Artificial Intelligence
Why Microsoft’s Orca-2 AI Model Marks a Significant Stride in Sustainable AI?
Despite the notable advancements made by artificial intelligence in the last decade, which include defeating human champions in strategic games like Chess and GO and predicting the 3D structure of proteins, the widespread adoption of large language models (LLMs) signifies a paradigm shift. These models, poised to transform human-computer interactions, have become indispensable across various sectors, including education, customer services, information retrieval, software development, media, and healthcare. While these technological strides unlock scientific breakthroughs and fuel industrial growth, a notable downside for the planet exists.
The process of training and utilizing LLMs consumes an immense amount of energy, resulting in a substantial environmental impact marked by an increased carbon footprint and greenhouse gas emissions. A recent study from the College of Information and Computer Sciences at the University of Massachusetts Amherst revealed that training LLMs can emit over 626,000 pounds of carbon dioxide, roughly equivalent to the lifetime emissions of five cars. Hugging Face, an AI startup, found that the training of BLOOM, a large language model launched earlier in the year, led to 25 metric tons of carbon dioxide emissions. Similarly, Facebook's AI model, Meena, accumulates a carbon footprint on par with the environmental impact of driving a car for more than 240,000 miles throughout its training process.
Despite training LLMs, the demand for cloud computing, crucial for LLMs, now contributes more emissions than the entire airline industry. A single data centre can consume as much power as 50,000 homes. Another study highlights that training a single large language model can release as much CO2 as five cars using energy throughout their entire lifetimes. Predictions suggest that AI emissions will surge by 300% by 2025, emphasizing the urgency of balancing AI progress with environmental responsibility and prompting initiatives to make AI more eco-friendly. To address the adverse environmental impact of AI advancements, sustainable AI is emerging as a crucial field of study.
Sustainable AI
Sustainable AI represents a paradigm shift in the development and deployment of artificial intelligence systems, focusing on minimizing environmental impact, ethical considerations, and long-term societal benefits. The approach aims to create intelligent systems that are energy-efficient, environmentally responsible, and aligned with human values. Sustainable AI focuses on using clean energy for computers, smart algorithms that use less power, and following ethical guidelines to ensure fair and transparent decisions. It is important to note that there is a difference between AI for sustainability and sustainable AI; the former may involve using AI to optimize existing processes without necessarily considering its environmental or societal consequences, while the latter actively integrates principles of sustainability into every phase of AI development, from design to deployment, to create a positive and lasting impact on the planet and society.
From LLMs towards Small Language Models (SLMs)
In the pursuit of sustainable AI, Microsoft is working on developing Small Language Models (SLMs) to align with the capabilities of Large Language Models (LLMs). In this effort, they recently introduce Orca-2, designed to reason like GPT-4. Unlike its predecessor, Orca-1, boasting 13 billion parameters, Orca-2 contains 7 billion parameters using two key techniques.
- Instruction Tuning: Orca-2 improves by learning from examples, enhancing its content quality, zero-shot capabilities, and reasoning skills across various tasks.
- Explanation Tuning: Recognizing limitations in instruction tuning, Orca-2 introduces Explanation Tuning. This involves creating detailed explanations for teacher models, enriching reasoning signals, and improving overall understanding.
Orca-2 uses these techniques to achieve highly efficient reasoning, comparable to what LLMs achieve with many more parameters. The main idea is to enable the model to figure out the best way to solve a problem, whether it's giving a quick answer or thinking through it step by step. Microsoft calls this “Cautious Reasoning.”
To train Orca-2, Microsoft builds a new set of training data using FLAN annotations, Orca-1, and the Orca-2 dataset. They start with easy questions, add in some tricky ones, and then use data from talking models to make it even smarter.
Orca-2 undergoes a thorough evaluation, covering reasoning, text completion, grounding, truthfulness, and safety. The results show the potential of enhancing SLM reasoning through specialized training on synthetic data. Despite some limitations, Orca-2 models show promise for future improvements in reasoning, control, and safety, proving the effectiveness of applying synthetic data strategically in refining the model after training.
Significance of Orca-2 Towards Sustainable AI
Orca-2 represents a significant leap towards sustainable AI, challenging the prevailing belief that only larger models, with their substantial energy consumption, can truly advance AI capabilities. This small language model presents an alternative perspective, suggesting that achieving excellence in language models doesn't necessarily require enormous datasets and extensive computing power. Instead, it underscores the importance of intelligent design and effective integration.
This breakthrough opens new possibilities by advocating a shift in focus—from simply enlarging AI to concentrating on how we design it. This marks a crucial step in making advanced AI more accessible to a broader audience, ensuring that innovation is inclusive and reaches a wider range of people and organizations.
Orca-2 has the potential to significantly impact the development of future language models. Whether it's improving tasks related to natural language processing or enabling more sophisticated AI applications across various industries, these smaller models are poised to bring about substantial positive changes. Moreover, they act as pioneers in promoting more sustainable AI practices, aligning technological progress with a commitment to environmental responsibility.
