Artificial Intelligence
Algorithm for Predicting Planets’ Orbits Could Be Key to Endless Energy Supply
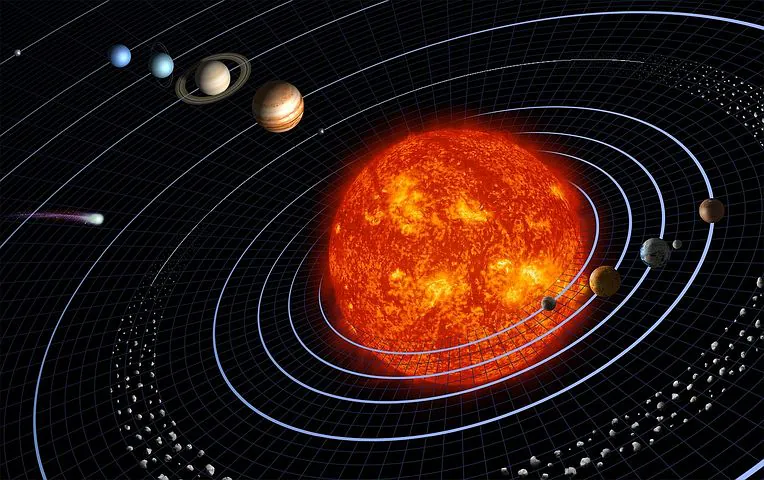
A computer algorithm developed by a scientist at the U.S Department of Energy’s (DOE) Princeton Plasma Physics Laboratory (PPPL) can accurately predict the orbit of planets in the solar system. It relies on artificial intelligence (AI) to develop predictions based on previous experience.
Now, the fundamentals of that algorithm are being adapted in other domains, specifically in regard to predicting and controlling plasma in fusion facilities. These facilities can harvest fusion energy, which powers the sun and stars, on Earth.
Hong Qin is a PPPL physicist and author of the paper published in Scientific Reports.
“Usually in physics, you make observations, create a theory based on those observations, and then use that theory to predict new observations,” said Qin. “What I'm doing is replacing this process with a type of black box that can produce accurate predictions without using a traditional theory or law.”
The Computer Program
The computer program developed by Qin relies on fed data from past observations regarding the orbits of Earth, Mars, Mercury, Venus, Jupter, and the dwarf planet Ceres. Along with a “serving algorithm,” accurate predictions of the orbits of other planets can be made, all without the use of Newton’s laws of motion and gravitation.
“Essentially, I bypassed all the fundamental ingredients of physics. I go directly from data to data,” Qin said. “There is no law of physics in the middle.”
Joshua Burby is a physicist at the DOE’s Los Alamos National Laboratory. He received a Ph.D under Qin.
“Hong taught the program the underlying principle used by nature to determine the dynamics of any physical system,” said Burby. “The payoff is that the network learns the laws of planetary motion after witnessing very few training examples. In other words, his code really ‘learns' the laws of physics.”
Fusion Experiments
These principles and theories are now being adapted by Qi and Eric Palmerduca, who is a graduate student in the Princeton University Program in Plasma Physics. The pair are attempting to use them to predict the behavior of plasma particles in fusion experiments, which take place all around the world.
Fusion is combined light elements in the form of plasma, and it is the power behind stars and the sun. Plasma represents 99% of the visible universe, and it carries the potential to produce massive amounts of energy. If scientists can replicate fusion on Earth, it would provide us with an endless supply of power for electricity.
“In a magnetic fusion device, the dynamics of plasmas are complex and multi-scale, and the effective governing laws or computational models for a particular physical process that we are interested in are not always clear,” Qin said. “In these scenarios, we can apply the machine learning technique that I developed to create a discrete field theory and then apply this discrete field theory to understand and predict new experimental observations.”
The scientists also believe that this new technique could develop a traditional physical theory.
While in some sense this method precludes the need of such a theory, it can also be viewed as a path toward one,” Palmerduca said. “When you're trying to deduce a theory, you'd like to have as much data at your disposal as possible. If you're given some data, you can use machine learning to fill in gaps in that data or otherwise expand the data set.”
