Artificial Intelligence
Scalable Autonomous Vehicle Safety Tools Developed By Researchers
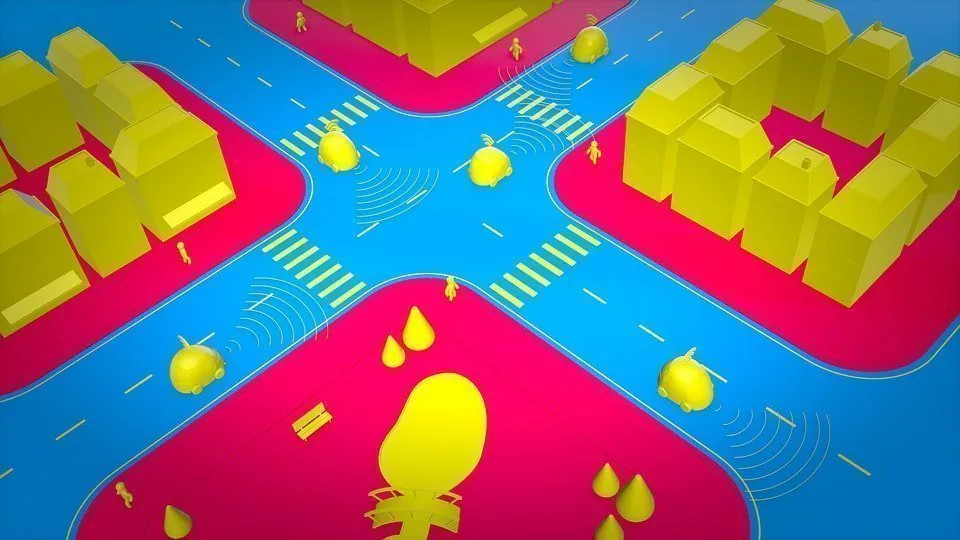
As the speed of autonomous vehicle manufacturing and deployment increases, the safety of autonomous vehicles becomes even more important. For that reason, researchers are investing in the creation of metrics and tools to track the safety of autonomous vehicles. As reported by ScienceDaily, a research team from the University of Illinois at Urbana-Champaign have used machine learning algorithms to create a scalable autonomous vehicle safety analysis platform, utilizing both hardware and software improvements to do so.
Improving the safety of autonomous vehicles has remained one of the more difficult tasks in AI, because of the many variables involved in the task. Not only are the sensors and algorithms involved in the vehicle extremely complex, but there are many external conditions that are constantly in flux, such as road conditions, topography, weather, lighting and traffic.
The landscape and algorithms of autonomous vehicles are both constantly changing, and companies need a way to keep up with the changes and respond to new issues. The Illinois researchers are working on a platform that lets companies address recently identified safety concerns in a quick, cost-effective method. However, the sheer complexity of the systems that drive autonomous vehicles make this a massive undertaking. The research team is designing a system that will be able to keep track of and update autonomous vehicle systems that contain dozens of processors and accelerators running millions of lines of code.
In general, autonomous vehicles drive quite safely. However, when a failure or unexpected event occurs, an autonomous vehicle is currently more likely to get in an accident than human drivers, as the vehicle often has trouble negotiating sudden emergencies. While it is admittedly difficult to quantify how safe autonomous vehicles are and what is to blame for accidents, it is obvious that failures of a vehicle going at 70 mph down a road could prove extremely dangerous, hence the need to improve the handling of emergencies by autonomous vehicles.
Saurabh Jha, a doctoral candidate and one of the researchers involved with the program, explained to ScienceDaily the need to improve failure handling in autonomous vehicles. Jha explained:
“If a driver of a typical car senses a problem such as vehicle drift or pull, the driver can adjust his/her behavior and guide the car to a safe stopping point. However, the behavior of the autonomous vehicle may be unpredictable in such a scenario unless the autonomous vehicle is explicitly trained for such problems. In the real world, there are an infinite number of such cases.”
The researchers are aiming to solve this problem by gathering and analyzing data involving safety reports submitted by autonomous vehicle companies. Companies like Waymo and Uber are required to submit reports to the DMV in California at least annually. These reports contain data on statistics like how far cars have driven, how many accidents occured, and what conditions the vehicles were operating under.
The University of Illinois research team analyzed reports covering the years 2014 to 2017. During this period, autonomous vehicles drove around 1,116,000 miles distributed across 144 different vehicles. According to the findings of the research team, when compared with the same distance driven by human drivers, accidents were 4000 times more likely to occur. The accidents may imply that the AI of the vehicle failed to properly disengage and avoid the accident, relying instead on the human driver to take over.
It’s difficult to diagnose potential errors in the hardware or software of the autonomous vehicle because many errors will manifest only under the correct conditions. It also isn’t feasible to conduct tests under every possible condition that could occur on the road. Instead of collecting data on hundreds of thousands of real miles logged by autonomous vehicles, the research team is utilizing simulated environments to drastically reduce the amount of money and time spent in generating data for the training of AVs.
The research team uses the generated data to explore situations where AV failures can happen and safety issues can occur. It appears that utilizing the simulations can genuinely help companies find safety risks they wouldn’t be able to otherwise. For instance, when the team tested the Apollo AV, created by Baidu, they isolated over 500 instances where the AV failed to handle an emergency situation and an accident occurred as a result. The research team hopes that other companies will make use of their testing platform and improve the safety of their autonomous vehicles.