Artificial Intelligence
Baidu’s AI Technology Being Used to Combat Coronavirus
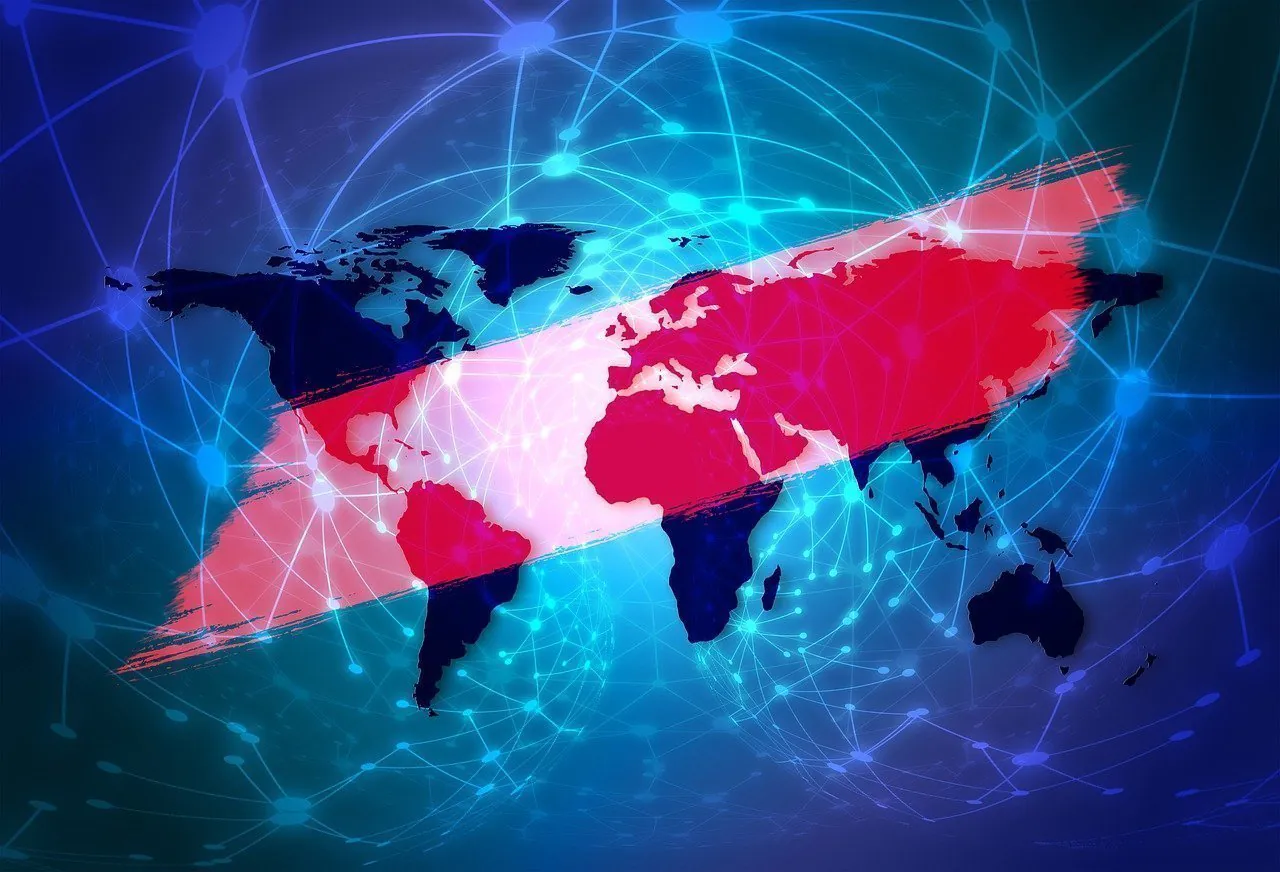
As Coronavirus continues to spread rapidly around the globe, the Dow tries to recover from its worst day in three decades, and some governments botch their responses, new approaches involving artificial intelligence (AI) are being implemented. With the virus now present in more than 100 countries and infections increasing exponentially, many argue that AI could have prevented much of the damage.
