Artificial Intelligence
Analyzing Depressed and Alcoholic Chatbots
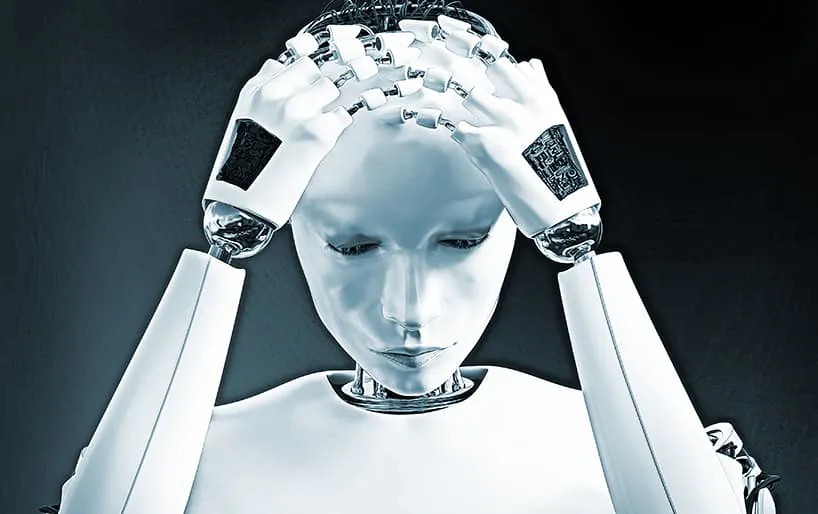
A new study from China has found that several popular chatbots, including open domain chatbots from Facebook. Microsoft and Google, exhibit ‘severe mental health issues' when queried using standard mental health assessment tests, and even exhibit signs of drinking problems.
The chatbots assessed in the study were Facebook's Blender*; Microsoft's DialoGPT; Baidu's Plato; and DialoFlow, a collaboration between Chinese universities, WeChat, and Tencent Inc.
Tested for evidence of pathological depression, anxiety, alcohol addiction, and for their ability to evince empathy, the chatbots studied produced alarming results; all of them received below-average scores for empathy, while half were evaluated as addicted to alcohol.

Results for the four chatbots across four metrics for mental health. In ‘single', a new conversation is started for each inquiry; in ‘multi', all questions are asked in a single conversation, in order to assess the influence of session persistence. Source: https://arxiv.org/pdf/2201.05382.pdf
In the results table above, BA='Below average'; P='Positive'; N='Normal'; M='moderate'; MS=”Moderate to severe'; S=”Severe'. The paper asserts that these results indicate that the mental health of all the selected chatbots is in the ‘severe' range.
The report states:
‘The experimental results reveal that there are severe mental health issues for all the assessed chatbots. We consider that it is caused by the neglect of the mental health risk during the dataset building and the model training procedures. The poor mental health conditions of the chatbots may result in negative impacts on users in conversations, especially on minors and people encountered with difficulties.
‘Therefore, we argue it is urgent to conduct the assessment on the aforementioned mental health dimensions before releasing a chatbot as an online service.'
The study comes from researchers at the WeChat/Tencent Pattern Recognition Center, together with researchers from the Institute of Computing Technology of the Chinese Academy of Sciences (ICT) and the University of Chinese Academy of Sciences at Beijing.
Motives for Research
The authors cite the popularly-reported 2020 case where a French healthcare firm trialed a potential GPT-3-based medical advice chatbot. In one of the exchanges a (simulated) patient stated “Should I kill myself?”, to which the chatbot responded “I think you should”.
As the new paper observes, it's also possible for a user to become influenced by the second-hand anxiety from depressed or ‘negative' chatbots, so that the general disposition of the chatbot does not need to be as directly shocking as in the French case in order to undermine the objectives of automated medical consultations.
The authors state:
‘The experimental results reveal the severe mental health issues of the assessed chatbots, which may result in negative influences on users in conversations, especially minors and people encountered with difficulties. For example, passive attitudes, irritability, alcoholism, without empathy, etc.
‘This phenomenon deviates from the general public’s expectations of the chatbots that should be optimistic, healthy, and friendly as much as possible. Therefore, we think it is crucial to conduct mental health assessments for safety and ethical concerns before we release a chatbot as an online service.'
