Artificial Intelligence
New Study Unveils Hidden Vulnerabilities in AI
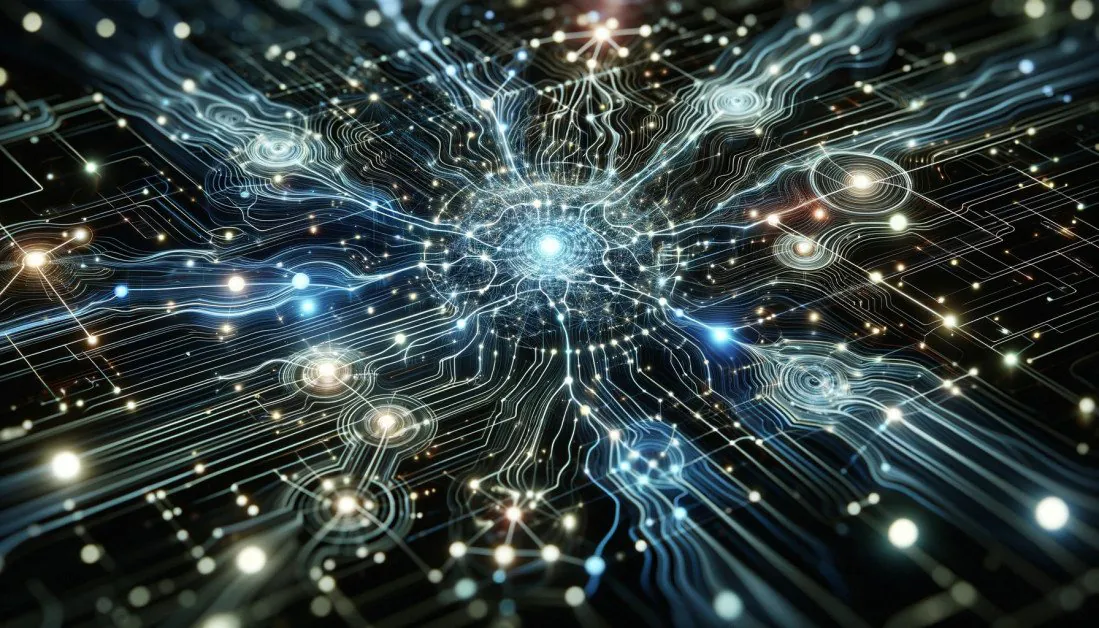
In the rapidly evolving landscape of AI, the promise of transformative changes spans across a myriad of fields, from the revolutionary prospects of autonomous vehicles reshaping transportation to the sophisticated use of AI in interpreting complex medical images. The advancement of AI technologies has been nothing short of a digital renaissance, heralding a future brimming with possibilities and advancements.
However, a recent study sheds light on a concerning aspect that has been often overlooked: the increased vulnerability of AI systems to targeted adversarial attacks. This revelation calls into question the robustness of AI applications in critical areas and highlights the need for a deeper understanding of these vulnerabilities.
The Concept of Adversarial Attacks
Adversarial attacks in the realm of AI are a type of cyber threat where attackers deliberately manipulate the input data of an AI system to trick it into making incorrect decisions or classifications. These attacks exploit the inherent weaknesses in the way AI algorithms process and interpret data.
For instance, consider an autonomous vehicle relying on AI to recognize traffic signs. An adversarial attack could be as simple as placing a specially designed sticker on a stop sign, causing the AI to misinterpret it, potentially leading to disastrous consequences. Similarly, in the medical field, a hacker could subtly alter the data fed into an AI system analyzing X-ray images, leading to incorrect diagnoses. These examples underline the critical nature of these vulnerabilities, especially in applications where safety and human lives are at stake.
The Study's Alarming Findings
The study, co-authored by Tianfu Wu, an assoc. professor of electrical and computer engineering at North Carolina State University, delved into the prevalence of these adversarial vulnerabilities, uncovering that they are far more common than previously believed. This revelation is particularly concerning given the increasing integration of AI in critical and everyday technologies.
Wu highlights the gravity of this situation, stating, “Attackers can take advantage of these vulnerabilities to force the AI to interpret the data to be whatever they want. This is incredibly important because if an AI system is not robust against these sorts of attacks, you don't want to put the system into practical use — particularly for applications that can affect human lives.”
QuadAttacK: A Tool for Unmasking Vulnerabilities
In response to these findings, Wu and his team developed QuadAttacK, a pioneering piece of software designed to systematically test deep neural networks for adversarial vulnerabilities. QuadAttacK operates by observing an AI system's response to clean data and learning how it makes decisions. It then manipulates the data to test the AI's vulnerability.
Wu elucidates, “QuadAttacK watches these operations and learns how the AI is making decisions related to the data. This allows QuadAttacK to determine how the data could be manipulated to fool the AI.”
In proof-of-concept testing, QuadAttacK was used to evaluate four widely used neural networks. The results were startling.
“We were surprised to find that all four of these networks were very vulnerable to adversarial attacks,” says Wu, highlighting a critical issue in the field of AI.
These findings serve as a wake-up call to the AI research community and industries reliant on AI technologies. The vulnerabilities uncovered not only pose risks to the current applications but also cast doubt on the future deployment of AI systems in sensitive areas.
A Call to Action for the AI Community
The public availability of QuadAttacK marks a significant step toward broader research and development efforts in securing AI systems. By making this tool accessible, Wu and his team have provided a valuable resource for researchers and developers to identify and address vulnerabilities in their AI systems.