Some of those new approaches are coming from Baidu. Headquartered in Beijing's Haidian District, Baidu is one of the largest AI and internet companies in the world.
Vaccine Development
Covid-19 is unpredictable and highly-contagious, raising the importance of a vaccine. In order to create one, those in the medical and academic fields are analyzing the structure of the virus, but it is difficult due to Covid-19’s ability to rapidly mutate.
Back in 2019, Baidu developed its Linearfold algorithm and published in partnership with Oregon State University and the University of Rochester. The company has now made it available to scientific and medical teams in order to help fight against the virus.
The Linearfold algorithm is fast at predicting a virus’s secondary RNA structure, which is important since scientists can better understand how viruses spread across species through analyzing the secondary structural changes between homologous RNA virus sequences. The algorithm was used by AI scientists at Baidu to predict the secondary structure prediction for the Covid-19 RNA sequence. Because of the algorithm, the overall analysis time was able to be reduced from 55 minutes to 27 seconds.
With a faster structural analysis, a potential mRNA vaccine can be developed in a shorter amount of time, and the vaccine will be more stable and effective. The Linearfold algorithm has been provided to the broader community, and Baidu works with health and academic institutions by sharing computation resources, providing customized support, and collaborating to optimize mRNA vaccine design.
Haifeng Wang is CTO at Baidu.
“We hope that this powerful ability can be quickly leveraged by our researchers and anti-epidemic experts, and work with society as a whole to help improve the speed of virus research and vaccine development,” Wang says.
Screening and Monitoring Tools
Besides contributing to vaccine development, Baidu has developed various tools for building awareness and screening populations. One of those tools is a non-contact infrared sensor system powered by AI. The system can monitor multiple individual's temperature quickly and detect a person suspected of having a fever.
“Traditional approaches, such as station personnel using frontal medical thermometer devices, can easily cause crowds and increase the risk of cross-infection. Baidu's AI temperature sensor system can quickly screen crowds to improve detection efficiency and accuracy without creating unnecessary risks,” says Wang.
Baidu has also developed the industry’s first open-source model capable of detecting whether or not individuals are wearing masks in crowded areas. The model has a classification accuracy of 97.27%, and it can be used to monitor public response to the outbreak.
Identifying Flow of Travel
Baidu has used AI-power mapping systems and created Baidu Maps’ “Migration Big Data Platform” to track the flow of travel across high-risk areas. AI technology like this can help understand the migration paths of people, including those carrying the virus. By following the movements out of Wuhan, where the outbreak began, the early spread of Covid-19 could be tracked. Technology like this can help with preparedness and response effectiveness.
Baidu’s Autonomous Vehicles
Autonomous vehicles are another way to combat major outbreaks. Baidu has an autonomous vehicle platform called Apollo, and it partnered with Neolix, a local self-driving startup, in order to deliver food and supplies to Beijing Haidian Hospital. Together, they are helping to provide over 100 frontline staff members with food. Baidu Apollo also has low-speed driverless micro-car kits and autonomous driving cloud services that are being offered for free to combat the outbreak.
AI technology like Baidu’s could make a huge difference in the response effort across the globe. However, it needs to be embraced and expanded to make a big difference in a global pandemic. Various companies have similar technology, and the effectiveness of it continues to get better with time.
