Artificial Intelligence
DeepScribe AI Can Help Translate Ancient Tablets
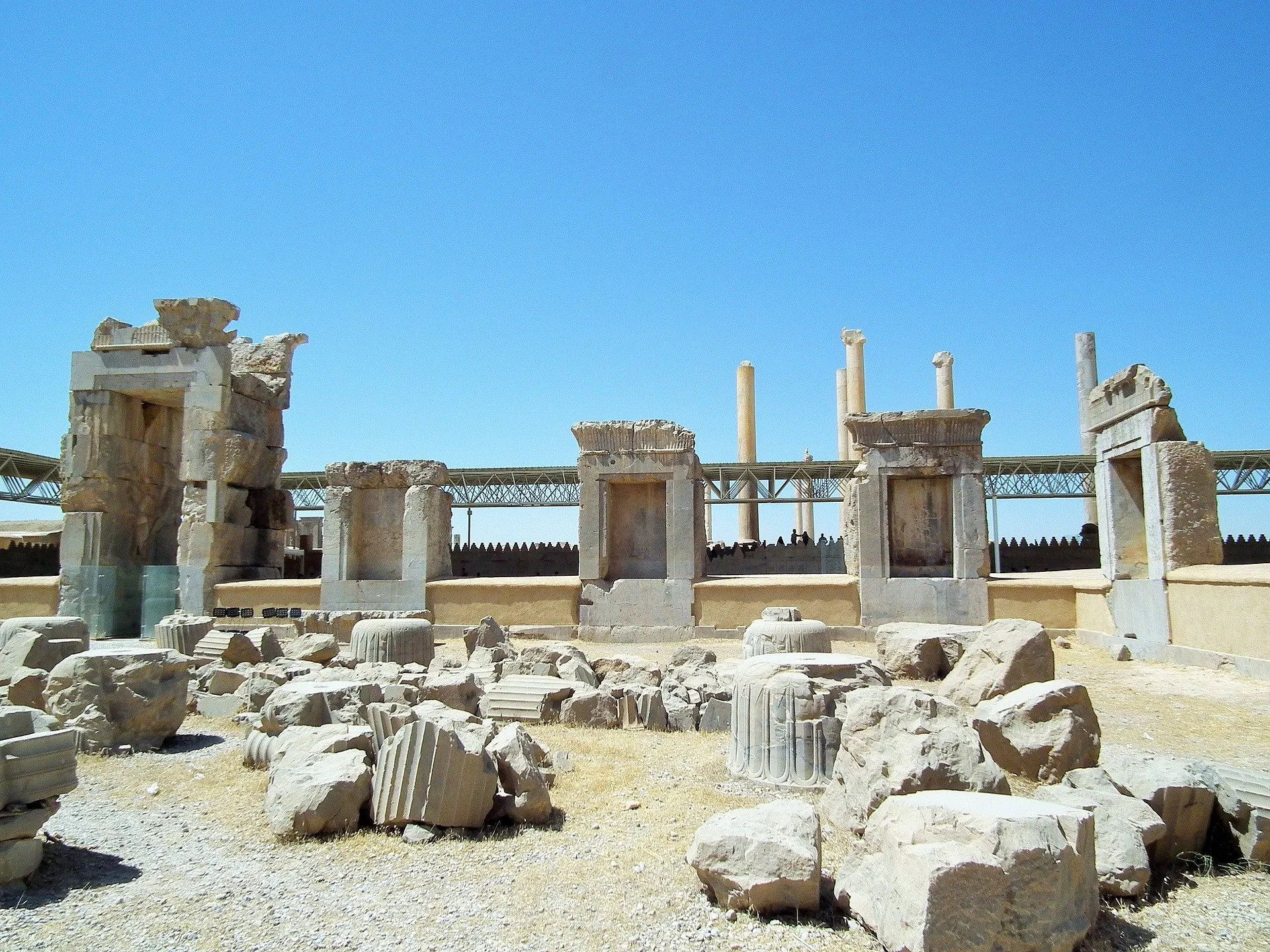
Researchers from the University of Chicago’s Oriental Institute and the Department of Computer Science have collaborated to design an AI that can help decode tablets from ancient civilizations. According to Phys.org, the AI is called DeepScribe and was trained on over 6,000 annotated images pulled from the Persepolis Fortification Archive, when it is complete the AI model will be able to interpret unanalyzed tablets, making studying ancient documents easier.
