Robotics
A Mobile Recharging System for Power-Starved Autonomous Robots
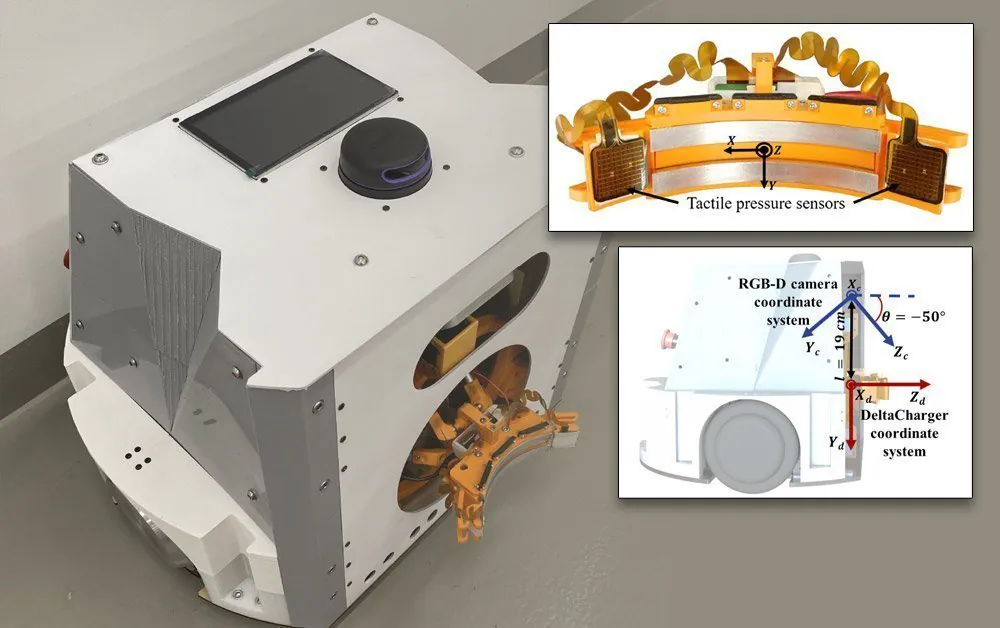
Russian researchers have developed a prototype robot capable of undertaking ad hoc recharging missions for agricultural and industrial robots whose autonomy is otherwise limited by lack of power outlets, and which also features a proposed standard for a charging plug/socket configuration applicable to mobile robotics systems.
The prototype for ‘MobileCharger', revealed in a recent paper from the Skolkovo Institute of Science and Technology at Moscow, is equipped with an engagement mechanism not dissimilar to the wide interlocking capabilities of military air transport systems designed for in-flight refueling.
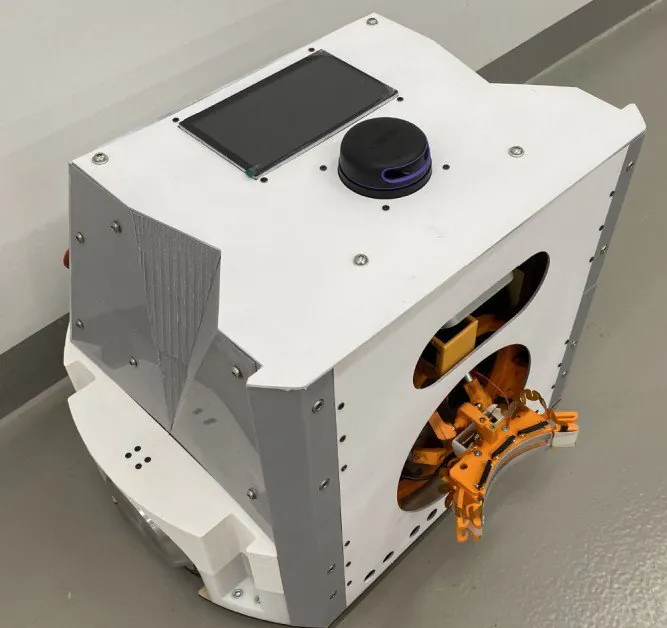
The MobileCharger prototype robot. Source: https://arxiv.org/pdf/2107.10585.pdf
MobileCharger attempts to improve on similar initiatives of recent years, such as Volkswagen's ‘Laderoboter' mobile charging robot and Samsung's EVAR Recharging Robot in several ways: by making a better trade-off between weight and capacity; by reducing the space requirements for recharging operations (a weakness of EVAR); by using Convolutional Neural Networks (CNNs) to optimize the engagement operation; and by using a range of sensors, including LiDAR and tactile sensors to estimate the relative position of the robot to be recharged.
The coupling system features a relative freedom of movement in three directions, in order to accommodate different designs of field robots, and to account for environments that may feature more uneven terrain than the flat surfaces of a factory floor.
Recharging operations are intended to last about four hours, with a consistent transfer of 22 volts. During the process, it's essential that the physical connection remain consistent, since horizontal or vertical misalignment is very likely to lead to damage of either or both robots, with the possibility of ungrounded electrical discharge.
The inverted delta actuator featured in MobileCharger offers a better operational speed than recent solutions to in-the-field recharging scenarios, with the researchers asserting that its compact and distinct design will be easier to manufacture.
The Delta Actuator also features magnet-based locking, though this would not be enough by itself to maintain safe contact during recharging operations, and is backed up by several complementary approaches, including a setup ring and an end effector with electrodes and servomotors.
