Artificial Intelligence
Paraphrase Generation Using Deep Reinforcement Learning – Thought Leaders
When writing or talking we’ve all wondered whether there is a better way of communicating an idea to others. What words should I use? How should I structure the thought? How are they likely to respond? At Phrasee, we spend a lot of time thinking about language – what works and what doesn’t.
Imagine you are writing the subject line for an email campaign that will go to 10 million people in your list promoting 20% off a fancy new laptop.
Which line would you pick:
- You can now take an extra 20% off your next order
- Get ready – an extra 20% off
While they convey the same information, one achieved an almost 15% higher open rate than the other (and I bet you can’t beat our model at predicting which one ?). While language can often be tested through A/B testing or multi-armed bandits, automatically generating paraphrases remains a really challenging research problem.
Two sentences are considered paraphrases of one another if they share the same meaning and can be used interchangeably. Another important thing which is often taken for granted is whether a machine generated sentence is fluent.
Unlike supervised learning, Reinforcement Learning (RL) agents learn through interacting with their environment and observing the rewards they receive as a result. This somewhat nuanced difference has massive implications for how the algorithms work and how the models are trained. Deep Reinforcement Learning uses neural networks as a function approximator to allow the agent to learn how to outperform humans in complex environments such as Go, Atari, and StarCraft II.
Despite this success, reinforcement learning has not been widely applied to real-world problems including Natural Language Processing (NLP).
As part of my MSc thesis in Data Science, we demonstrate how Deep RL can be used to outperform supervised learning methods in automatically generating paraphrases of input text. The problem of generating the best paraphrase can be viewed as finding the series of words which maximizes the semantic similarity between sentences while maintaining fluency in the output. RL agents are well-suited for finding the best set of actions to achieve the maximum expected reward in control environments.
In contrast with most problems in machine learning, the largest problem in most Natural Language Generation (NLG) applications does not lie in the modelling but rather in the evaluation. While human evaluation is currently considered the gold standard in NLG evaluation, it suffers from significant disadvantages including being expensive, time-consuming, challenging to tune, and lacking reproducibility across experiments and datasets (Han, 2016). As a result, researchers have long been searching for automatic metrics which are simple, generalizable, and which reflect human judgment (Papineni et al., 2002).
The most common automatic evaluation methods in evaluating machine generated image captions are summarized below with their pros and cons:
Paraphrase Generation using Reinforcement Learning Pipeline
We developed a system named ParaPhrasee which generates high quality paraphrases. The system consists of multiple steps in order to apply reinforcement learning in a computationally efficient way. A brief summary of the high-level pipeline is shown below with more detail contained in the thesis.
Dataset
There are several paraphrase datasets available that are used in research including: the Microsoft Paraphrase corpus, ACL's Semantic Text Similarity competition, Quora Duplicate Questions, and Twitter Shared Links. We have selected MS-COCO given its size, cleanliness, and use as a benchmark for two notable paraphrase generation papers. MS-COCO contains 120k images of common scenes with 5 image captions per image provided by 5 different human annotators.
While it is primarily designed for computer vision research, the captions tend to have high semantic similarity and are interesting paraphrases. Given the image captions are provided by different people they tend to have slight variations in detail provided in the scene therefore the generated sentences tend to hallucinate details.
Supervised Model
While reinforcement learning has improved considerably in terms of sample efficiency, training times, and overall best practices, training RL models from scratch is still comparatively very slow and unstable (Arulkumaran et al., 2017). Therefore, rather than train from scratch, we first train a supervised model and then fine-tune it using RL.
We use an Encoder-Decoder model framework and evaluate the performance of several baseline supervised models. When fine-tuning the model using RL, we only fine-tune the decoder network and treat the encoder network as static. As such we consider two main frameworks:
- Training the supervised model from scratch using a standard/vanilla encoder decoder with GRUs
- Using pretrained sentence embedding models for the encoder including: pooled word embeddings (GloVe), InferSent, and BERT
The supervised models tend to perform fairly similarly across models with BERT and the vanilla encoder-decoder achieving the best performance.
While the performance tends to be reasonable, there are three common sources of error: stuttering, generating sentence fragments, and hallucinations. These are the main problems that using RL aims to solve.
Reinforcement Learning Model
Implementing RL algorithms is very challenging especially when you don’t know if the problem can be solved. There can be problems in the implementation of your environment, your agents, your hyperparameters, your reward function, or a combination of all of the above! These problems are exacerbated when doing deep RL as you get the fun of the added complexity of debugging neural networks.
