Artificial Intelligence
Neural Networks Used to Help Build 3D Map of the Universe
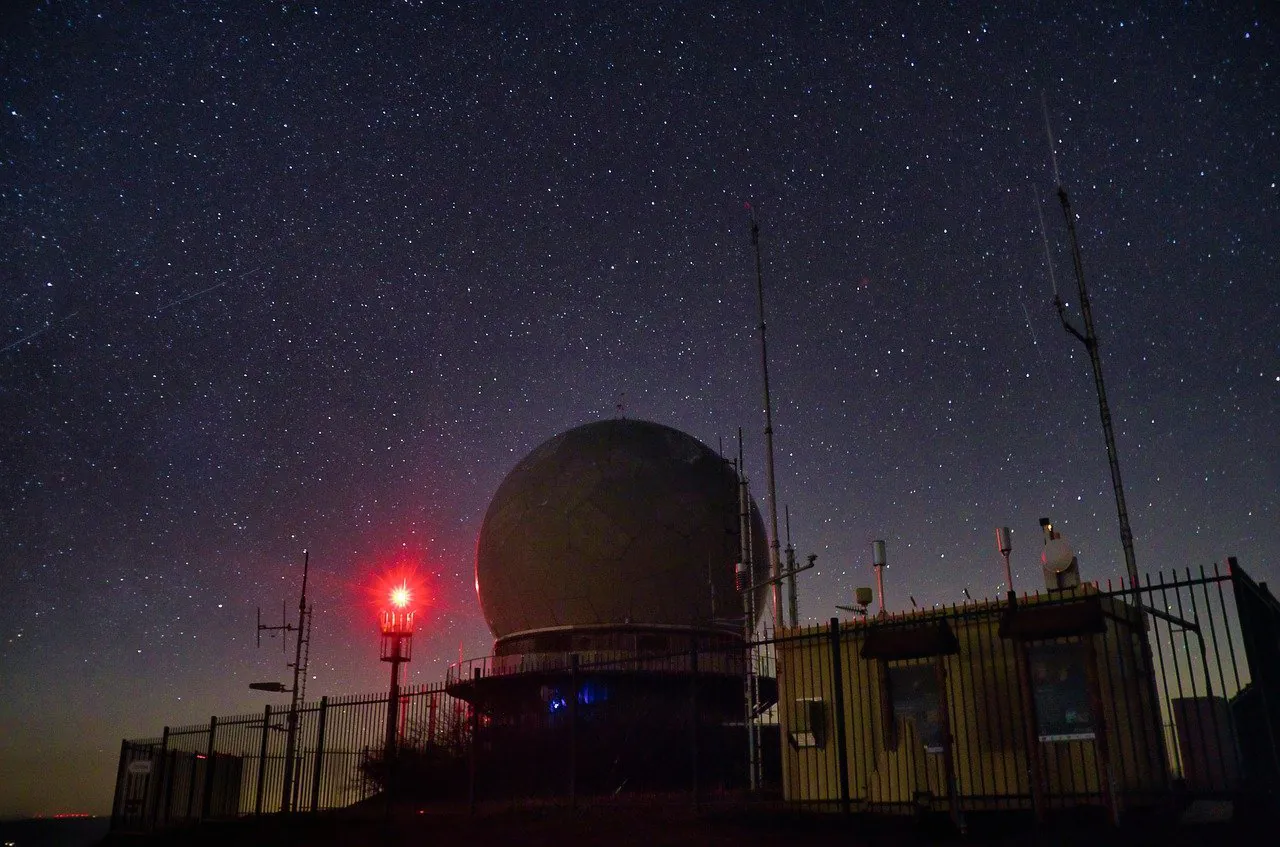
Astronomers from the University of Hawaii’s Astronomy department recently made use of AI algorithms to map build a massive 3D map of over 3 billion celestial objects. The astronomy team made use of spectroscopic data and neural network classification algorithms to accomplish the task.
Back in 2016, astronomers from the University of Hawaii at Manoa’s (UHM) Institute for Astronomy released to the public a massive dataset containing observational data for over 3 billion stars, galaxies, and other celestial objects, collected over 4 years of observing around three-quarters of the night sky. The project was called the Pan-STARRS project and the dataset it produced was approximately 2 petabytes (two million gigabytes) in size.
As Hans-Walter Rix, the director of the Galaxies and Cosmology department at the Max Plank Institutes for Astronomy explained according to Phys.org:
“Pan-STARRS1 mapped our home galaxy, the Milky Way, to a level of detail never achieved before. The survey provides, for the first time, a deep and global view of a significant fraction of the Milky Way plane and disk… Its unique combination of imaging depth, area and colors allowed it to discover the majority of the most distant known quasars: these are the earliest examples in our universe that giant black holes had grown at the centers of galaxies”.
One of the goals of releasing the dataset was that it would be used to build a map of the observable sky, classifying the many points of light that were observed in the dataset. Researchers involved with the Pan-STARRS project used the dataset to train machine learning algorithms they could use to generate the map.
The University of Hawaii researchers work with the PS1 telescope, located on Hawaii’s Big Island. The PS1 can scan approximately 75% of the observable sky. The telescope is the largest deep multicolor optical survey in the world, and the researchers wanted to leverage this power to build a sophisticated skymap. This involved training the PS1’s computers to classify objects, distinguishing one type of celestial body from another type. The dataset they used to train the computer contained millions of measurements, characterized by features like size and color.
