Artificial Intelligence
Pixelmator Brings AI-Driven Super Resolution To Its Users
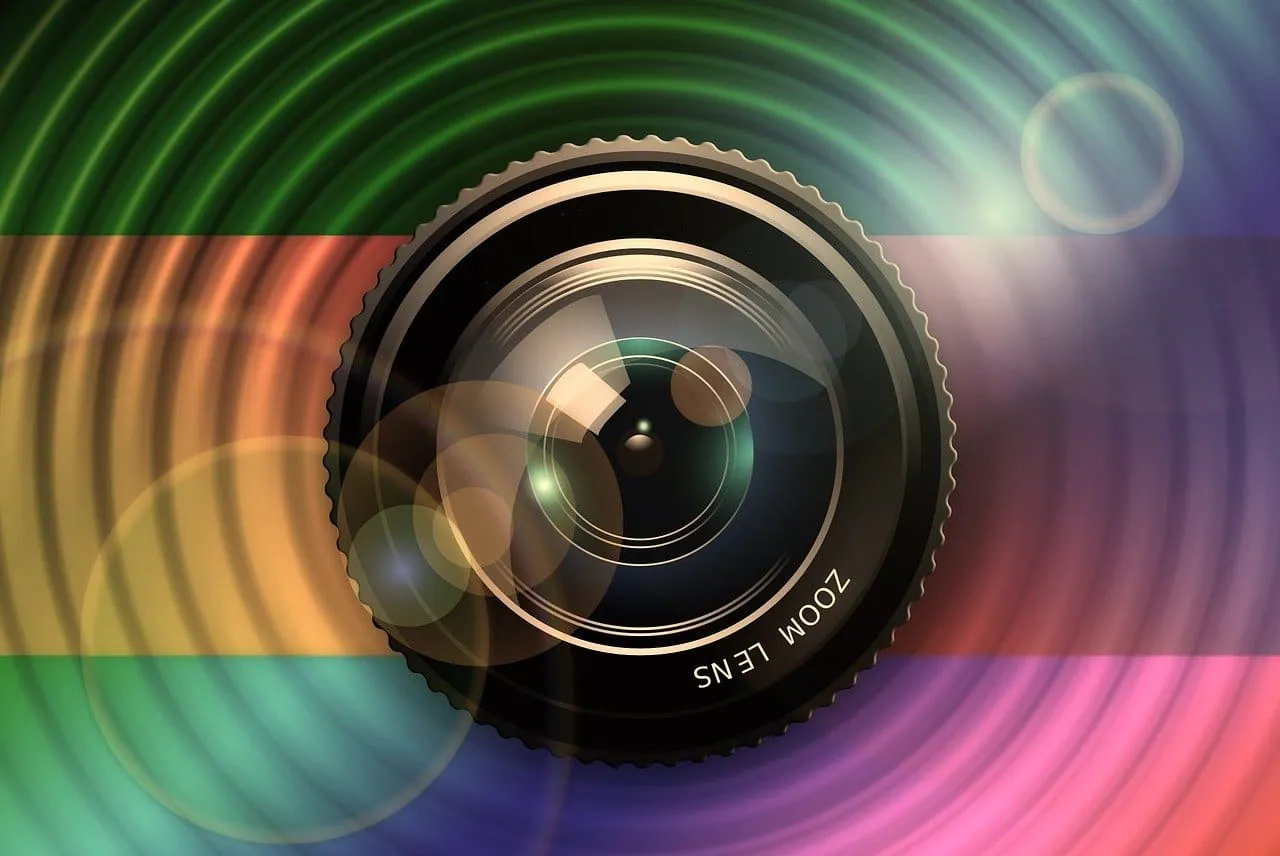
Pixelmator has recently enabled owners of Pixelmator Pro, a photo manipulation app, to make use of a super-resolution tool powered by AI.
Super-resolution enables blurry, low-resolution photos to be enhanced and the resolution of the image improved. Super-resolution technology is able to sharpen images to impressive effect, often evoking the “enhancing” trope that is often seen in crime shows. Pixelmator recently announced the inclusion of its “ML Super Resolution” tool in the Pro version of its photo editing software. Pixelmator's demonstration of some of the results can be seen here.
Early tests of the tool show that it is able to reduce blur in multiple types of images, including text, photographs, and illustration. As reported by The Verge, the results created by the program also seem to be better than other image upscaling tools, which often utilize algorithms such as Nearest Neighbors and Bilinear algorithms.
Research conducted into super-resolution has been driven by a variety of tech companies like Google, Microsoft, and Nvidia. Multiple companies have designed their own super-resolution algorithms, but the method used to train the difference super-resolution devices uses the same basic principles.
ML Super Resolution, and other super-resolution tools, are trained using pairs of low-resolution and high-resolution images. The low-resolution images are typically just scaled-down versions of the regular, high-resolution images. Comparisons are made between the low-resolution and high-resolution images, and the machine learning algorithms learn how the regions of pixels in the high-resolution images are different from the low-resolution images. The goal is that the neural networks learn to distinguish patterns of pixels that will lead to a higher resolution image. It can then use these patterns of difference to predict where to add pixels to an image to improve resolution when it is presented with an unseen image.
Super-resolution applications can be created with a variety of methods. For instance, one method of super-resolution is the use of Generative Adversarial Networks (GANs). GANs are actually two neural networks pitted against one another, borrowing concepts from Game Theory like the zero-sum game and the actor-critic model. Essentially, one neural network’s job is to create fake images, while the job of the other network is to detect these fake images. The network that creates the fakes is called the generator, while the network that detects them is the discriminator.
