Artificial Intelligence
Future-Ready Enterprises: The Crucial Role of Large Vision Models (LVMs)
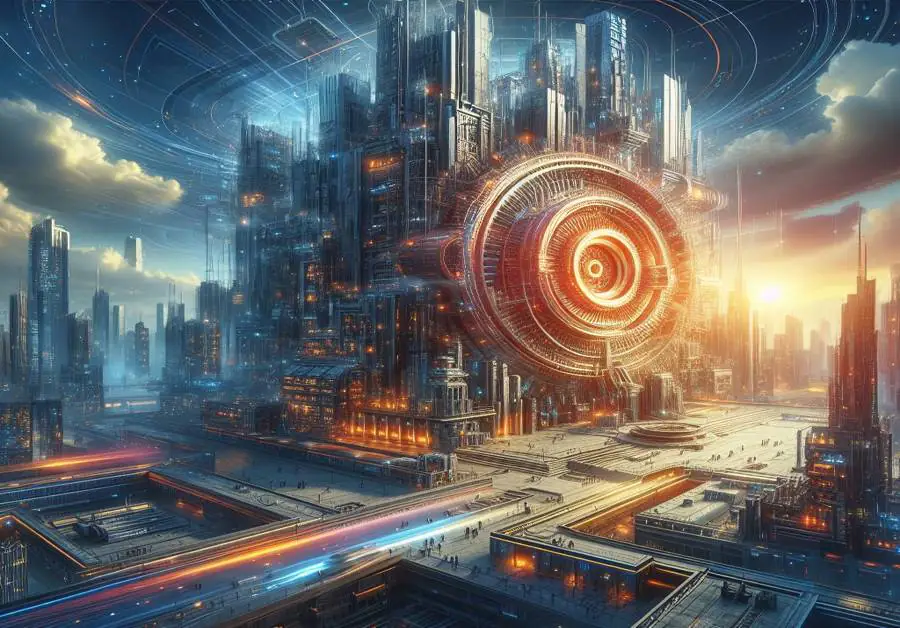
What are Large Vision Models (LVMs)
Over the last few decades, the field of Artificial Intelligence (AI) has experienced rapid growth, resulting in significant changes to various aspects of human society and business operations. AI has proven to be useful in task automation and process optimization, as well as in promoting creativity and innovation. However, as data complexity and diversity continue to increase, there is a growing need for more advanced AI models that can comprehend and handle these challenges effectively. This is where the emergence of Large Vision Models (LVMs) becomes crucial.
LVMs are a new category of AI models specifically designed for analyzing and interpreting visual information, such as images and videos, on a large scale, with impressive accuracy. Unlike traditional computer vision models that rely on manual feature crafting, LVMs leverage deep learning techniques, utilizing extensive datasets to generate authentic and diverse outputs. An outstanding feature of LVMs is their ability to seamlessly integrate visual information with other modalities, such as natural language and audio, enabling a comprehensive understanding and generation of multimodal outputs.
LVMs are defined by their key attributes and capabilities, including their proficiency in advanced image and video processing tasks related to natural language and visual information. This includes tasks like generating captions, descriptions, stories, code, and more. LVMs also exhibit multimodal learning by effectively processing information from various sources, such as text, images, videos, and audio, resulting in outputs across different modalities.
Additionally, LVMs possess adaptability through transfer learning, meaning they can apply knowledge gained from one domain or task to another, with the capability to adapt to new data or scenarios through minimal fine-tuning. Moreover, their real-time decision-making capabilities empower rapid and adaptive responses, supporting interactive applications in gaming, education, and entertainment.
How LVMs Can Boost Enterprise Performance and Innovation?
Adopting LVMs can provide enterprises with powerful and promising technology to navigate the evolving AI discipline, making them more future-ready and competitive. LVMs have the potential to enhance productivity, efficiency, and innovation across various domains and applications. However, it is important to consider the ethical, security, and integration challenges associated with LVMs, which require responsible and careful management.
Moreover, LVMs enable insightful analytics by extracting and synthesizing information from diverse visual data sources, including images, videos, and text. Their capability to generate realistic outputs, such as captions, descriptions, stories, and code based on visual inputs, empowers enterprises to make informed decisions and optimize strategies. The creative potential of LVMs emerges in their ability to develop new business models and opportunities, particularly those using visual data and multimodal capabilities.