Experts who study ancient documents, like the researchers who are studying the documents created during the Achaemenid Empire in Persia, need to translate ancient documents by hand, a long process that is prone to errors. Researchers have been using computers to assist in interpreting ancient documents since the 1990s, but the computer programs that were used were of limited help. The complex cuneiform characters, as well as the three-dimensional shape of the tablets, put a cap on how useful the computer programs could be.
Computer vision algorithms and deep learning architectures have brought new possibilities to this field. Sanjay Krishnan, from the Department of Computer Science at OI collaborated with associate professor of Assyriology Susanne Paulus to launch the DeepScribe program. The researchers oversaw a database management platform called OCHRE, which organized data from archaeological excavations. The goal is to create an AI tool that is both extensive and flexible, able to interpret scripts from digfferent geographical regions and time periods.
As Phys.org reported, Krishnan explained that the challenges of recognizing script, which archaeological researchers face, are essentially the same challenges faced by computer vision researchers:
“From the computer vision perspective, it's really interesting because these are the same challenges that we face. Computer vision over the last five years has improved so significantly; ten years ago, this would have been hand wavy, we wouldn't have gotten this far. It's a good machine learning problem, because the accuracy is objective here, we have a labeled training set and we understand the script pretty well and that helps us. It's not a completely unknown problem.”
The training set in question is the result of taking the tablets and translations, from over approximately 80 years of the archaeological research done at OI and U Chicago and making high-resolution annotated images from them. Currently, the training data is approximately 60 terabytes in size. Researchers were able to use the dataset and create a dictionary of over 100,000 individually identified signs that the model could learn from. When the trained model was tested on an unseen image set, the model achieved approximately 80% accuracy.
While the team of researchers is attempting to increase the accuracy of the model, even 80% accuracy can assist in the process of transcription. According to Paulus, the model could be used to identify or translate highly repetitive parts of the documents, letting experts spend their time interpreting the more difficult parts of the document. Even if the model can’t say with certainty what a symbol translates to, it can give researchers probabilities, which already puts them ahead.
The team is also aiming to make DeepScribe a tool that other archeologists can use in their projects. For instance, the model could be retrained on other cuneiform languages, or the model could make informed estimates about the text on damaged or incomplete tablets. A sufficiently robust model could potentially even estimate the age and origin of tablets or other artifacts, something typically done with chemical testing.
The DeepScribe project is funded by the Centre for the Development of Advanced Computing (CDAC). Computer vision has been used in other CDAC-funded projects as well, like a project intended to recognize style in works of art and a project designed to quantify biodiversity in marine bivalves. The team of researchers is also hoping their collaboration will lead to future collaborations between the Department of Computer Science and OI at the University of Chicago.
