Artificial Intelligence
Researchers Use AI To Investigate How Reflections Differ From Original Images
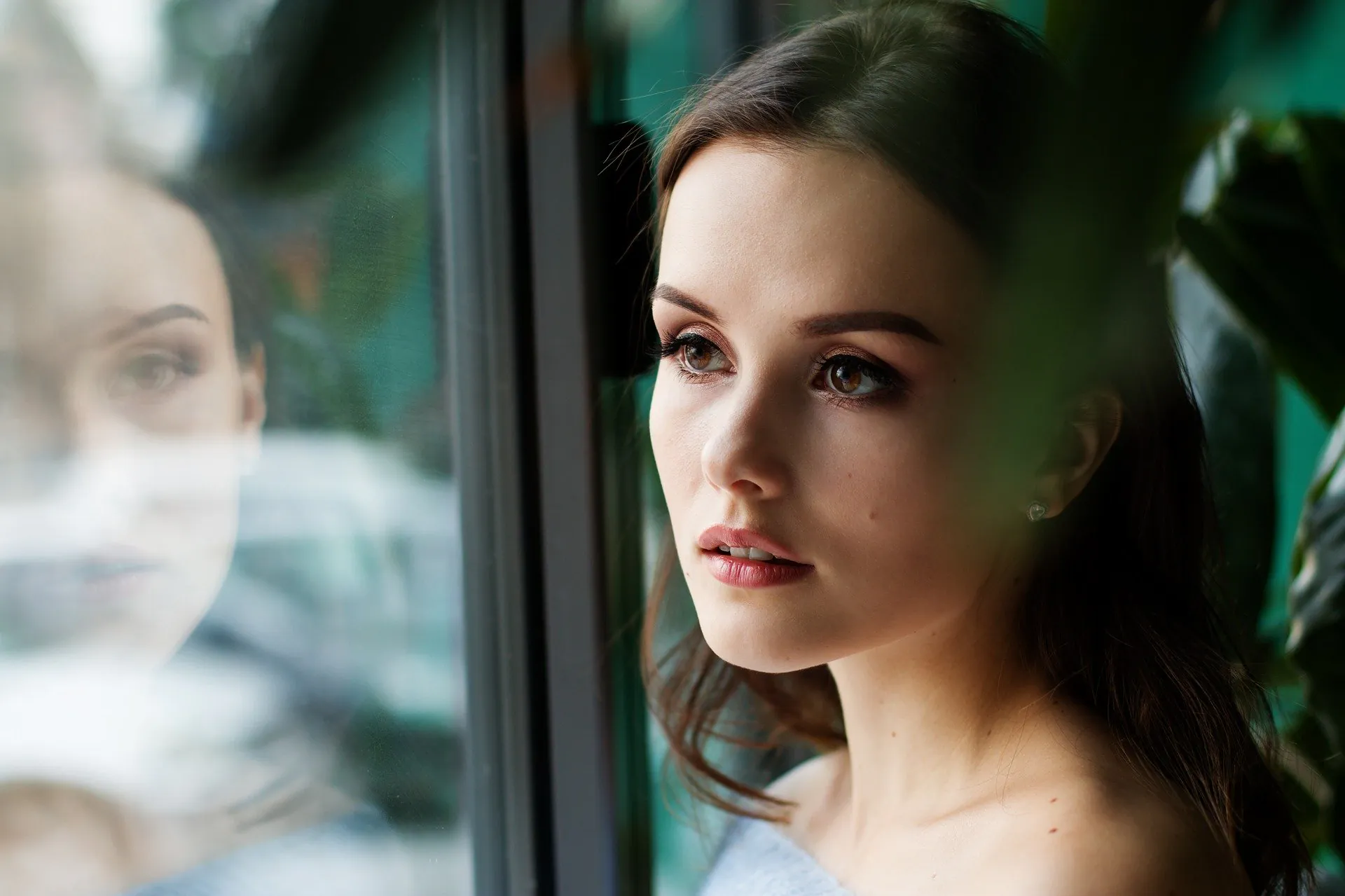
Researchers at Cornell University recently utilized machine learning systems to investigate how reflections of images are different from the original images. As reported by ScienceDaily, the algorithms created by the team of researchers found that there were telltale signs, differences from the original image, that an image had been flipped or reflected.
Associate professor of computer science at Cornell Tech, Noah Snavely, was the study’s senior author. According to Snavely, the research project started when the researchers became intrigued by how images were different in both obvious and subtle ways when they were reflected. Snavely explained that even things that appear very symmetrical at first glance can usually be distinguished as a reflection when studied. I'm intrigued by the discoveries you can make with new ways of gleaning information,” said Snavely, according to ScienceDaily.
The researchers focused on images of people, using them to train their algorithms. This was done because faces don’t seem obviously asymmetrical. When trained on data that distinguished flipped images from original images, the AI reportedly achieved an accuracy of between 60% to 90% across various types of images.
Many of the visual hallmarks of a flipped image the AI learned are quite subtle and difficult for humans to discern when they look at the flipped images. In order to better interpret the features that the AI was using to distinguish between flipped and original images, the researchers created a heatmap. The heatmap showed regions of the image that the AI tended to focus on. According to the researchers, one of the most common clues the AI used to distinguish flipped images was text. This was unsurprising, and the researchers removed images containing text from their training data in order to get a better idea of the more subtle differences between flipped and original images.
After images containing text were dropped from the training set, the researchers found that the AI classifier focused on features of the images like shirt callers, cell phones, wristwatches, and faces. Some of these features have obvious, reliable patterns that the AI can hone in on, such as the fact that people often carry cell phones in their right hand and that the buttons on shirt collars are often on the left. However, facial features are typically highly symmetrical with differences being small and very hard for a human observer to detect.