As with all debugging, it is crucial to start simple. We implemented variations of two well understood toy RL environments (CartPole and FrozenLake) to test RL algorithms and find a repeatable strategy for transferring knowledge from the supervised model.
We found that using an Actor-Critic algorithm outperformed REINFORCE in these environments. In terms of transferring knowledge to the actor-critic model, we found that initializing the actor’s weights with the trained supervised model and pretraining the critic achieved the best performance. We found it challenging to generalize sophisticated policy distillation approaches to new environments as they introduce many new hyperparameters which require tuning to work.
Supported by these insights, we then turn to developing an approach for the paraphrase generation task. We first need to create an environment.
The environment allows us to easily test the impact of using different evaluation metrics as reward functions.
We then define the agent, given its many advantages we use an actor-critic architecture. The actor is used to select the next word in the sequence and has its weights initialized using the supervised model. The critic provides an estimate of the expected reward a state is likely to receive to help the actor learn.
Designing the Right Reward Function
The most important component of designing a RL system is the reward function as this is what the RL agent is trying to optimize. If the reward function is incorrect, then the results will suffer even if every other part of the system works!
A classic example of this is CoastRunners where the OpenAI researchers set the reward function as maximizing the total score rather than winning the race. The result of this is the agent discovered a loop where it could get the highest score by hitting turbos without ever completing the race.
Given evaluating the quality of paraphrases is itself an unsolved problem, designing a reward function that automatically captures this objective is even harder. Most aspects of language do not decompose nicely into linear metrics and are task dependent (Novikova et al., 2017).
The RL agent often discovers an interesting strategy to maximize rewards which exploits the weaknesses in the evaluation metric rather than generating high quality text. This tends to result in poor performance on metrics which the agent is not directly optimizing.
We consider three main approaches:
- Word-overlap Metrics
Common NLP evaluation metrics consider the proportion of word overlap between the generated paraphrase and the evaluation sentence. The greater the overlap the greater the reward. The challenge with word-level approaches is the agent includes too many connecting words such as “a is on of” and there is no measure of fluency. This results in very low-quality paraphrases.
- Sentence-level Similarity and Fluency Metrics
The main properties of a generated paraphrase are that it must be fluent and semantically similar to the input sentence. Therefore, we try to explicitly score these individually then combine the metrics. For semantic similarity, we use the cosine similarity between sentence embeddings from pretrained models including BERT. For fluency, we use a score based on the perplexity of a sentence from GPT-2. The greater the cosine similarity and fluency scores the greater the reward.
We tried many different combinations of sentence embedding models and fluency models and while the performance was reasonable, the main issue the agent faced was not sufficiently balancing semantic similarity with fluency. For most configurations, the agent prioritized fluency resulting in removing detail and most entities being placed “in the middle” of something or being moved “on a table” or “side of the road”.
Multi-objective reinforcement learning is an open research question and is very challenging in this case.
- Using an Adversarial Model as a Reward Function
Given humans are considered the gold standard in evaluation, we train a separate model called the discriminator to predict whether or not two sentences are paraphrases of one another (similar to the way a human would evaluate). The goal of the RL model is then to convince this model that the generated sentence is a paraphrase of the input. The discriminator generates a score of how likely the two sentences are to be paraphrases of one another which is used as the reward to train the agent.
Every 5,000 guesses the discriminator is told which paraphrase came from the dataset and which was generated so it can improve its future guesses. The process continues for several rounds with the agent trying to fool the discriminator and the discriminator trying to differentiate between the generated paraphrases and the evaluation paraphrases from the dataset.
After several rounds of training, the agent generates paraphrases that outperform the supervised models and other reward functions.
Conclusion and Limitations
Adversarial approaches (including self-play for games) provide an extremely promising approach for training RL algorithms to exceed human level performance on certain tasks without defining an explicit reward function.
While RL was able to outperform supervised learning in this instance, the amount of extra overhead in terms of code, computation, and complexity is not worth the performance gain for most applications. RL is best left to situations where supervised learning cannot be easily applied, and a reward function is easy to define (such as Atari games). The approaches and algorithms are far more mature in supervised learning and the error signal is much stronger which results in much faster and more stable training.
Another consideration is, as with other neural approaches, that the agent can fail very dramatically in cases where the input is different from the inputs it has previously seen, requiring an additional layer of sanity checks for production applications.
The explosion of interest in RL approaches and advances in computational infrastructure in the last few years will unlock huge opportunities for applying RL in industry, especially within NLP.