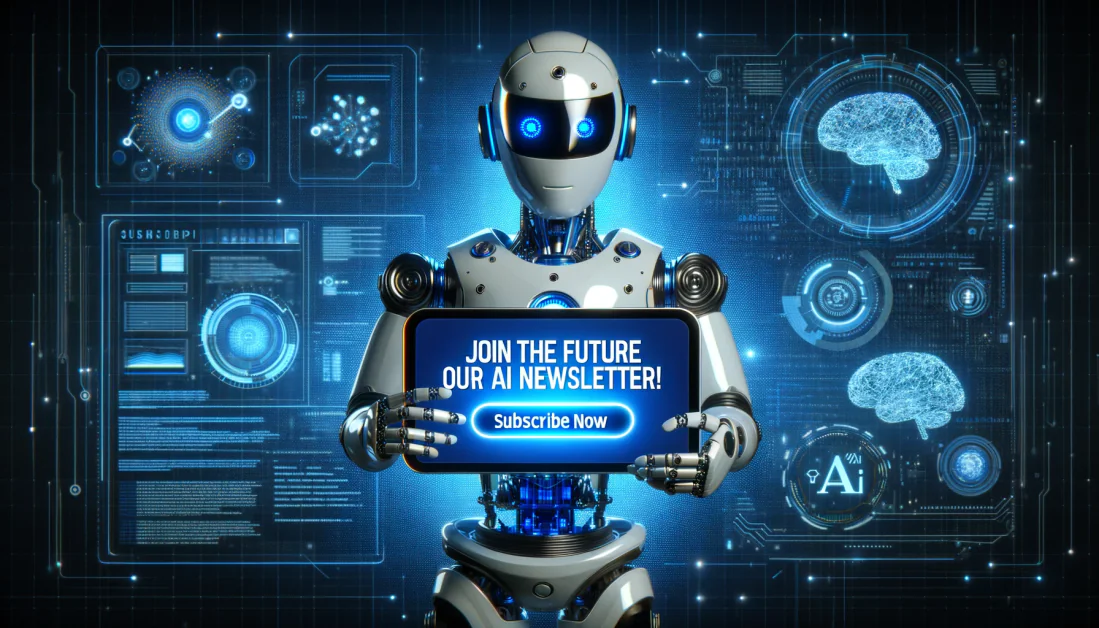
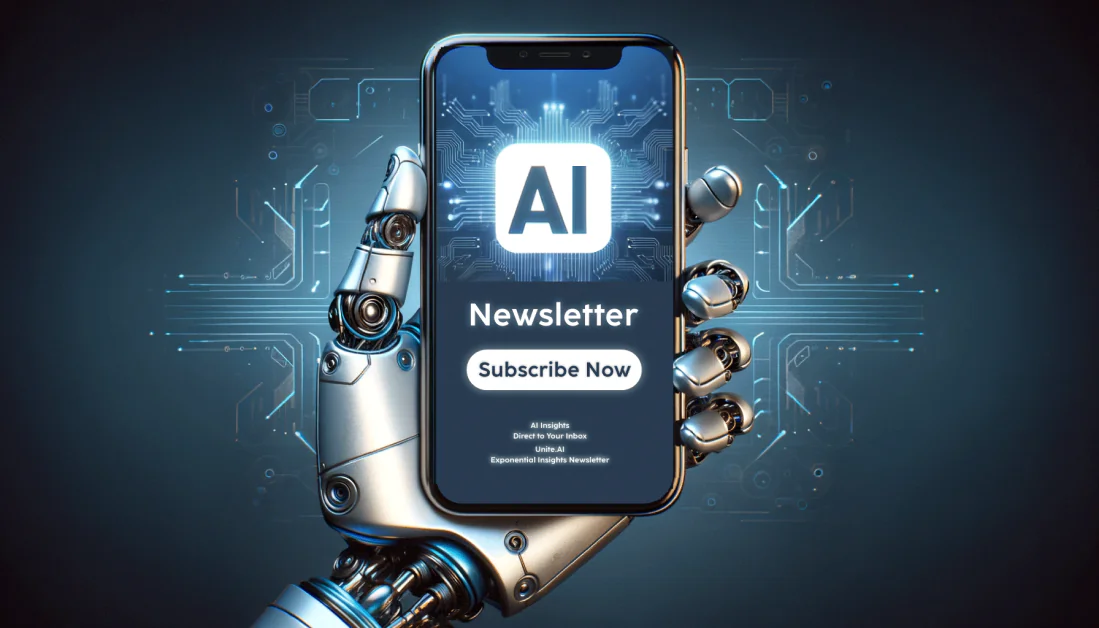