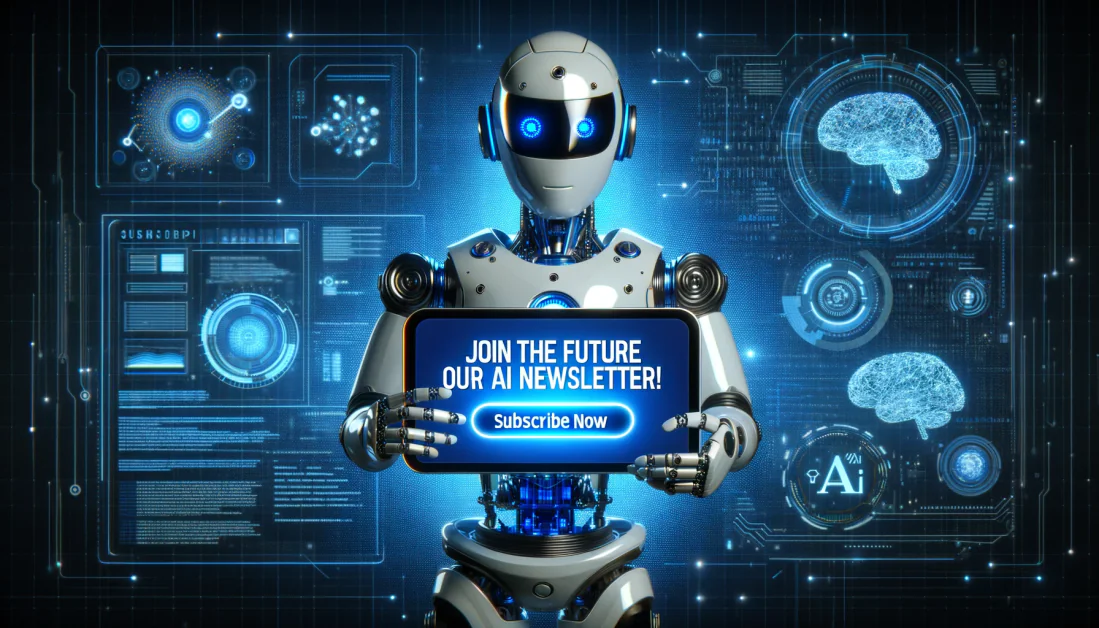
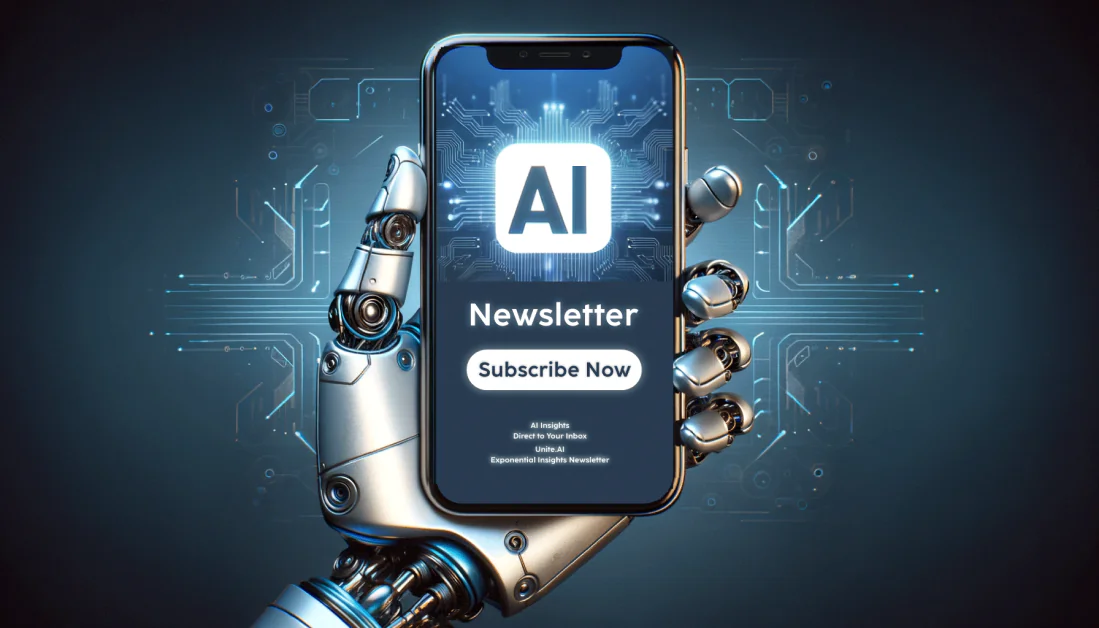