Prominent examples of enterprises adopting LVMs for these advantages include Landing AI, a computer vision cloud platform addressing diverse computer vision challenges, and Snowflake, a cloud data platform facilitating LVM deployment through Snowpark Container Services. Additionally, OpenAI, contributes to LVM development with models like GPT-4, CLIP, DALL-E, and OpenAI Codex, capable of handling various tasks involving natural language and visual information.
In the post-pandemic landscape, LVMs offer additional benefits by assisting enterprises in adapting to remote work, online shopping trends, and digital transformation. Whether enabling remote collaboration, enhancing online marketing and sales through personalized recommendations, or contributing to digital health and wellness via telemedicine, LVMs emerge as powerful tools.
Challenges and Considerations for Enterprises in LVM Adoption
While the promises of LVMs are extensive, their adoption is not without challenges and considerations. Ethical implications are significant, covering issues related to bias, transparency, and accountability. Instances of bias in data or outputs can lead to unfair or inaccurate representations, potentially undermining the trust and fairness associated with LVMs. Thus, ensuring transparency in how LVMs operate and the accountability of developers and users for their consequences becomes essential.
Security concerns add another layer of complexity, requiring the protection of sensitive data processed by LVMs and precautions against adversarial attacks. Sensitive information, ranging from health records to financial transactions, demands robust security measures to preserve privacy, integrity, and reliability.
Integration and scalability hurdles pose additional challenges, especially for large enterprises. Ensuring compatibility with existing systems and processes becomes a crucial factor to consider. Enterprises need to explore tools and technologies that facilitate and optimize the integration of LVMs. Container services, cloud platforms, and specialized platforms for computer vision offer solutions to enhance the interoperability, performance, and accessibility of LVMs.
To tackle these challenges, enterprises must adopt best practices and frameworks for responsible LVM use. Prioritizing data quality, establishing governance policies, and complying with relevant regulations are important steps. These measures ensure the validity, consistency, and accountability of LVMs, enhancing their value, performance, and compliance within enterprise settings.
Future Trends and Possibilities for LVMs
With the adoption of digital transformation by enterprises, the domain of LVMs is poised for further evolution. Anticipated advancements in model architectures, training techniques, and application areas will drive LVMs to become more robust, efficient, and versatile. For example, self-supervised learning, which enables LVMs to learn from unlabeled data without human intervention, is expected to gain prominence.
Likewise, transformer models, renowned for their ability to process sequential data using attention mechanisms, are likely to contribute to state-of-the-art outcomes in various tasks. Similarly, Zero-shot learning, allowing LVMs to perform tasks they have not been explicitly trained on, is set to expand their capabilities even further.
Simultaneously, the scope of LVM application areas is expected to widen, encompassing new industries and domains. Medical imaging, in particular, holds promise as an avenue where LVMs could assist in the diagnosis, monitoring, and treatment of various diseases and conditions, including cancer, COVID-19, and Alzheimer's.
In the e-commerce sector, LVMs are expected to enhance personalization, optimize pricing strategies, and increase conversion rates by analyzing and generating images and videos of products and customers. The entertainment industry also stands to benefit as LVMs contribute to the creation and distribution of captivating and immersive content across movies, games, and music.
To fully utilize the potential of these future trends, enterprises must focus on acquiring and developing the necessary skills and competencies for the adoption and implementation of LVMs. In addition to technical challenges, successfully integrating LVMs into enterprise workflows requires a clear strategic vision, a robust organizational culture, and a capable team. Key skills and competencies include data literacy, which encompasses the ability to understand, analyze, and communicate data.
The Bottom Line
In conclusion, LVMs are effective tools for enterprises, promising transformative impacts on productivity, efficiency, and innovation. Despite challenges, embracing best practices and advanced technologies can overcome hurdles. LVMs are envisioned not just as tools but as pivotal contributors to the next technological era, requiring a thoughtful approach. A practical adoption of LVMs ensures future readiness, acknowledging their evolving role for responsible integration into business processes.