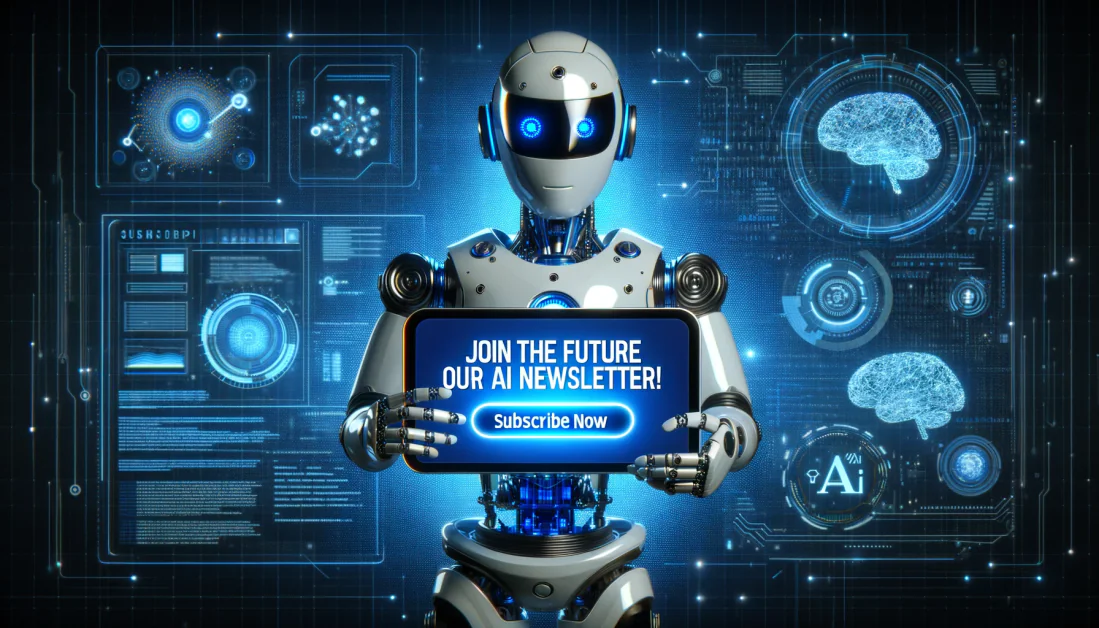
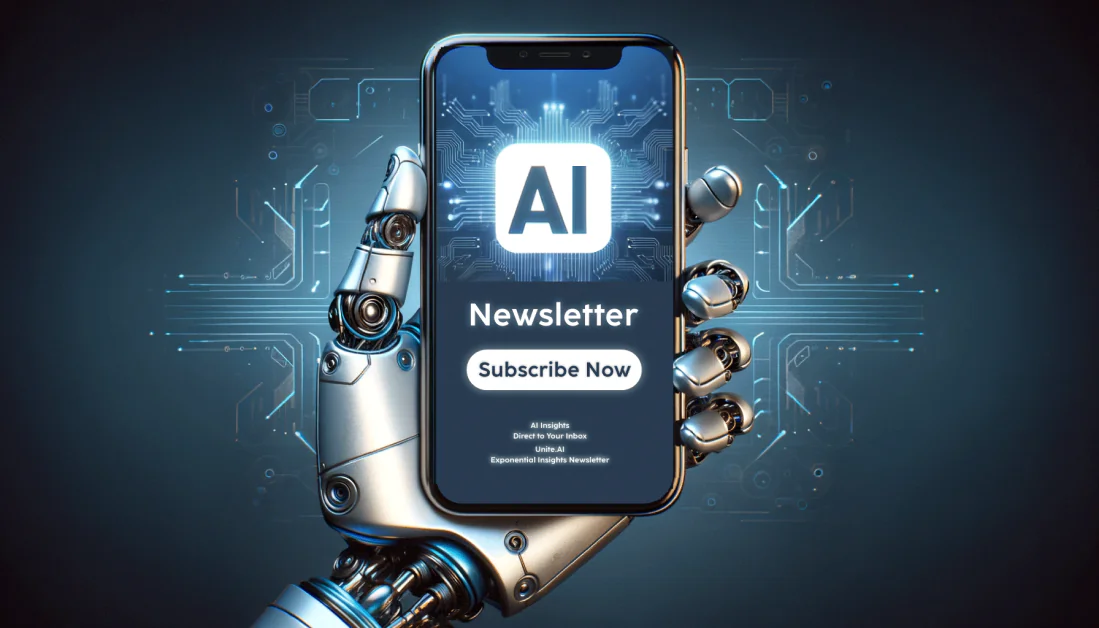