Ethics
Researchers Believe AI Can Be Used To Help Protect People’s Privacy
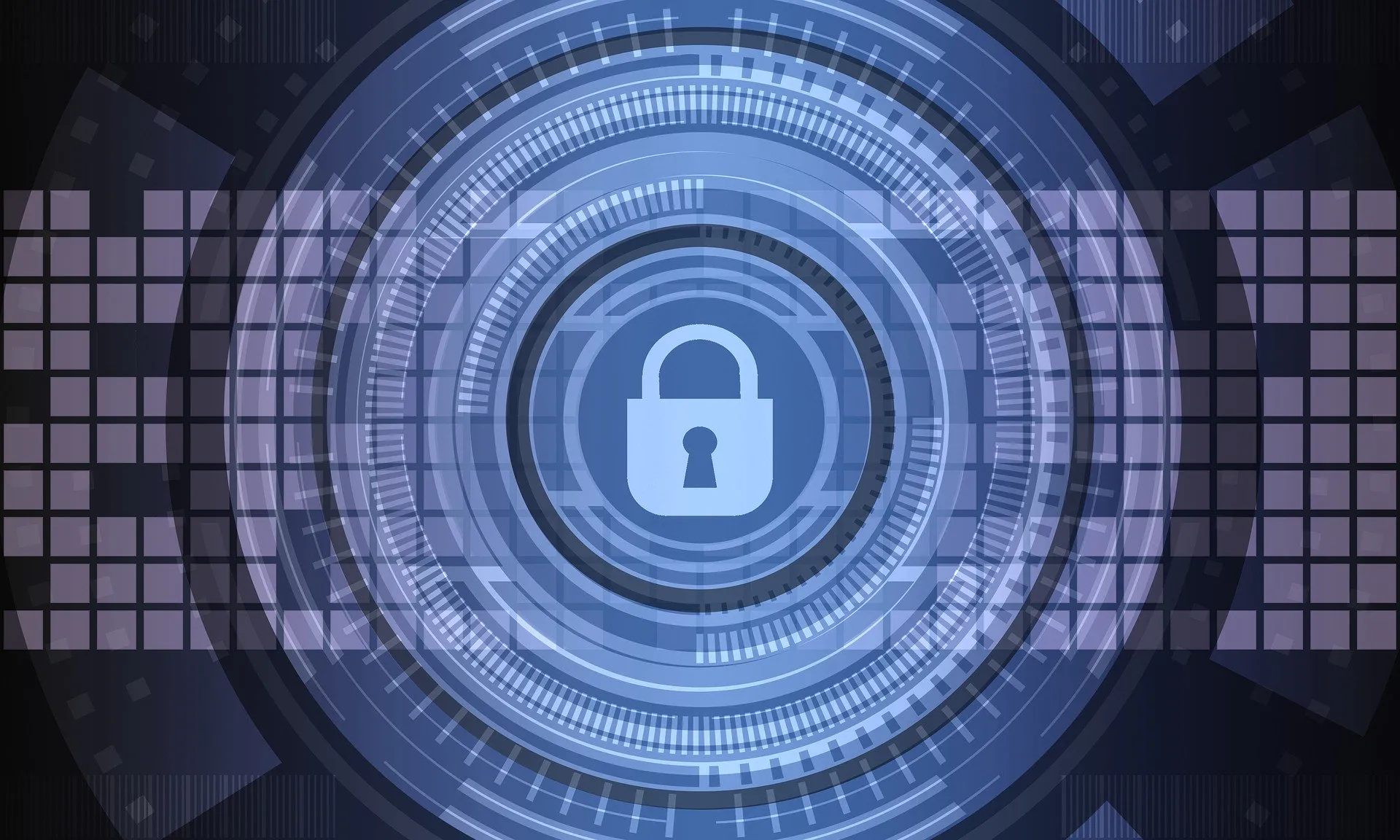
Two professors of information science have recently published a piece in The Conversation, arguing that AI could help preserve peopleās privacy, rectifying some of the issues that it has created.
Zhiyuan Chen and Aryya Gangopadhyay argue that artificial intelligence algorithms could be used to defend peopleās privacy, counteracting some of the many privacy concerns other uses of AI have created. Chen and Gangopadhyay acknowledge that many of the AI-driven products we use for convenience wouldnāt work without access to large amounts of data, which at first glance seems at odds with attempts to preserve privacy. Furthermore, as AI spreads out into more and more industries and applications, more data will be collected and stored in databases, making breaches of those databases tempting. However, Chen and Gangopadhyay believe that when used correctly, AI can help mitigate these issues.
Chen and Gangopadhyay explain in their post that the privacy risks associated with AI come from at least two different sources. The first source is the large datasets collected to train neural network models, while the second privacy threat is the models themselves. Data can potentially āleakā from these models, with the behavior of the models giving away details about the data used to train them.
Deep neural networks are comprised of multiple layers of neurons, with each layer connected to the layers around them. The individual neurons, as well as the links between neurons,Ā encode for different bits of the training data. The model may prove to be too good as remembering patterns of the training data, even if the model isnāt overfitting. Traces of the training data exist within the network and malicious actors may be able to ascertain aspects of the training data, as Cornell University found during one of their studies. Cornell researchers found that facial recognition algorithms could be exploited by attackers to reveal which images, and therefore which people, were used to train the face recognition model. The Cornell researchers discovered that even if an attacker doesnāt have access to the original model used to train the application, the attacker may still be able to probe the network and determine if a specific person was included in the training data simply by using models was that were trained on highly similar data.
Some AI models are currently being used to protect against data breaches and try to ensure peopleās privacy. AI models are frequently used to detect hacking attempts by recognizing the patterns of behavior that hackers use to penetrate security methods. However, hackers often change up their behavior to try and fool pattern-detecting AI.
New methods of AI training and development aim to make AI models and applications less vulnerable to hacking methods and security evasion tactics. Adversarial learning endeavors to train AI models on simulations of malicious or harmful inputs and in doing so make the model more robust to exploitation, hence the āadversarialā in the name. According to Chen and Gangopadhyay, their research has discovered methods of combatting malware designed to steal peopleās private info. The two researchers explained that one of the methods they found to be most effective at resisting malware was the introduction of uncertainty into the model. The goal is to make it more difficult for bad actors to anticipate how the model will react to any given input.
Other methods of utilizing AI to protect privacy include minimizing data exposure when the model is created and trained, as well as probing to discover the networkās vulnerabilities. When it comes to preserving data privacy, federated learning can help protect the privacy of sensitive data, as it allows a model to be trained by without the training data ever leaving the local devices that contain the data, insulating the data, and much of the modelās parameters from spying.
Ultimately, Chen and Gangopadhyay argue that while the proliferation of AI has created new threats to peopleās privacy, AI can also help protect privacy when designed with care and consideration.