Artificial Intelligence
DeepMind Poised To Transform Biological Sciences By Solving Protein Folding Problem
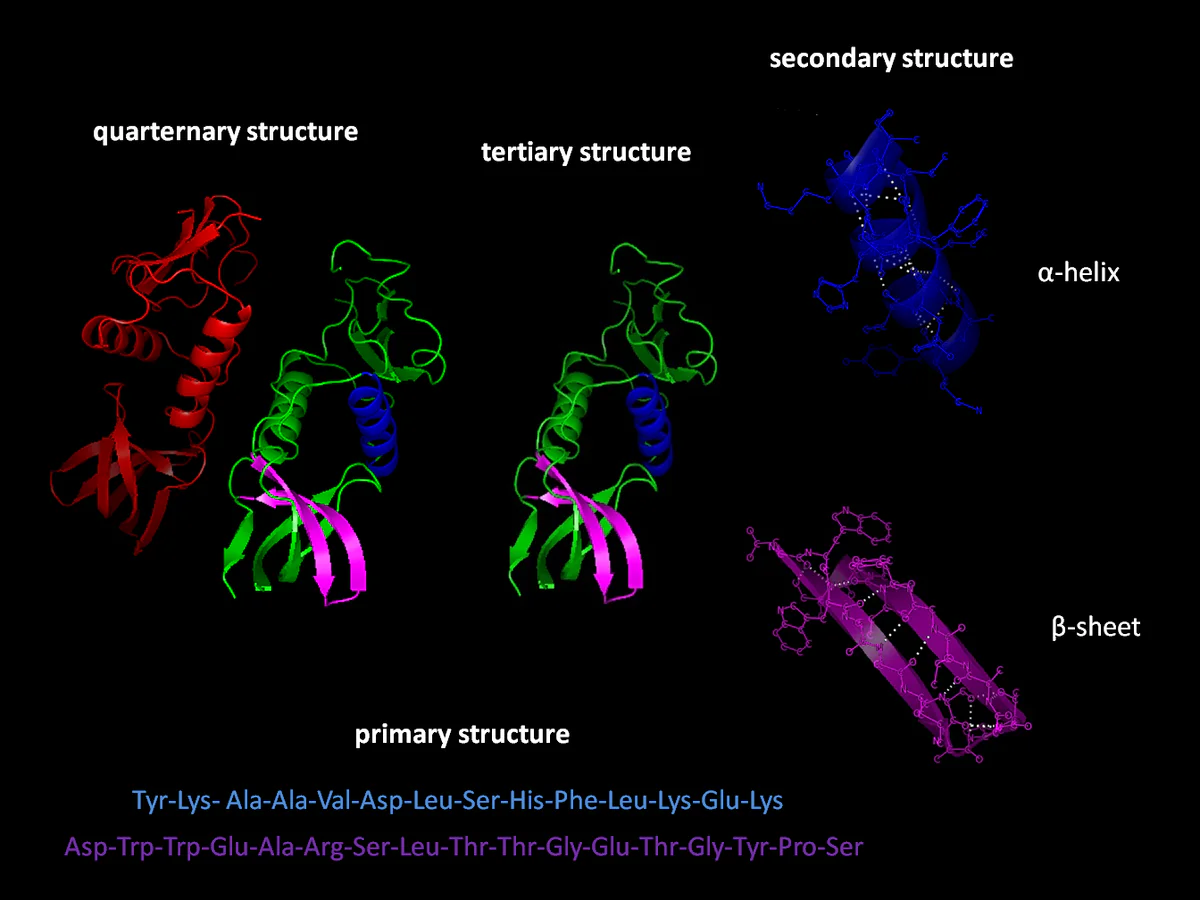
Google’s AI division DeepMind has recently made significant progress towards solving one of the oldest challenges in biology, calculating the shape of a protein from an amino-acid sequence. According to Nature, the breakthrough has the potential to transform the fields of biology and chemistry, enabling scientists to determine the function of many proteins that are currently mysterious.