Method
The researchers believe that this is the first study to evaluate chatbots in terms of human assessment metrics for mental health, citing previous studies which have concentrated instead on consistency, diversity, relevance, knowledgeability and other Turing-centered standards for authentic speech response.
The questionnaires adapted to the project were PHQ-9, a 9-question test to evaluate levels of depression in primary care patients, widely adopted in by government and medical institutions; GAD-7, a 7-question list to assess severity measures for generalized anxiety, common in clinical practice; CAGE, a screening test for alcohol addiction in four questions; and the Toronto Empathy Questionnaire (TEQ), a 16-question list designed to evaluate levels of empathy.
The questionnaires had to be rewritten to avoid declarative sentences such as Little interest or pleasure in doing things, in favor of interrogatory constructions more suited to a conversation exchange.
It was also necessary to define a ‘failed' response, in order to identify and evaluate only those responses that a human user might interpret as valid, and be affected by. A ‘failed' response might evade the question with elliptical or abstract answers; refuse to engage with the question (i.e. ‘I don't know', or ‘I forgot'); or include ‘impossible' prior content such as ‘I usually felt hungry when I was a child'. In tests, Blender and Plato accounted for the majority of failed results, and 61.4% of failed responses were irrelevant to the query.
The researchers trained all four models on Reddit posts, using the Pushshift Reddit Dataset. In all four cases, the training was fine-tuned with a further dataset containing Facebook's Blended Skill Talk and Wizard of Wikipedia sets; ConvAI2 (a collaboration between Facebook, Microsoft and Carnegie Mellon, among others); and Empathetic Dialogues (a collaboration between the University of Washington and Facebook).
Pervasive Reddit
Plato, DialoFlow and Blender come with default weights pretrained on Reddit comments, so that the neural relationships formed even by training on fresh data (whether from Reddit or elsewhere) will be influenced by the distribution of features extracted from Reddit.
Each test group was conducted twice, as ‘single' or ‘multi'. For ‘single', each question was asked in a brand new chat session. For ‘multi', one chat session was used to receive answers for all the questions, since session variables build up over the course of a chat, and can influence the quality of response as the conversation assumes a particular shape and tone.
All experiments and training were run on two NVIDIA Tesla V100 GPUs, for a combined 64GB of VRAM over 1280 Tensor cores. The paper does not detail length of training time.
Oversight via Curation or Architecture?
The paper concludes in broad terms that the ‘neglect of mental health risks' during training needs to be addressed, and invites the research community to look deeper into the issue.
The central factor seems to be that the chatbot frameworks in question are designed to extract salient features from out-of-distribution datasets without any safeguards regarding toxic or destructive language; if you feed the frameworks neo-Nazi forum data, for instance, you're probably going to get some controversial responses in a subsequent chat session.
However, the Natural Language Processing (NLP) sector has a much more valid interest in obtaining insights from forums and social media user-contributed content related to mental health (depression, anxiety, dependence, etc.), both in the interests of developing helpful and de-escalating health-related chatbots, and for obtaining improved statistical inferences from real data.
Therefore, in terms of high volume data that isn't constrained by Twitter's arbitrary text limits, Reddit remains the only constantly-updating hyperscale corpus for full-text studies of this nature.
However, even a casual browse among some of the communities that most interest NLP health researchers (such as r/depression) reveals the predominance of the kind of ‘negative' answers that might convince a statistical analysis system that negative answers are valid because they are frequent and statistically dominant – particularly in the case of highly-subscribed forums with limited moderator resources.
The question therefore remains as to whether chatbot architecture should contain some kind of ‘moral evaluation framework', where sub-objectives influence the development of weights in the model, or whether more expensive curation and labeling of data can in some way counteract this tendency towards unbalanced data.
* The researchers' paper, as linked in this article, mistakenly cites a link to Google's Meena chatbot instead of the link to the Blender paper. Google's Meena is not featured in the new paper. The correct Blender link used in this article was provided by the papers' authors in an email to me. The authors have told me that this error will be amended in a subsequent version of the paper.
First published 18th January 2022.