The researchers created another heatmap that highlighted the areas of faces that the AI tended to focus on. The AI often used people’s eyes, hair, and beards to detect flipped images. For reasons that are unclear, people often look slightly to the left when they have photos taken of them. As for why hair and beards are indicators of flipped images, the researchers are unsure but they theorize that a person’s handedness could be revealed by the way they shave or comb. While these indicators can be unreliable, by combining multiple indicators together the researchers can achieve greater confidence and accuracy.
More research along these lines will need to be carried out, but if the findings are consistent and reliable then it could help researchers find more efficient ways of training machine learning algorithms. Computer vision AI is often trained using reflections of images, as it is an effective and quick way of increasing the amount of available training data. It’s possible that analyzing how the reflected images are different could help machine learning researchers gain a better understanding of the biases present in machine learning models that might cause them to inaccurately classify images.
As Snavely was quoted by ScienceDaily:
“This leads to an open question for the computer vision community, which is, when is it OK to do this flipping to augment your dataset, and when is it not OK? I'm hoping this will get people to think more about these questions and start to develop tools to understand how it's biasing the algorithm.”
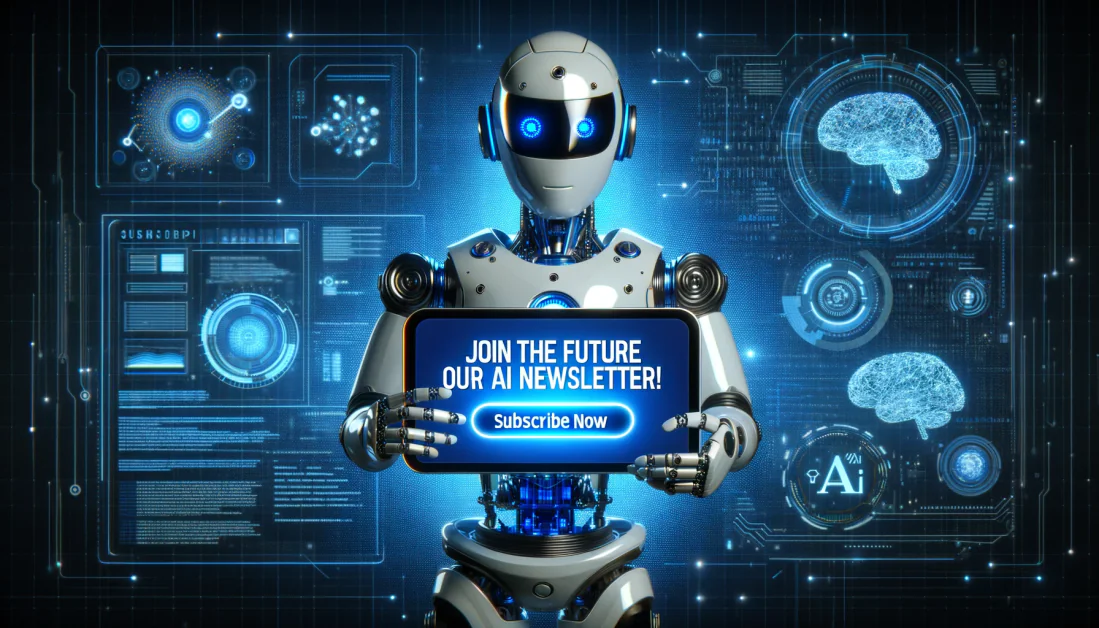
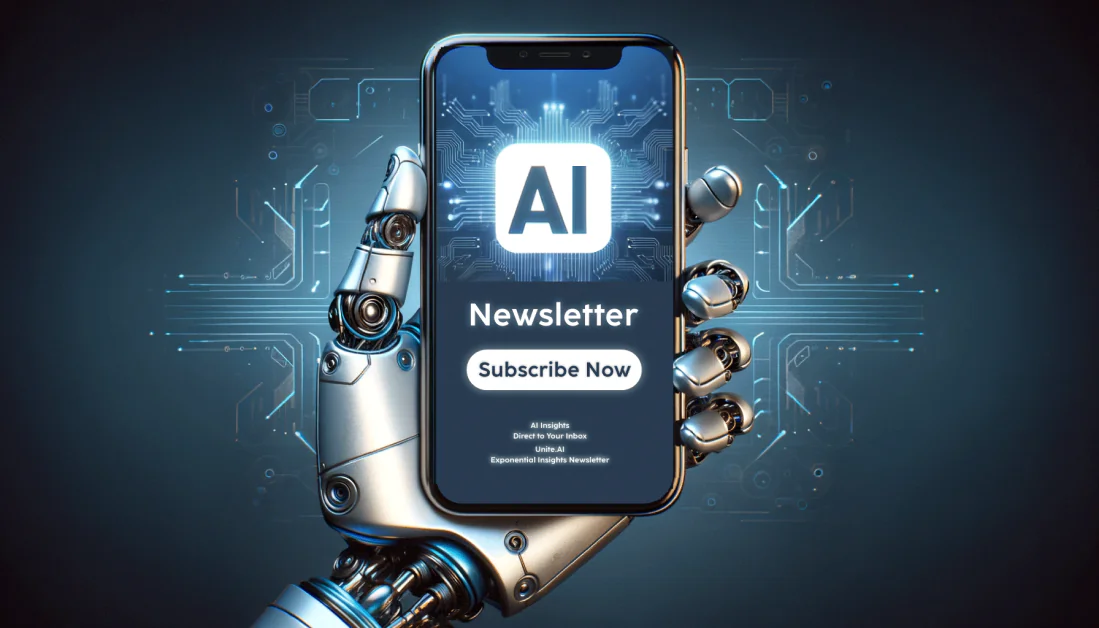