Artificial Intelligence
AI Researchers Develop Explainable Neural Network to Discover Genomic Rules
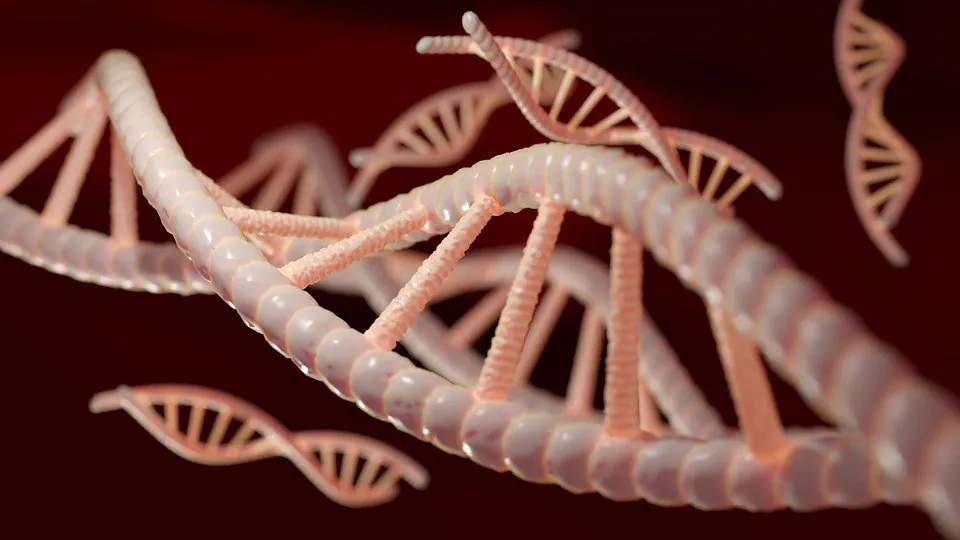
A team of researchers has recently created an explainable neural network intended to help biologists uncover the mysterious rules that govern the code of the human genome. The research team trained a neural network on maps of protein-DNA interactions, enabling the AI to discover how certain DNA sequences regulate certain genes. The researchers also made the model explainable, so that they could analyze the model’s conclusions and determine how to sequence motifs regulate genes.
One of the big mysteries in biology is the regulatory code of the genome. It’s known that DNA is comprised of four nucleotide bases – Adenine, Guanine, Thymine, and Cytosine – but it isn’t known how these base pairs are used to regulate activity. The four nucleotide bases encode the instructions for building proteins, but they also control where and how genes are expressed, (how they make proteins in an organism). Particular combinations and arrangements of the bases create sections of regulatory code that bind to segments of DNA, and it’s unknown just what these combinations are.
An interdisciplinary team of computer scientists and biologists set out to solve this mystery by creating an explainable neural network. The research team created a neural network they dubbed “Base Pair Network” or “BPNet”. The model used by BPNet to generate predictions can be interpreted to identify regulatory codes. This was accomplished by predicting how proteins called transcription factors bind with DNA sequences.
The researchers performed a variety of experiments and did comprehensive computer modeling to determine how transcription factors and DNA were bound together, developing a detailed map down to the level of individual nucleotide bases. The detailed transcription factor-DNA representations let the researchers create tools capable of interpreting both critical DNA sequence patterns and the rules that function as regulatory code.
Julia Zeitlinger, PhD biologist and computational researcher at Stanford University, explained that the results gathered from the explainable neural network meshed with existing experimental results, but they also contained surprising insights into the regulatory code of the genome. As an example, the AI model allowed the research team to discover a rule that influences how a transcription factor called Nanog operates. When multiple instances of the Nanog motif are present on the same side of a DNA double helix, they bind cooperatively to the DNA. As Zeitlinger explained via ScienceDaily:
“There has been a long trail of experimental evidence that such motif periodicity sometimes exists in the regulatory code. However, the exact circumstances were elusive, and Nanog had not been a suspect. Discovering that Nanog has such a pattern, and seeing additional details of its interactions, was surprising because we did not specifically search for this pattern.”
The recent research paper is far from the first study to use AI to analyze DNA, but it’s likely the first study to open the “black box” of AI to discern which DNA sequences regulate genes in the genome. Neural networks excel at findings patterns within data, but their insights are difficult to extract from the models they create. By creating a method of analyzing which features the model considers important to the prediction of genomic rules the researchers could train more nuanced models that lead to novel discoveries.
The architecture of BPNet is similar to networks used to recognize faces in images. When computer vision systems recognize faces in images, the network starts by detecting edges and then joins these edges together. The difference is that BPNet learns from DNA sequences, detecting sequence motifs and joining these motifs together into the higher-order rules that can be used to predict the binding of data at the base-resolution.