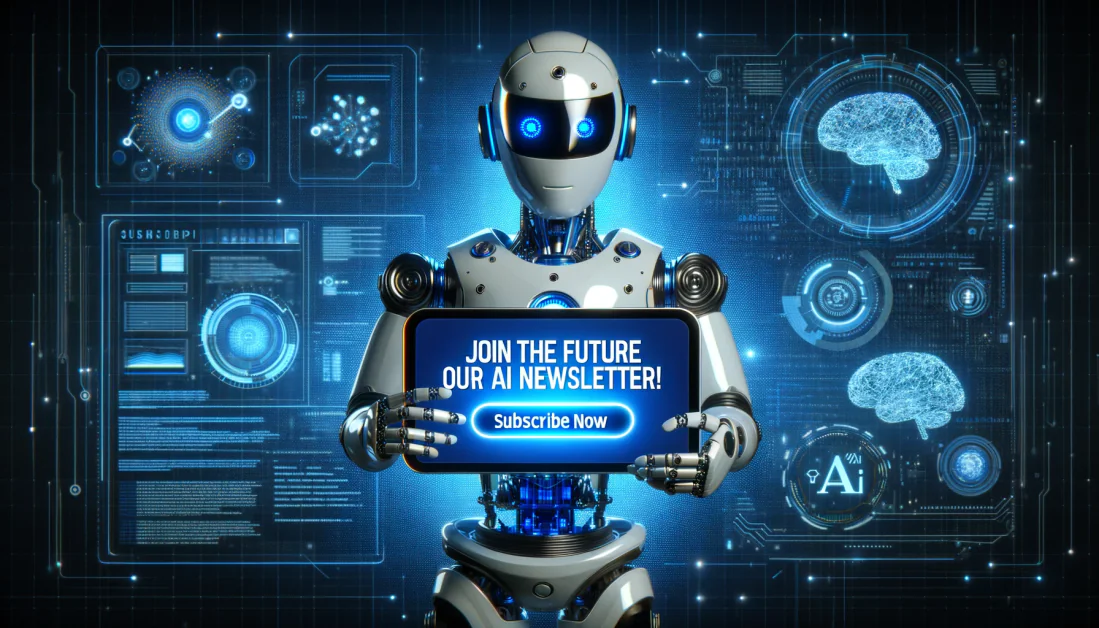
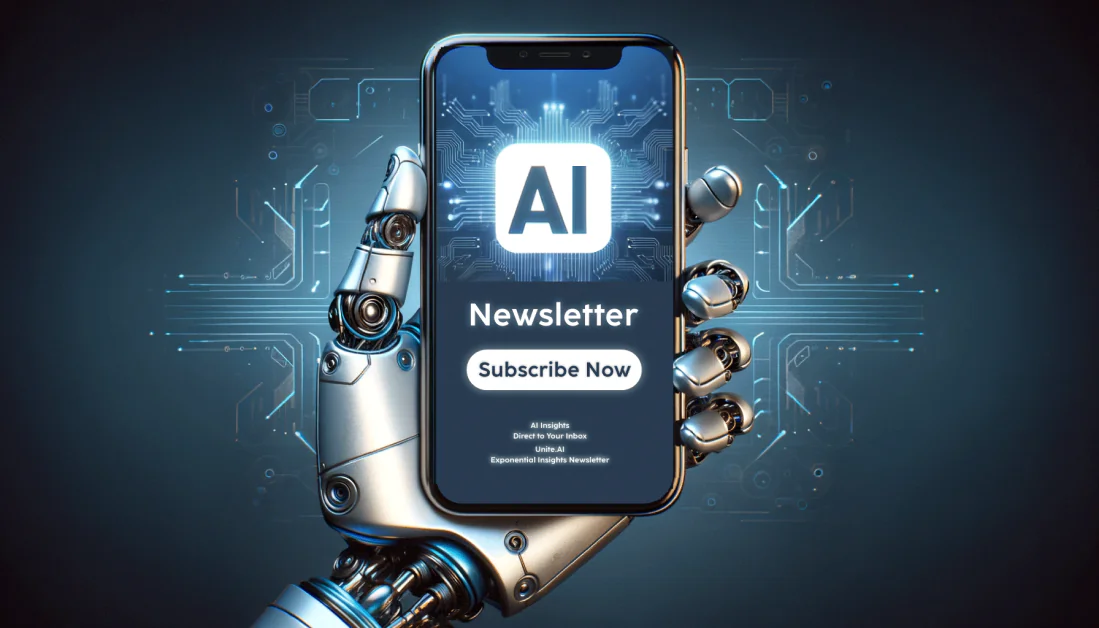