Artificial Intelligence
Safety of Self-Driving Cars Improved With New Training Method
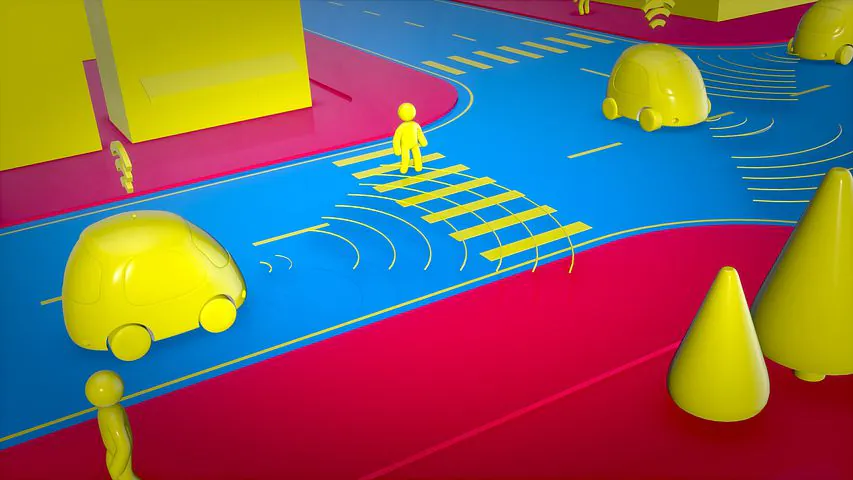
One of the most important tasks for a self-driving car when it comes to safety is tracking pedestrians, objects, and other vehicles or bicycles. In order to do this, self-driving cars rely on tracking systems. These systems could become even more effective with a new method developed by researchers at Carnegie Mellon University (CMU).
The new method has unlocked a lot more autonomous driving data compared to before, such as road and traffic data that is crucial for training tracking systems. The more data there is, the more successful the self-driving car can be.
The work was presented at the virtual Computer Vision and Pattern Recognition (CVPR) conference between June 14-19.
Himangi Mittal is a research intern who works alongside David Held, an assistant professor in CMU’s Robotics Institute.
“Our method is much more robust than previous methods because we can train on much larger datasets,” Mittal said.
Lidar and Scene Flow
Most of today’s autonomous vehicles rely on lidar as their main system for navigation. Lidar is a laser device that looks at what is surrounding the vehicle and generates 3D information out of it.
The 3D information comes in the form of a cloud of points, and the vehicle uses a technique called scene flow in order to process the data. Scene flow involves the speed and trajectory of each 3D point being calculated. So, whenever there are other vehicles, pedestrians, or moving objects, they are portrayed to the system as a group of points moving together.
Traditional methods for training these systems usually require labeled datasets, which is sensor data that has been annotated to track the 3D points over time. Because these datasets are required to be manually labeled and are expensive, a very minimal amount exists. To get around this, simulated data is used in scene flow training, and while it is less effective than the other way, a small amount of real-world data is used to improve it.
The named researchers, along with Ph.D. student Brian Okorn, developed the new method by using unlabeled data in scene flow training. This type of data is much easier to gather and only requires a lidar being placed on top of a car as it drives around.
Detecting Errors
In order for this to work, the researchers had to find a way for the system to detect its own errors in scene flow. The new system tries to make predictions about where each 3D point will end up and how fast it is traveling, and it then measures the distance between the predicted location and the actual location of the point. This is what forms one type of error to be minimized.
After that process, the system then reverses and works backward from the predicted point location to map where the point originated. By measuring the distance between the predicted position and the origin point, the second type of error is formed from the resulting distance.
After detecting these errors, the system then works to correct them.
“It turns out that to eliminate both of those errors, the system actually needs to learn to do the right thing, without ever being told what the right thing is,” Held said.
The results demonstrated scene flow accuracy at 25% when using a training set of synthetic data, and when it was improved with a small amount of real-world data, that number increased to 31%. The number improved even more to 46% when a large amount of unlabeled data was added to train the system.