The Bottom Line:
Microsoft's Orca-2 represents a groundbreaking move towards sustainable AI, challenging the belief that only large models can advance AI. By prioritizing intelligent design over size, Orca-2 opens new possibilities, offering a more inclusive and environmentally responsible approach to advanced AI development. This shift marks a significant step towards a new paradigm in intelligent system design.
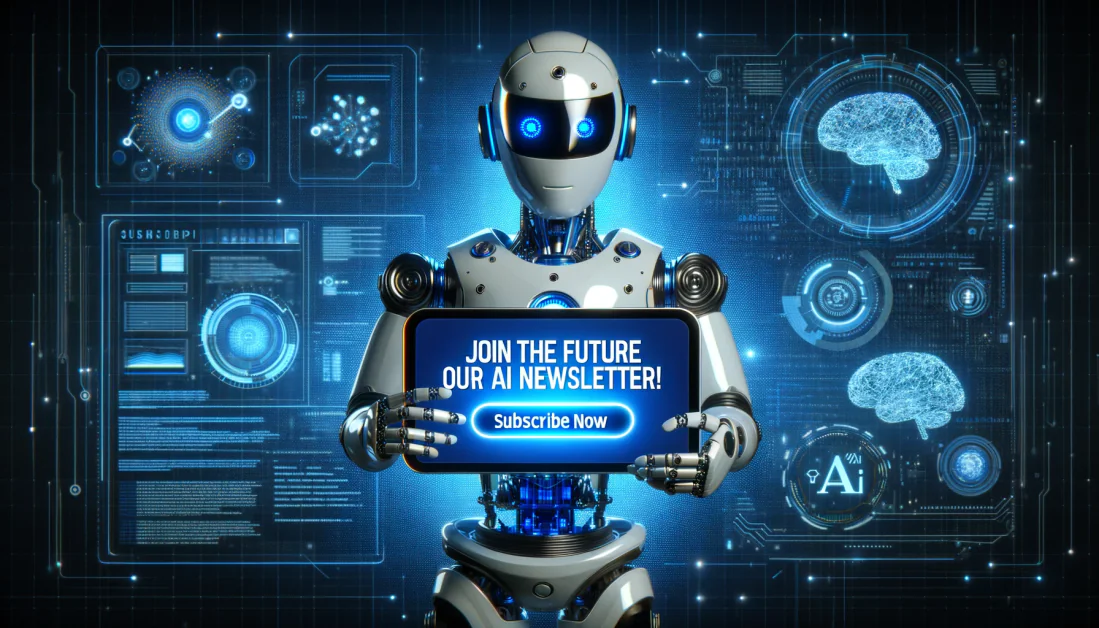
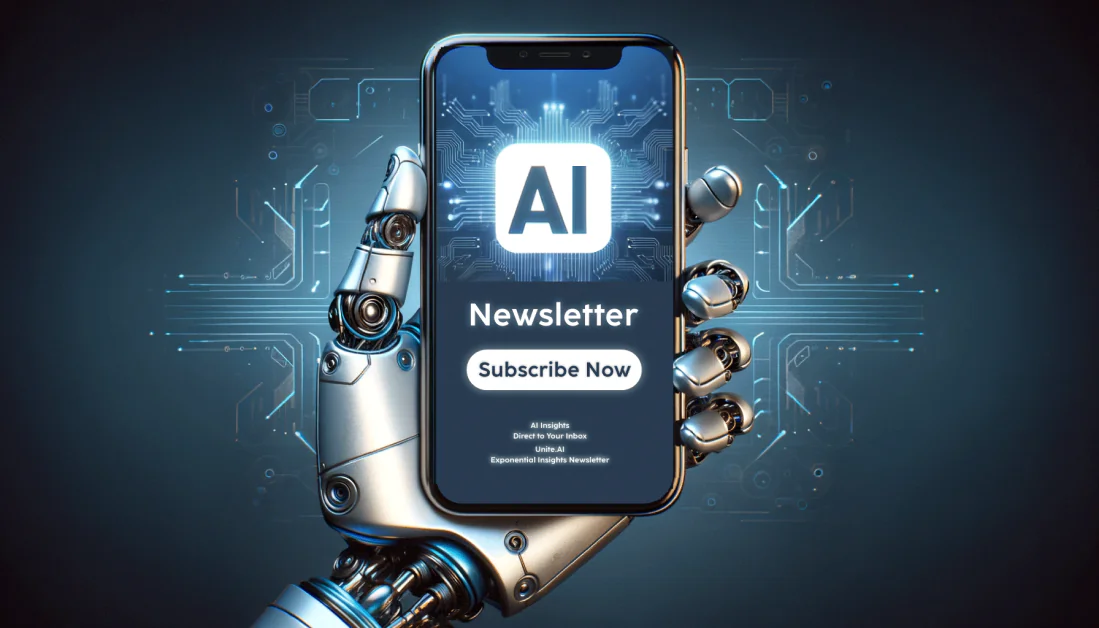