After the model has hit a high accuracy threshold, the patterns learned by the model are traced back to the original input sequences, revealing the sequence motifs. Finally, the model is provided with systematic DNA sequence queries, letting the researchers understand the rules by which sequence motifs combine and function. According to Zeitlinger, the model is capable of predicting many more sequences than the researchers could hope to test in a traditional, experimental fashion. Additionally, predicting the outcome of experimental anomalies let the researchers identify which experiments were most informative when validating the model.
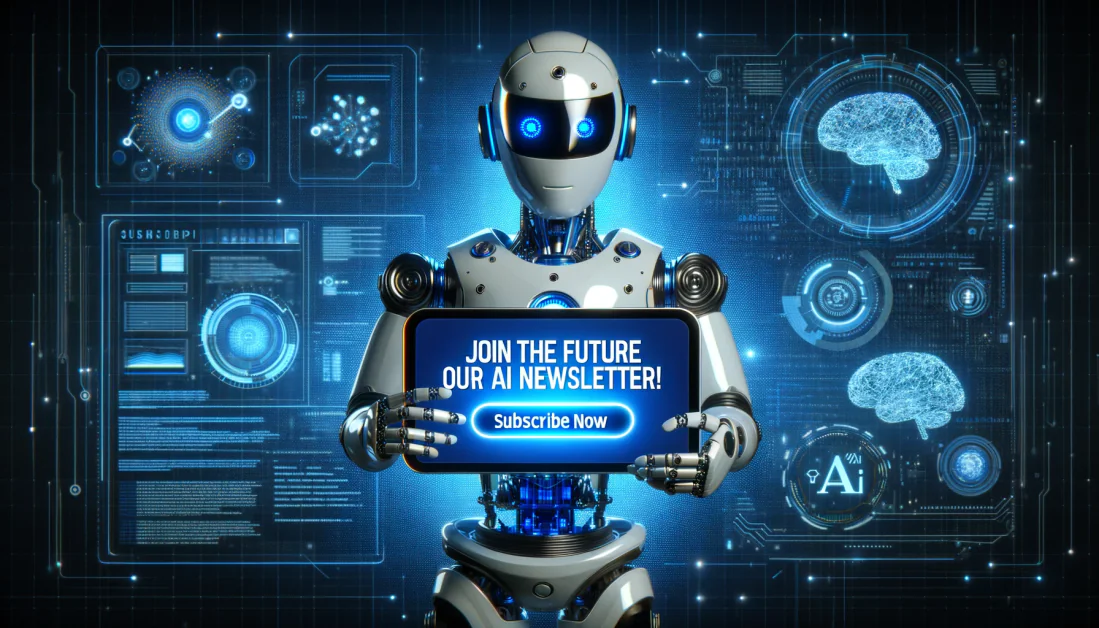
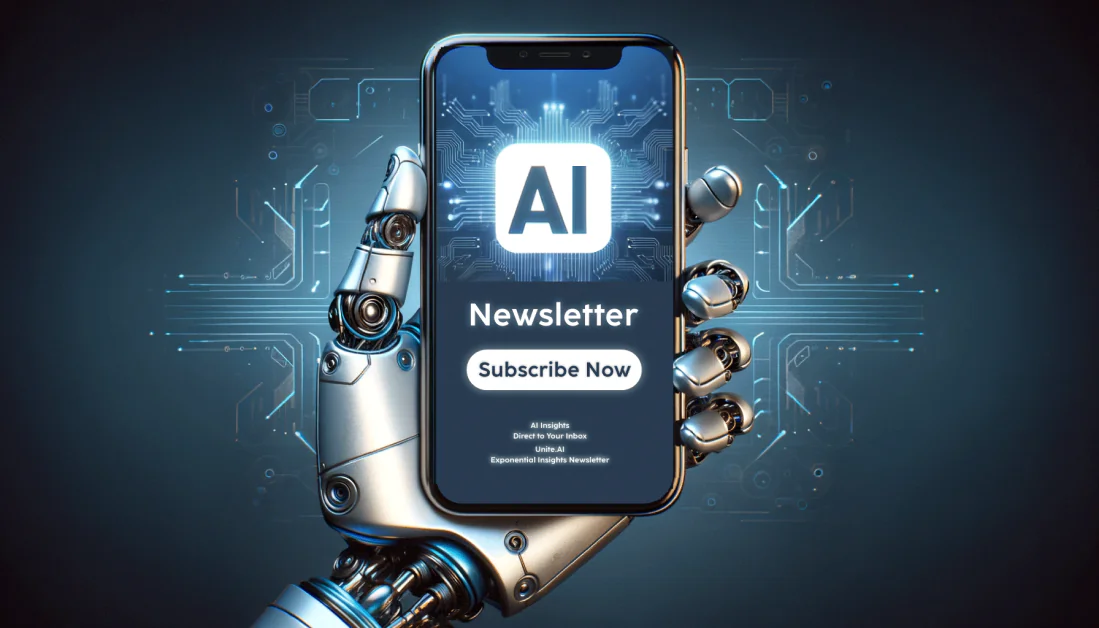