Robotics
NASA to Use Machine Learning to Enhance Search for Alien Life on Mars
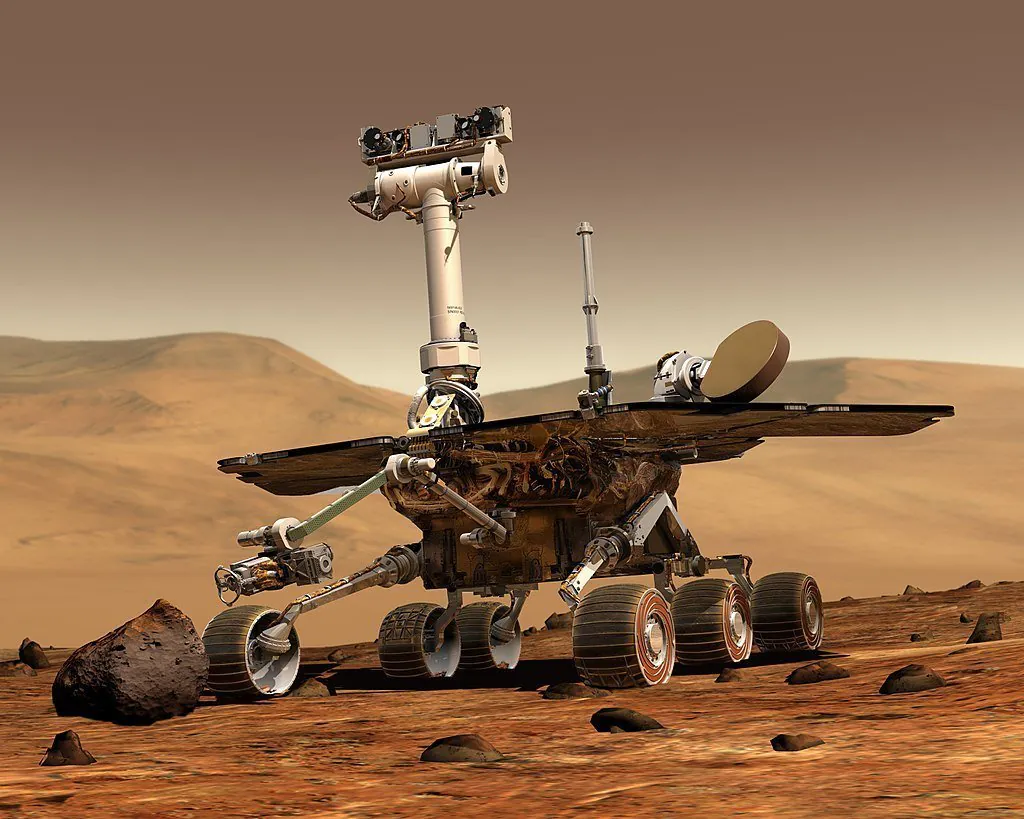
Researchers at NASA have been hard at work on a pilot AI system intended to help future exploration missions find evidence of life on other planets in our solar system. Machine learning algorithms will help exploration devices analyze soil samples on Mars and return the most relevant data to NASA. The pilot program is currently slated for a test run during the ExoMars mission that will see its launch in mid-2022.
As IEEE Spectrum reports, the decision to use machine learning and artificial intelligence to aid the search for life on other planets was driven largely by Erice Lyness, the head of the Goddard Planetary Environments Lab at NASA. Lyness needed to come up with ways of automating aspects of geochemical analyses of samples taken in other parts of our solar system. Lyness decided machine learning could help automate many of the tasks that exploration craft like the Mars rovers must carry out, including the collection and analysis of Martian soil samples.
The ExoMars rover Roslanind Franklin will be capable of drilling at least two meters deep into the martian soil. At this depth, any microbes living there won’t have been killed by the UV light of the sun. This makes it possible that the rover could find living bacteria. Even if no living bacteria samples are found, it’s possible that the drill may find fossilized evidence of life on Mars, held over from earlier eras when the planet was more hospitable to life. The samples that the rover’s drill finds will be given to an instrument called a mass spectrometer for the purpose of analysis.
The mass spectrometer’s purpose is to study the distribution of mass in the ions found within a given sample. This is accomplished by using a laser on the soil sample, which frees up molecules in the soil sample, and then calculating the atomic mass from the different molecules. This process produces a mass spectrum, which researchers will analyze to discern why the patterns of spikes they are seeing in the spectrum could be occurring. There’s an issue with the spectrums generated by the mass spectrometer, however. Various compounds produce a wide variety of different spectrums. It’s a puzzle to analyze a mass spectrum and determine what compounds are within the sample, but machine learning algorithms might be able to help.
The researchers are studying a mineral called montmorillonite. Montmorillonite is commonly found within the Martian soil, and the researchers are aiming to understand how the mineral could manifest itself within a mass spectrum. The team of researchers include montmorillonite samples to see how that output of the mass spectrometer changes, giving them clues as to what the mineral looks like within a mass spectrum. The AI algorithms will assist the researchers in extracting meaningful patterns from the mass spectrometer.
