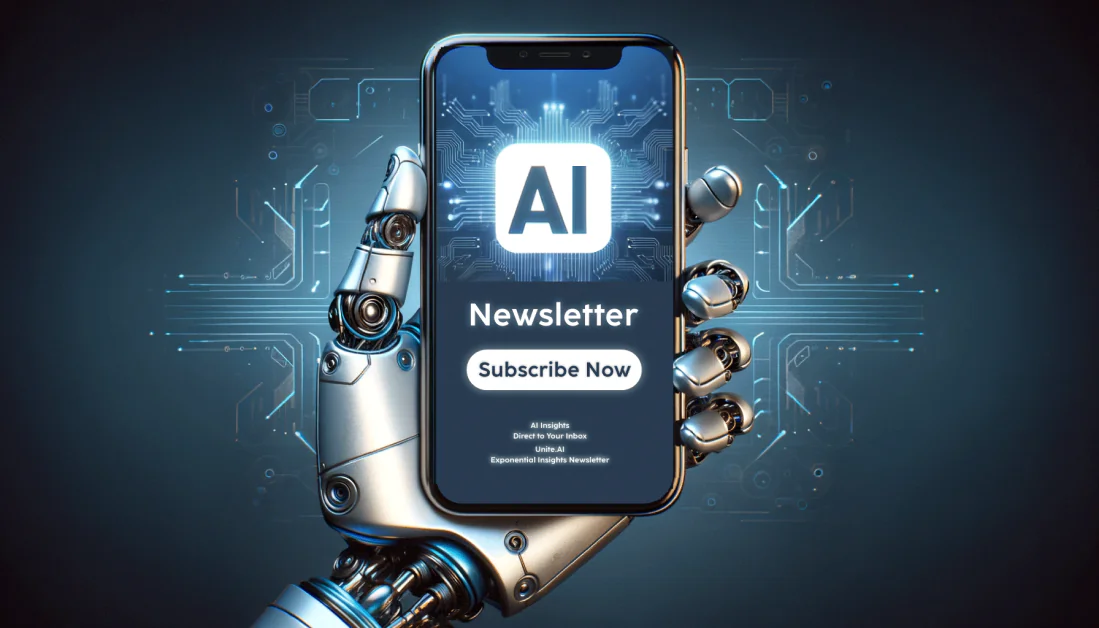