Design-Agnostic Charging
MobileCharger is not designed to have complete domain knowledge of every robot that it might be required to recharge, but instead will use a range of methods to identify a compatible charging socket somewhere on the chassis of the robot, and maneuver itself into a proximity that allows it to connect to the target robot.
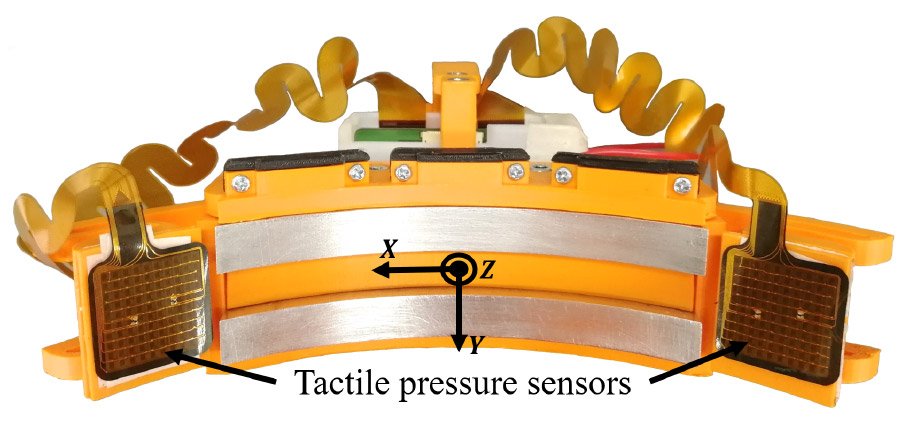
Tactile pressure sensor array in the docking mechanism of MobileCharger.
Effectively MobileCharger throws a gamut of solutions at the challenge of alignment evaluation and consistent engagement, including the RGB-D (depth-enabled) computer vision systems of fruit picking robots and pressure-sensitive tactile and haptic feedback systems of enclosed industrial robots.
Image Recognition
MobileCharger's LiDAR scanner is backed up by an Intel RealSense D435 RGB depth camera for proximity estimation, also serving as a feed mechanism for the robot's CNN-based contact estimation capabilities.
The object detection system used by MobileCharger is based around YOLOv3, an effective real-time image recognition system with 106 layers, powered by a custom-trained dataset centering on the proposed recharging dock design.
The dataset contains 170 samples, divided between 120 for training and 50 in test sets, made in a variety of lighting conditions, and a gamut of yaw variations from 75 to 105 degrees. Images in the training dataset are at 840×480 resolution, and feature RGB and depth data.
Convolutional Neural Network
The CNN used in MobileCharger addresses the issue of consistent contact during the charging operation, and is powered by two bespoke datasets: the first dataset features data on angular misalignment between electrodes, with 600 data pairs derived from tactile sensor mechanisms; the second dataset deals with horizontal and vertical misalignment of the end effector (the charging assembly), and contains 500 data pairs.
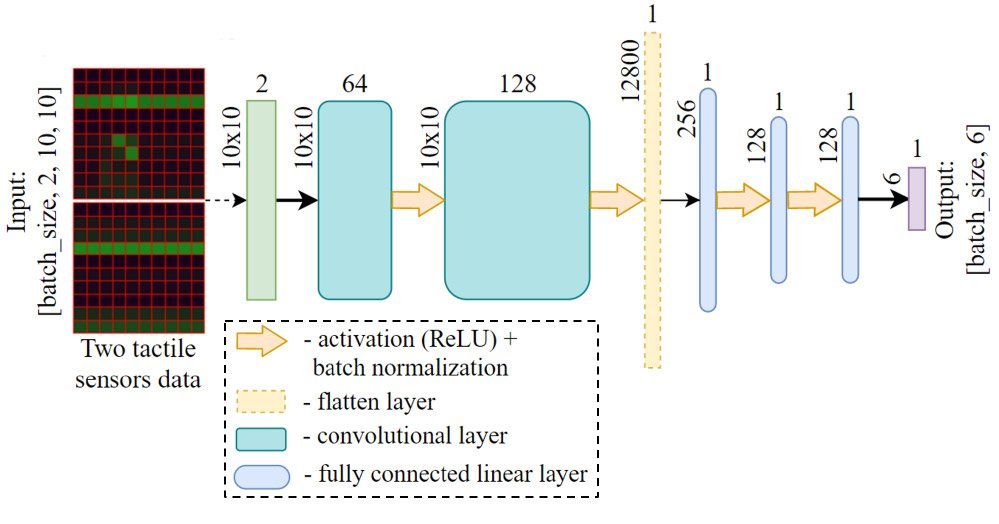
The CNN architecture for grasp consistency.
The data was split into 67% training and 33% evaluation sets, with accuracy as the target metric.
Prior to settling on the CNN/YOLO method, the researchers considered using Histogram of oriented gradients (HoG) with Support Vector Machine (SVM), and also contour detection with Canny edge detector. Neither method was considered adequately resilient to the challenges of in-the-field charging operations.
SwarmCharge
The MobileCharger system is intended for indoor and exterior environments, and future work by the researchers will address the development of localization, and of mapping algorithms. 1-Dof will also be explored as an additional facility for electrode rotation in the roll axis of the connection mechanism.
As one might expect, the proprietary coupling system is intended eventually for a wider ecostructure of robots and autonomous devices, which the paper says will be called SwarmCharge. MobileCharger is intended to be a support system for a wide variety of types of robot, including humanoid, quadruped and UAV mechanisms.