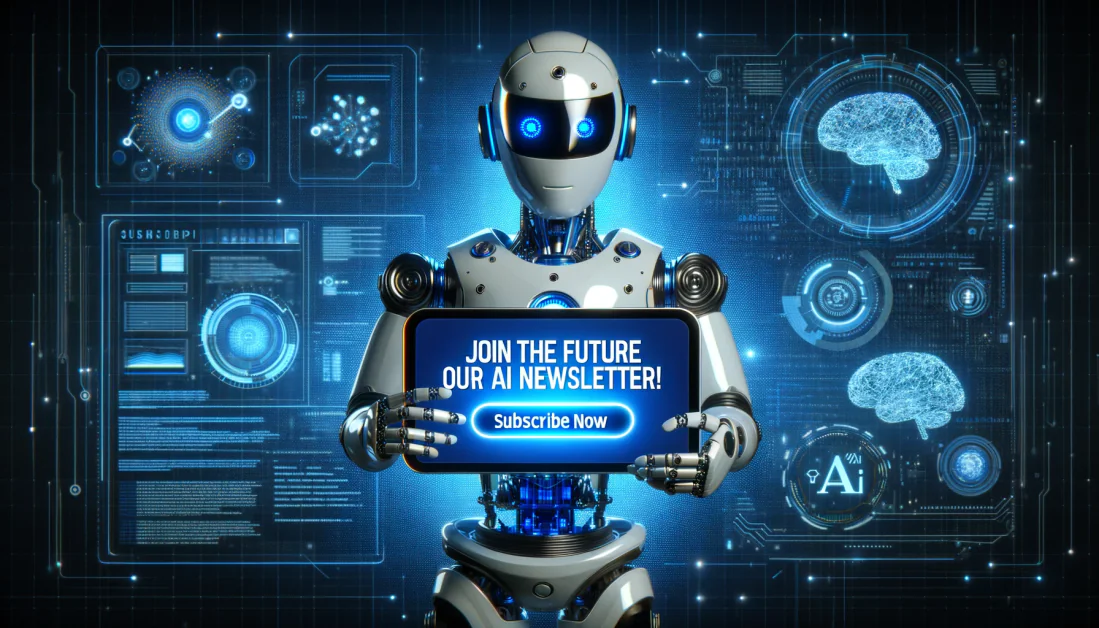
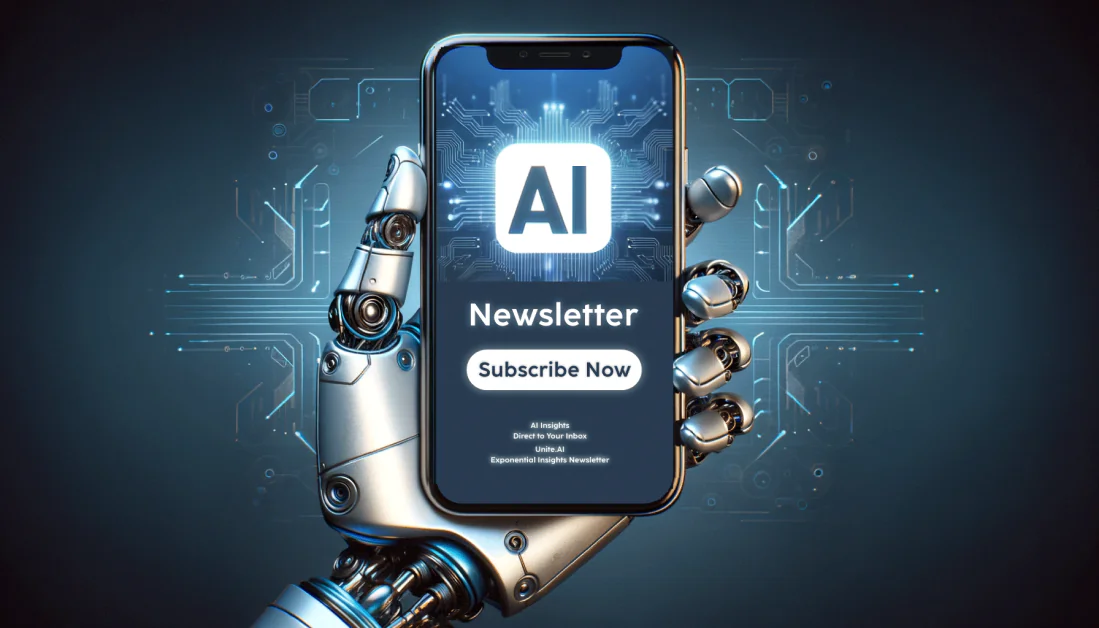