The shape of a protein defines its function, and most biological functions are dependent on proteins. “Protein folding” is the name given to the process that converts chains of amino acids into the three-dimensional structures protiones require to carry out their functions. If scientists can determine the relationship between amino acid sequences and the shape of the proteins that they generate, they can determine which proteins impact different biological processes.
Scientists hypothesize that there are at least 80,000 proteins within the human proteome, but only a small fraction of these proteins have known structures. The traditional method of determining the shape of a protein can take years of laboratory experiments, even leveraging the power of computer science algorithms and models. The work done by DeepMind can dramatically speed up the process of discovering protein structures, reliably determining the structure of proteins in a fraction of the normal time.
Researchers at DeepMind trained their algorithms on database comprised of approximately 170,0000 protein sequences and the shapes corresponding with those sequences. The algorithms developed by the researchers were trained on between 100 to 200 GPUs, and the training process took a few weeks to complete. The model developed the researchers was dubbed “AlphaFold”.
AlphaFold operates through a “tension algorithm”, beginning by connecting small pieces of the protein together and then scaling up to connect larger and larger sections. Small amino acid clusters were linked together at first, and then the algorithm sought to find ways of linking these clusters.
AlphaFold researchers initially tried using conventional deep learning algorithms on genetic and structural data to to predict the relationship between amino acids and proteins. AlphaFold then created consensus models for the style of the proteins. When this technique proved to have too many limitations, the researchers tried a new strategy. The AlphaFold research team created models trained on more features, and this time they had the model return predictions for the final structure of the protein sequences.
The engineering team stress tested AlphaFold by entering it in a competition where computer algorithms compete to assess the structure of a protein from amino acid sequences. The competition was the “Critical Assessment of Protein Structure Prediction” or CASP. Participants in the competition are provided with 100 amino acid sequences and their models must work out the structure of the proteins. Not only did AlphaFold beat out the other computer models in terms of accuracy, but it also performed comparably to the traditional, lab-based modeling techniques. AlphaFold’s final, median score was approximately 92 out of 100, with lab-based experimental methods being assigned a score of 90. AlphaFold’s median score fell to 87 percent on the most difficult proteins.
According to DeepMind Chief Executive and co-founder Demis Hassabis, the company is already making plans to give researchers access to AlphaFold, with scientists from the Max Planck Institute for Development Biology already utilizing the model to discover protein structures they had been working on for over a decade.
Janet Thornton, the European Bioinformatics Institute’s director emeritus, was quoted via ScienceMag as saying that DeepMind’s achievements “will change the future of structural biology and protein research”. Meanwhile, biologist at University of Maryland, Shady Grove, John Moult says that he never thought the protein-folding problem would never be solved in this lifetime.
While AlphaFold is highly unlikely to completely replace traditional, experimental methods of discovering protein structures, it could dramatically increase the speed at which protein structures are discovered. Researchers may require less high-quality experimental data to determine a protein structure, and researchers already have access to a large volume of genomic data that could be translated into structures using AlphaFold’s solutions.