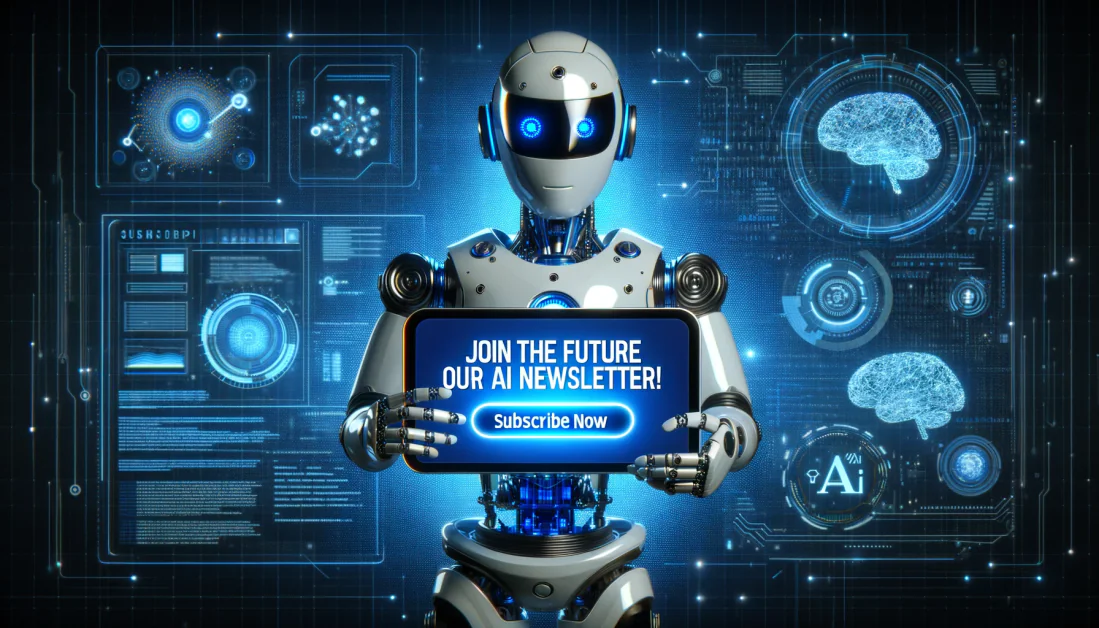
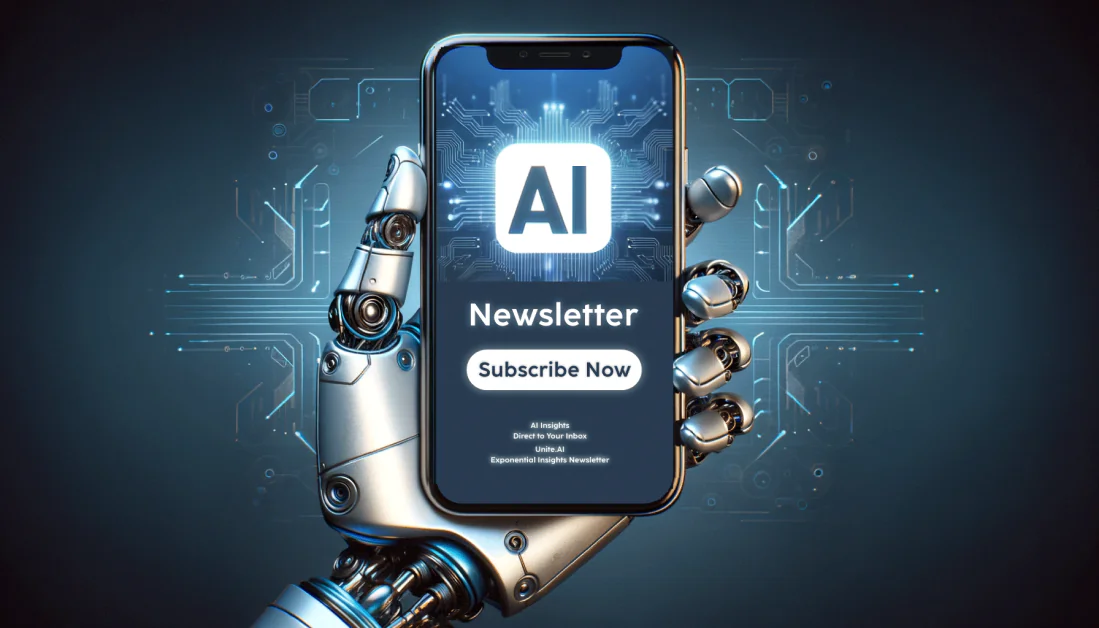