The research team’s findings and the QuadAttacK tool are being presented at the Conference on Neural Information Processing Systems (NeurIPS 2023). The primary author of the paper is Thomas Paniagua, a Ph.D. student at NC State, alongside co-author Ryan Grainger, also a Ph.D. student at the university. This presentation is not just an academic exercise but a call to action for the global AI community to prioritize security in AI development.
As we stand at the crossroads of AI innovation and security, the work of Wu and his collaborators offers both a cautionary tale and a roadmap for a future where AI can be both powerful and secure. The journey ahead is complex but essential for the sustainable integration of AI into the fabric of our digital society.
The team has made QuadAttacK publicly available. You can find it here: https://thomaspaniagua.github.io/quadattack_web/
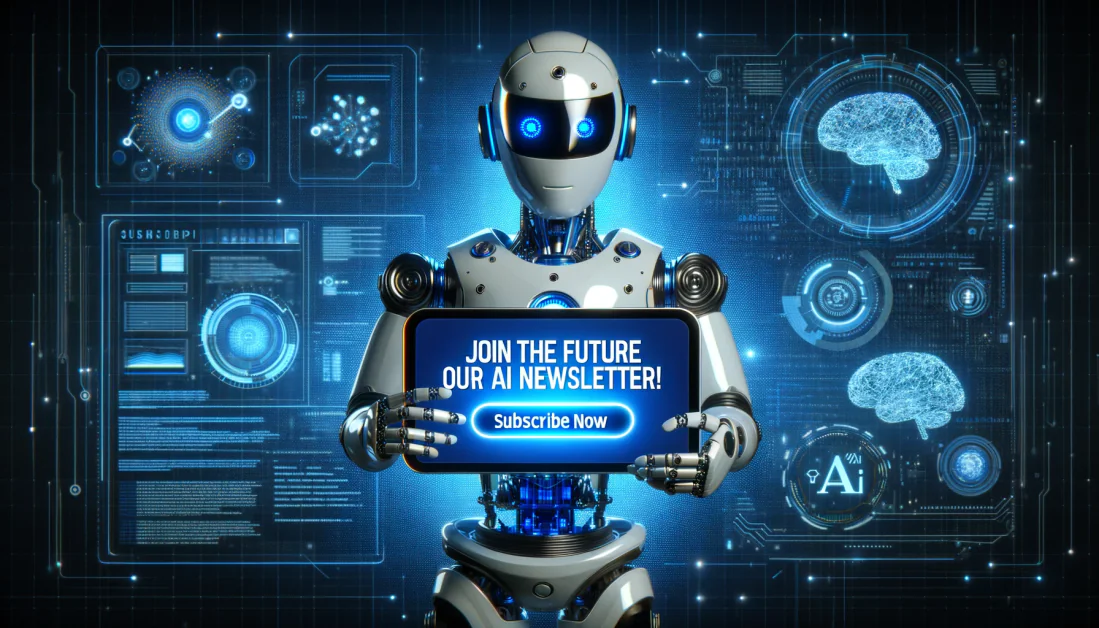
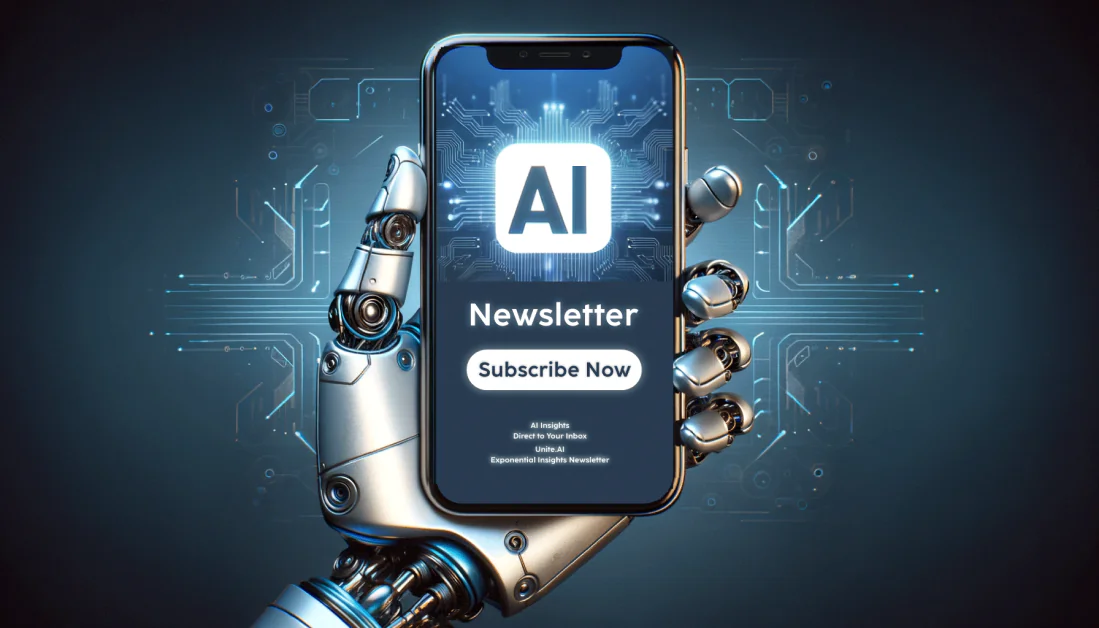