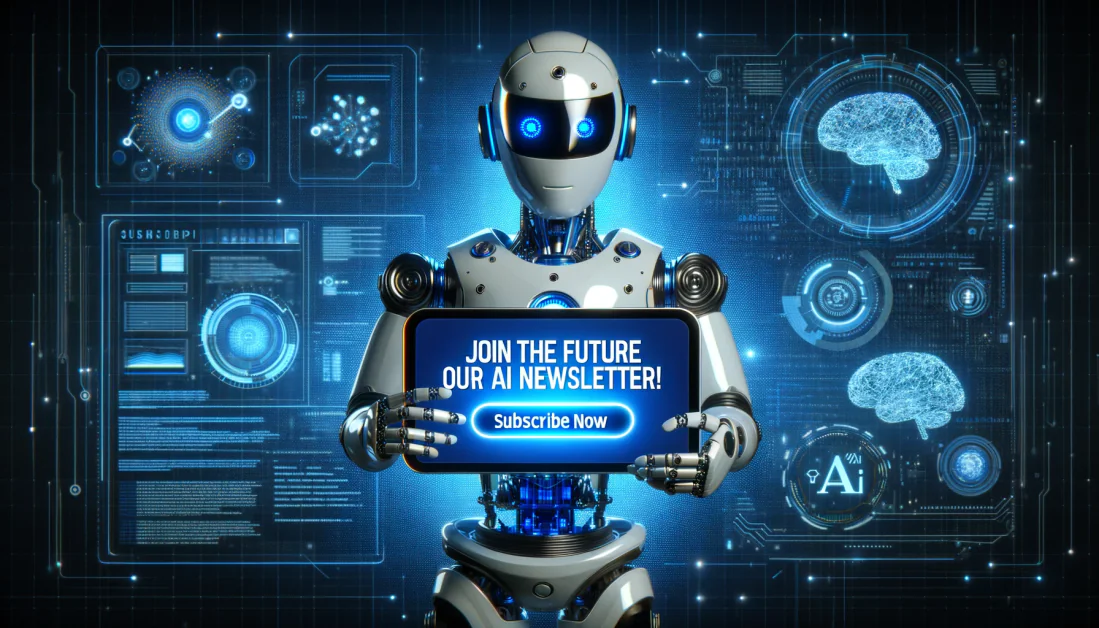
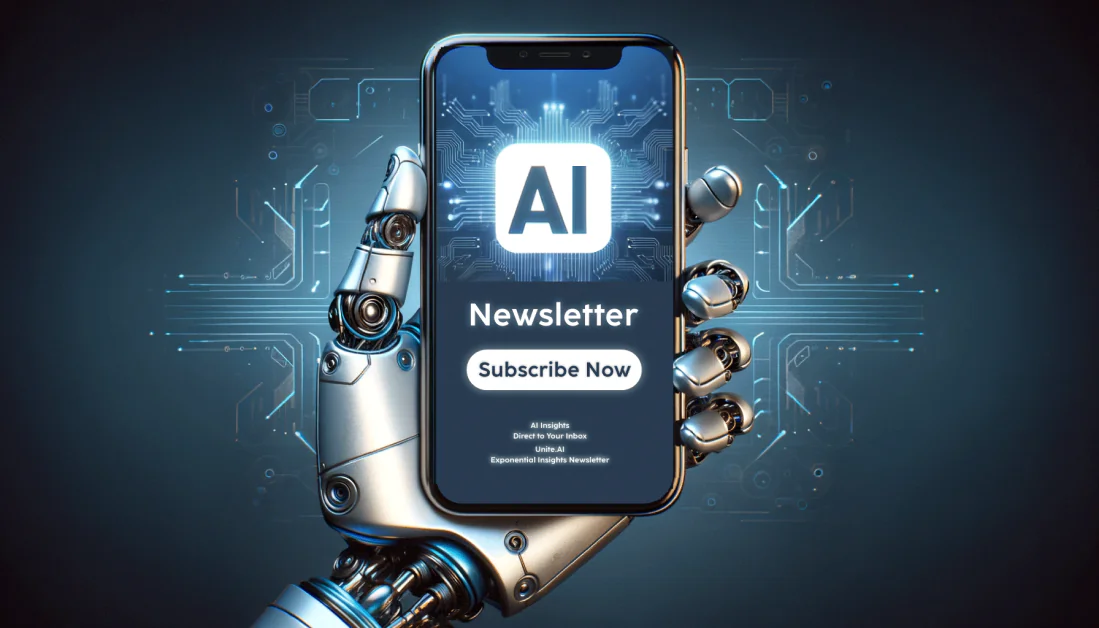