Artificial Intelligence
Researchers Train An AI To Predict The Smell Of Chemicals
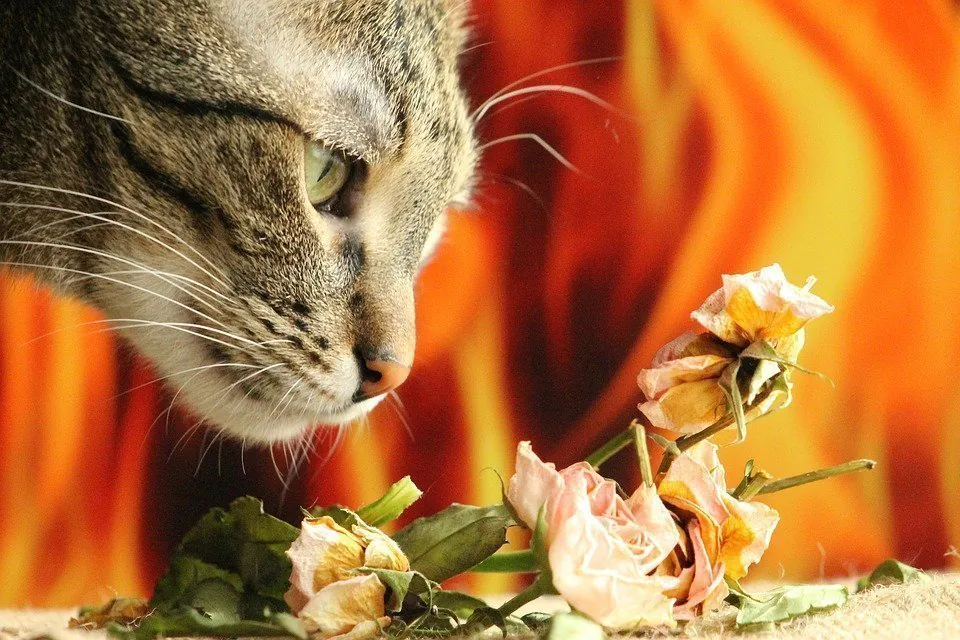
A recent paper published by researchers at Google Brain demonstrates how researchers managed to train an AI to predict the smell of objects, based on the structure of the chemicals passed into the network. As reported by Wired, the researchers are hopeful that their work could help unravel some of the mysteries surrounding the human sense of smell, which is poorly understood in comparison to our other senses.
The differences between smells are complex and a single atom being changed in a molecule can change a smell from pleasant to unpleasant. It’s difficult for researchers to understand the patterns that cause chemical structures to be interpreted by our olfactory senses as pleasant or aversive. In contrast, the patterns of the electromagnetic spectrum that appear as color to our eyes are much more easily quantifiable, with scientists being able to make precise measurements that will tell them what certain wavelengths of light will look like.
Machine learning algorithms excel at finding patterns within data, and for this reason, AI researchers have attempted to use machine learning to gain better insight into how smells are interpreted by the human brain. Attempts to utilize machine learning algorithms to quantify smell include the DREAM Olfaction Prediction Challenge carried out in 2015. Several studies took the data from the challenge and tried to generate natural language descriptions of mono-molecular odorants.
The recent study, published in Arxiv, catalogs the Google Brain researcher’s attempts to quantify smell using neural networks. The researchers utilized a Graph Neural Network or GNN. Graph Neural Networks are capable of interpreting graph data, which are data structures comprised of nodes and edges. Graphs are commonly used to represent networks or relationships between individual data points. In the context of a social network, a graph would have each person in the network represented by a node or vertex. Such graphs are used by social media companies to predict people on the peripherals of your current network and suggest new friends.
For the purposes of interpreting smells, the researchers trained the network on thousands of molecules, each matched with a natural language descriptor. The GNN was able to interpret the data and pick up on patterns in the structure of the molecules. The descriptors used by the researchers were phrases like “sweet”, “smoky”, or “woody”. Approximately two-thirds of the over 5,000 molecules that were compiled by the researchers were used to train the model, while the remaining third was used to test the model.
The model the researchers trained worked so well that once the first iteration was completed, performance already matched the peak performance achieved by other groups of researchers who tried to assign natural language labels to chemical structures.
Alex Wiltschko, one of the researchers who worked on the project, acknowledges that there are a couple of limitations to their current approach. For one, the AI may distinguish differences between chemical structures that humans would describe as being the same, calling two different chemicals “earthy” or “woody” in nature, even though the AI classifies them differently. Another issue with the classifier is that it doesn’t distinguish between chiral pairs, which are molecules that are mirror images of each other. The different orientations mean they have different smells, but the model currently doesn’t see them as being different.