As Lyness was quoted by IEEE Spectrum:
“It could take a long time to really break down a spectrum and understand why you’re seeing peaks at certain [masses] in the spectrum. So anything you can do to point scientists into a direction that says, ‘Don’t worry, I know it’s not this kind of thing or that kind of thing,’ they can more quickly identify what’s in there.”
According to Lyness, the ExoMars mission will be an excellent test case for the AI algorithms designed to help interpret the mass spectrums generated by samples.
There are other potential applications for AI and machine learning in the field of astrobiology. The Dragonfly drone, and potentially another future mision, will be operating farther from Earth and in harsher environments and it will require automating aspects of navigation and the transmission of data.
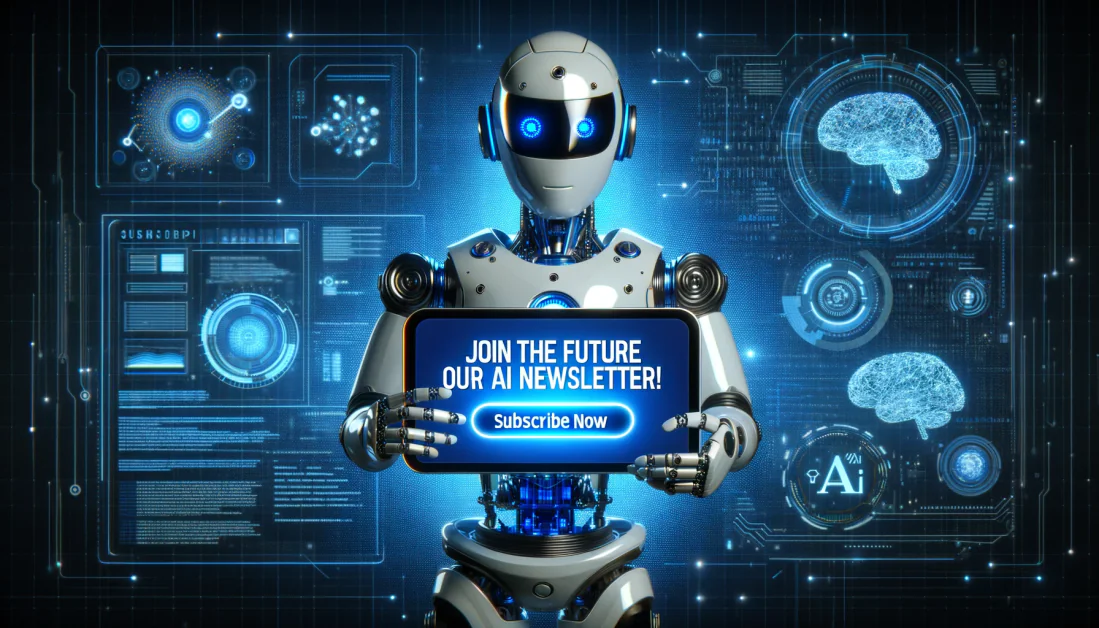
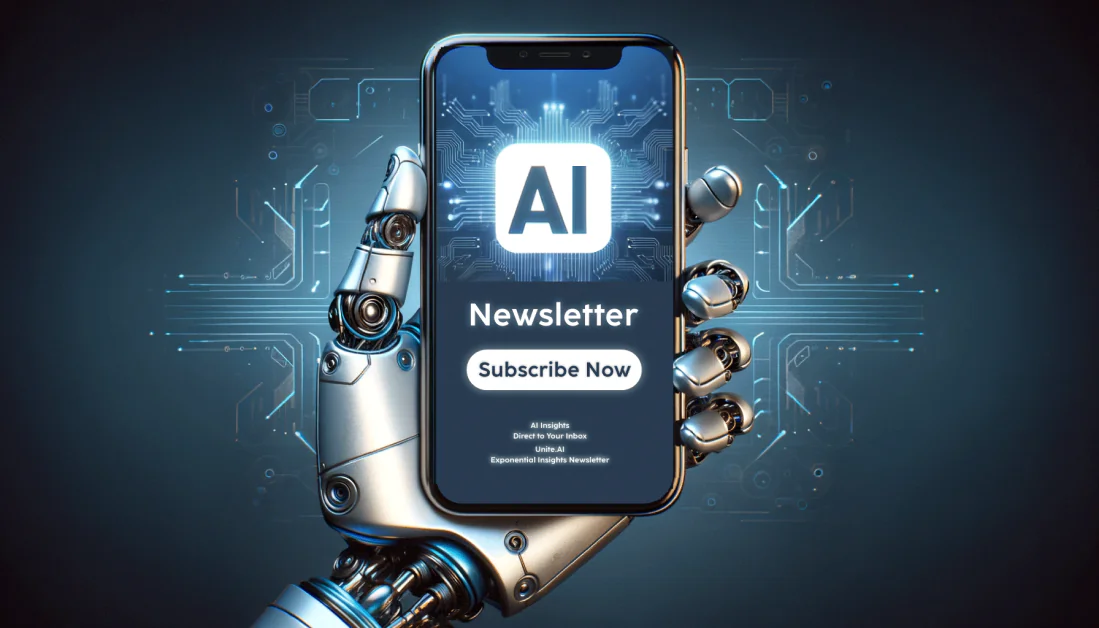