The AI algorithms used were normal feedforward neural networks combined with optimization methods that allowed the networks to learn the complex relationships between the millions of data points. Robert Beck, former cosmology postdoc at the UHM’s Institute for Astronomy, explained that state of the art optimization algorithms was used to train the computer on the approximately 4 million celestial objects described by the dataset. As TechExplorist reported, the research team also had to correct for the interference of dust within the Milky Way galaxy. The research team used a Monte-Carlo sampling method to estimate the uncertainty created due to the photometric redshift (an estimation of the velocity of an object) and then trained the machine learning model on the spectroscopic data.
After the model was trained, its performance was checked on a validation dataset. The network successfully identified around 96.6% of quasars, 97.8% of stars, and 98.1% of galaxies. In addition, the model predicted the distance to galaxies and when checked the predictions were only off by approximately 3%.
The end result of the AI training and utilization was the largest 3D catalog of stars, quasars, and galaxies in the world. Co-author on the study Kenneth Chambers explained, as quoted by Gizmodo, that the models used to generate the map should be able to be used again as more and more data is collected, improving the map further and enhancing our understanding of our solar system and the universe. Scientists will be able to use the map to gain insights into the shape of the universe and determine where our cosmological model fails to line up with the new projections.
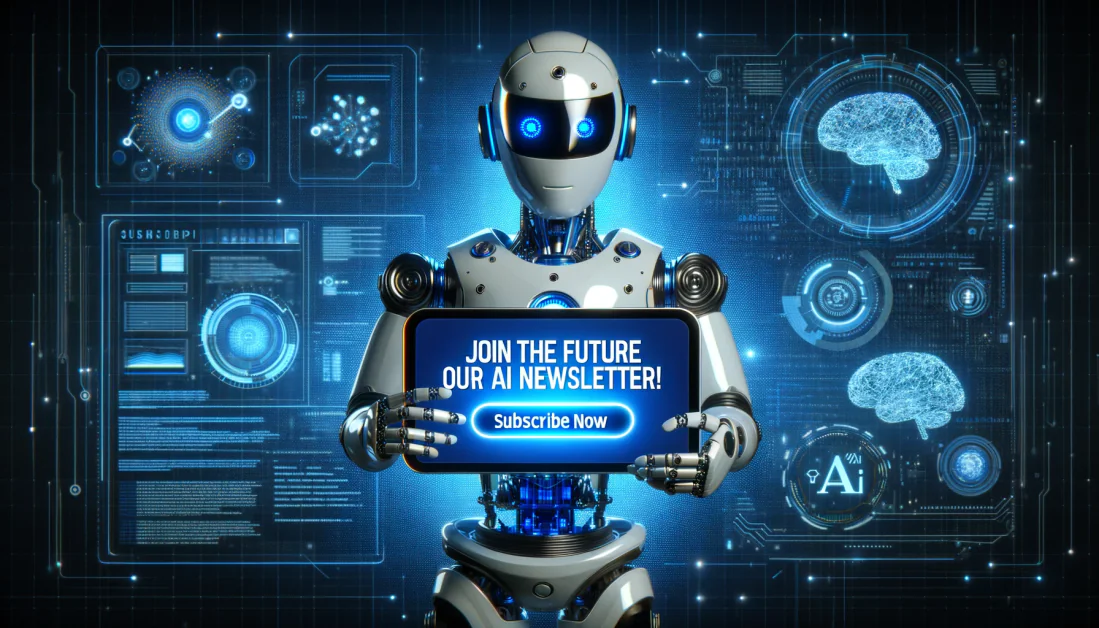
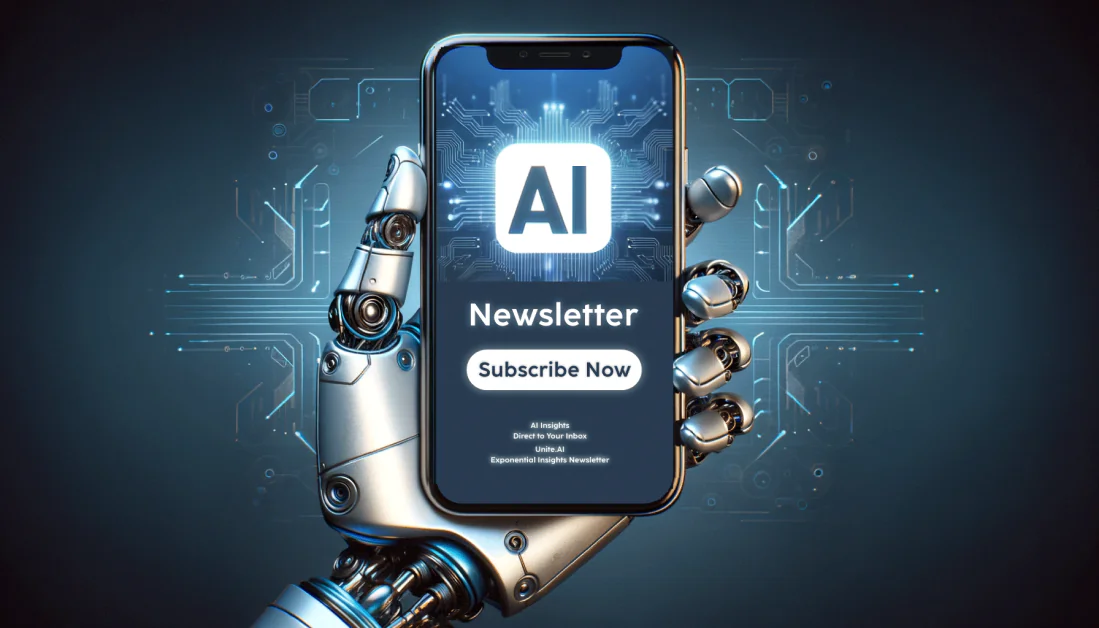