In the case of Pixelmator’s Super-Resolution tool, a convolutional neural network was created that also implemented an “enlarge” block which upscales the image after the 29 convolutional layers scan the image. The enlarged image array is then post-processed and transformed back into a traditional image with improved resolution. The network also contains functions that denoise the image and deal with compression artifacts, so that these aspects of the image are not upscaled. Pixelmator’s algorithms are much smaller than the algorithms used in research settings, in order that they can be included in the Pixelmator Pro app and run on a variety of devices. The training dataset is fairly small when compared to other datasets used for similar applications, just 15000 samples were used to train the algorithms.
According to The Verge, there are other super-resolution tools available for use by consumers. For instance, Adobe also has its own super-resolution tool in its Adobe Camera suite, but Pixelmator's tool seems to produce the most consistently high-quality images.
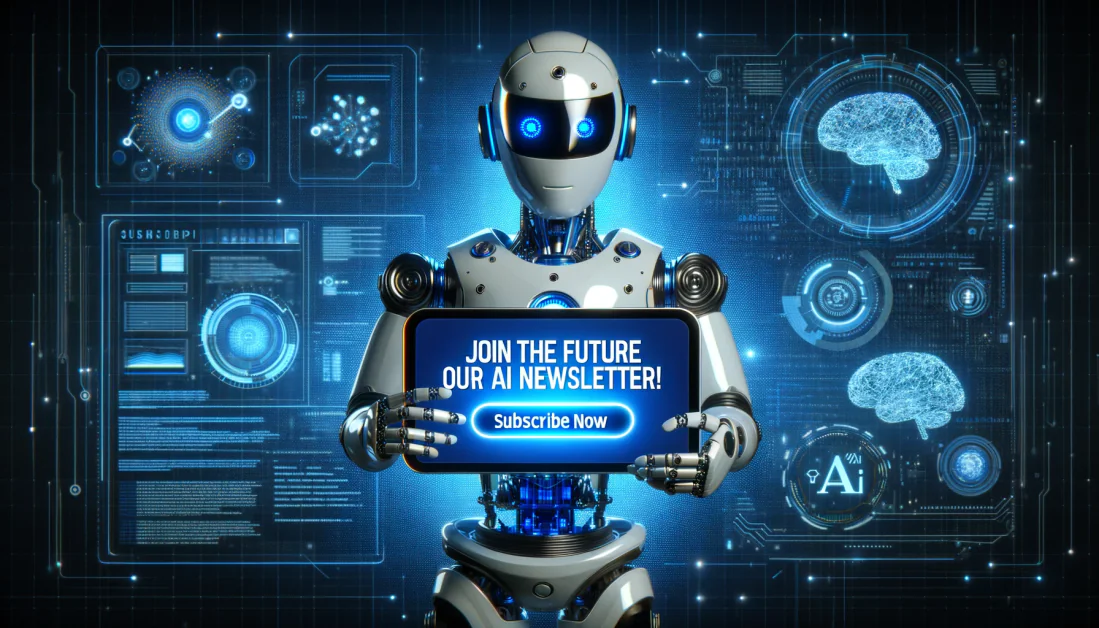
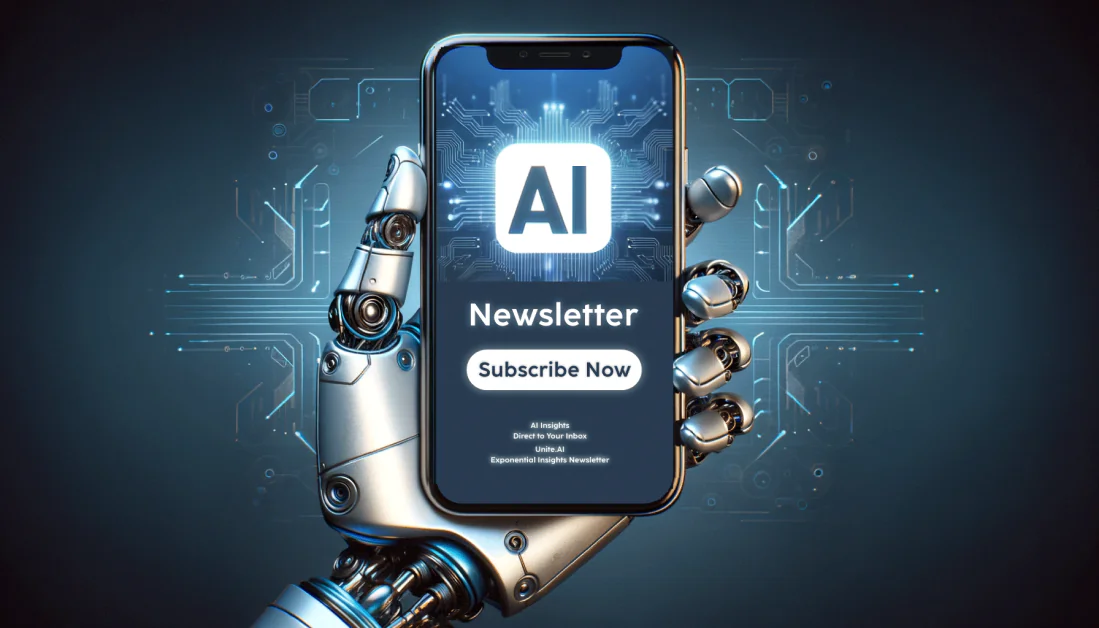