Artificial Intelligence
Researchers Develop Autonomous Systems Capable of Sensing Changes in Shadows
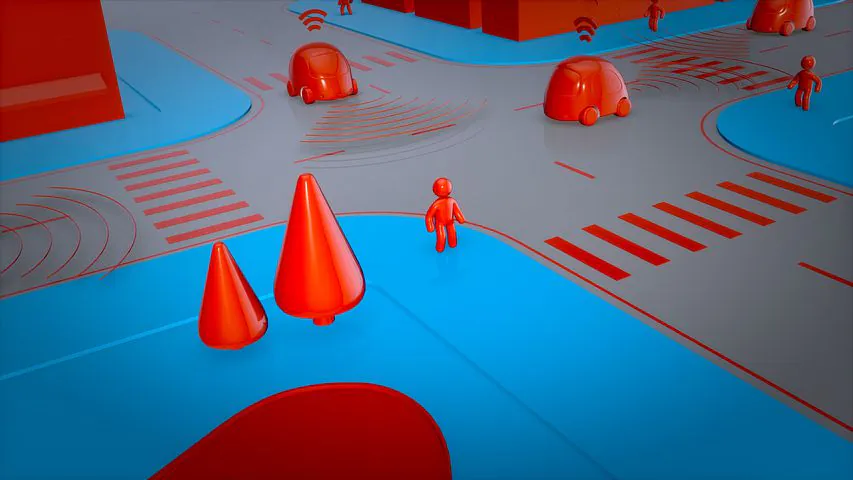
Engineers at MIT have developed a new system that is extremely important for autonomous vehicles and their safety. The system is capable of sensing small changes in shadows on the ground, and it can determine if there are any moving objects around the corner.
One of the major goals for any company seeking to create autonomous vehicles is increased safety. Engineers are constantly working on making the vehicles better at avoiding collisions with other cars or pedestrians, especially those that are coming around a building’s corner.
The new system also has the potential to be used on eventual robots that navigate hospitals. These robots could deliver medication or supplies throughout the hospital, and the system would help them avoid hitting people.
A paper will be presented next week at the International Conference on Intelligent Robots and Systems (IROS). It includes descriptions of the successful experiments conducted by the researchers, including an autonomous car maneuvering around a parking garage and stopping when approaching another vehicle.
The current system is often LIDAR, which is able to detect visible objects by more than a half of a second. According to the researchers, fractions of a second can make a huge difference in fast-moving autonomous vehicles.
“For applications where robots are moving around environments with other moving objects or people, our method can give the robot an early warning that somebody is coming around the corner, so the vehicle can slow down, adapt its path, and prepare in advance to avoid a collision,” adds co-author Daniela Rus, director of the Computer Science and Artificial Intelligence Laboratory (CSAIL) and the Andrew and Erna Viterbi Professor of Electrical Engineering and Computer Science. “The big dream is to provide ‘X-ray vision' of sorts to vehicles moving fast on the streets.”
The new autonomous system has only been tested indoors. In these conditions, lighting conditions are lower, and the robotic speeds are slower. The autonomous system can analyze and sense shadows much easier in this environment.
The paper was compiled by Daniela Rus; first author Felix Naser, who is a former CSAIL researcher; Alexander Amini, a CSAIL graduate student; Igor Gilitschenski, a CSAIL postdoc; graduate Christina Liao; Guy Rosman of the Toyota Research Institute; and Sertac Karaman, associate professor of aeronautics and astronautics at MIT.
ShadowCam System
Prior to the new developments, the researchers already had a system called “ShadowCam.” The system is able to identify and classify changes in shadows on the ground through the use of computer-vision techniques. The earlier versions of the system were developed by MIT professors William Freeman and Antonio Torralba. The two professors were not co-authors on the IROS paper, and their work was presented in 2017 and 2018.
ShadowCam utilizes video frames from a target-specific camera, and it is able to detect any changes in light intensity over time. This tells the system if something is moving further away or getting closer, and it then analyzes the information and classifies each image as a stationary object or moving one. This allows the system to proceed in the best possible way.
The ShadowCam was tweaked and changed to be used on autonomous vehicles. Originally, it used augmented reality labels termed “AprilTags,” which were like QR codes. ShadowCam used these to focus on certain clusters of pixels to determine if there were any shadows present. However, this system proved to be impossible to utilize in real-world scenarios.
Because of this, the researchers created a new process that uses image registration and a visual-odometry technique together. Image registration overlays multiple images in order to identify any variations.
The visual-odometry technique that the researchers use is called “Direct Sparse Odometry” (DSO), and it operates similarly to the AprilTags. DSO uses a 3D-print cloud, and it plots the different features of an environment on it. A computer-vision pipeline then locates a region of interest such as a floor.
ShadowCam used DSO-image-registration and overlays all of the images from the same viewpoint of the robot. The robot, moving or staying still, is then able to zero in on the same patch of pixels where there is a shadow.