The research team aims to address these limitations in their future work. The research still has a long way to go, but it is a step towards understanding what features of a molecule correspond with our perception of certain smells. The Google Brain team isn't the only research team to be working on applications of AI aimed at recognizing scents. Other AI experiments involving scent include IBM's experiments with AI-generated perfumes and an experiment by Russian scientists to detect potentially toxic mixtures of gas.
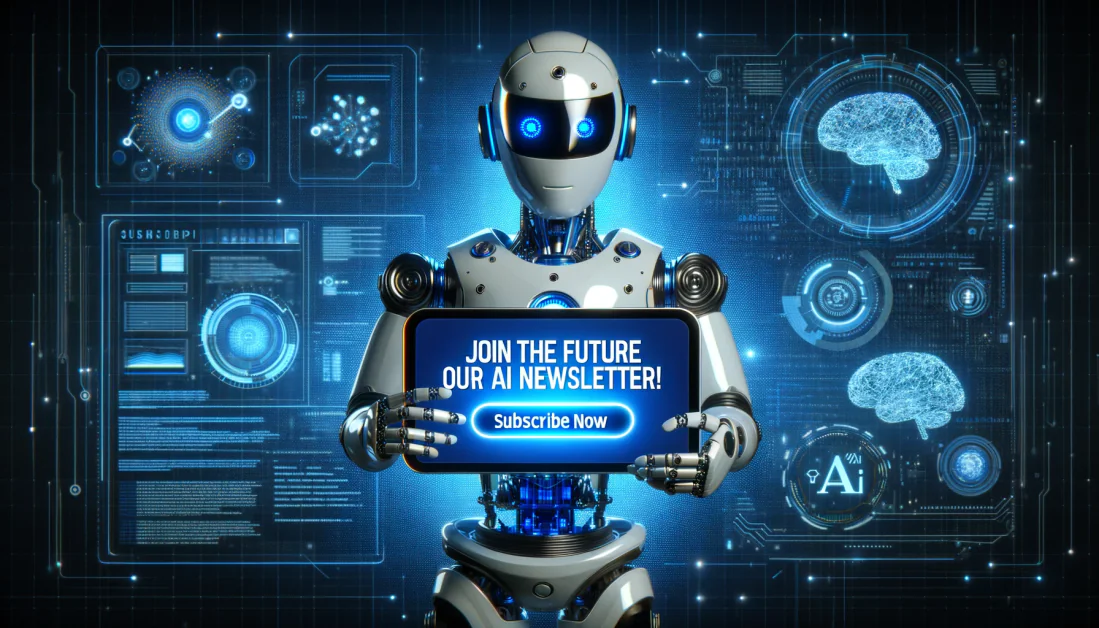
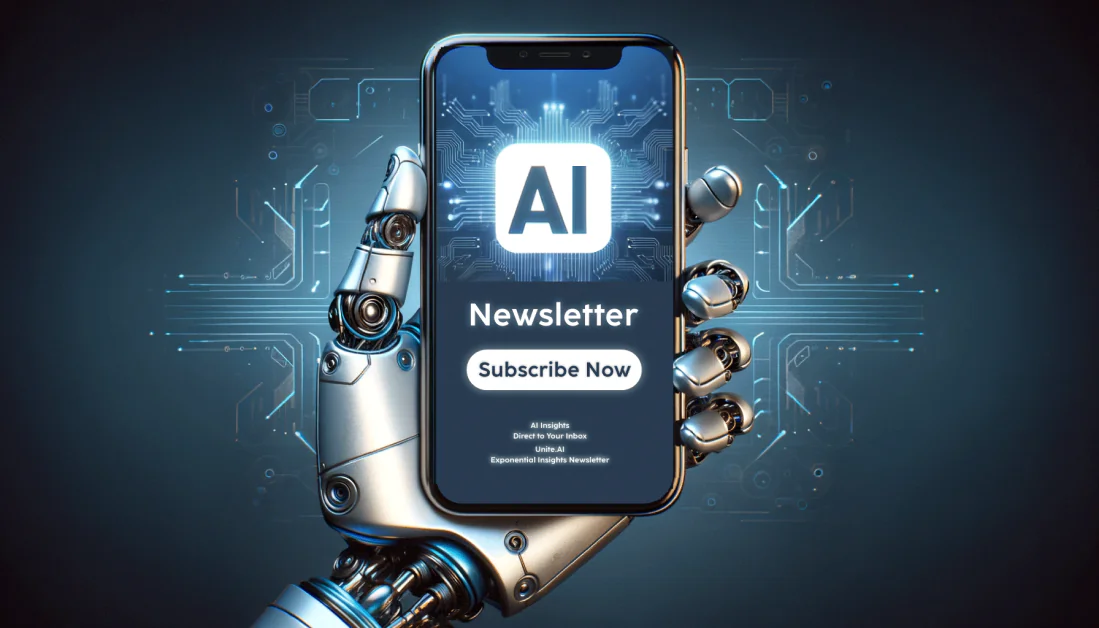