What’s Next
The researchers will continue to work on this system, and they will focus on the differences between indoor and outdoor lighting conditions. Ultimately, the team wants to increase the speed of the system as well as automate the process.
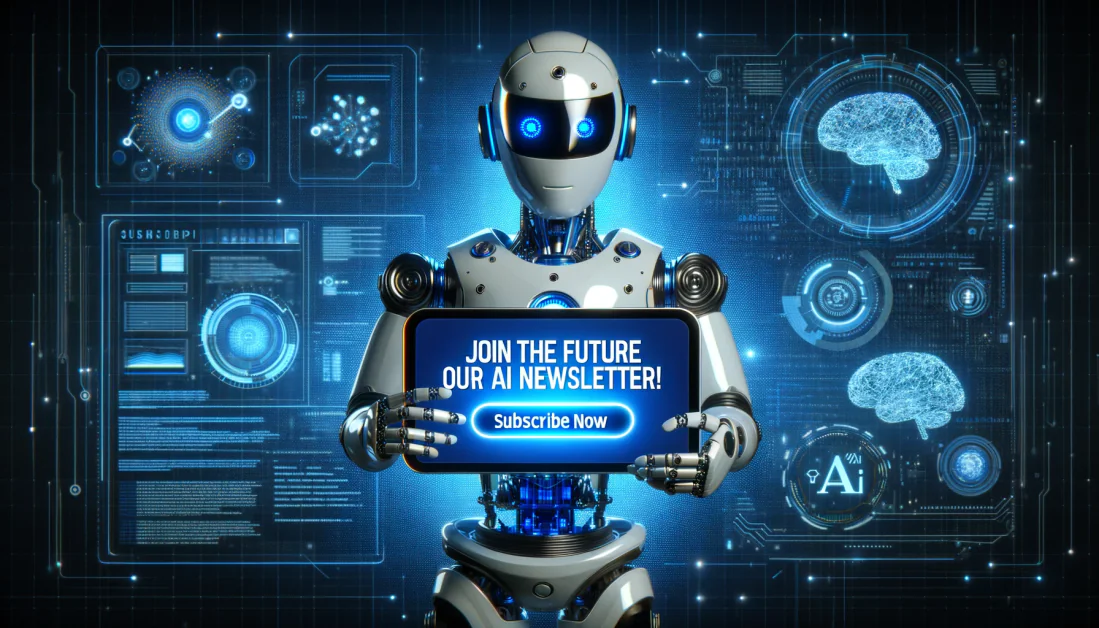
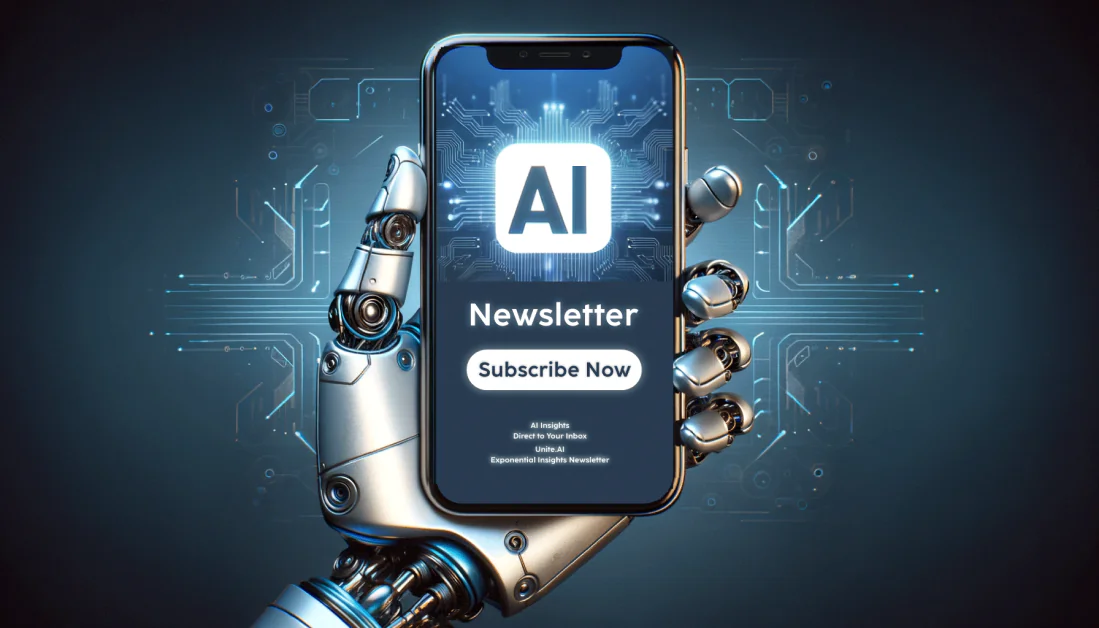