Artificial Intelligence
Why Are Self-Driving Cars the Future and How are They Created?
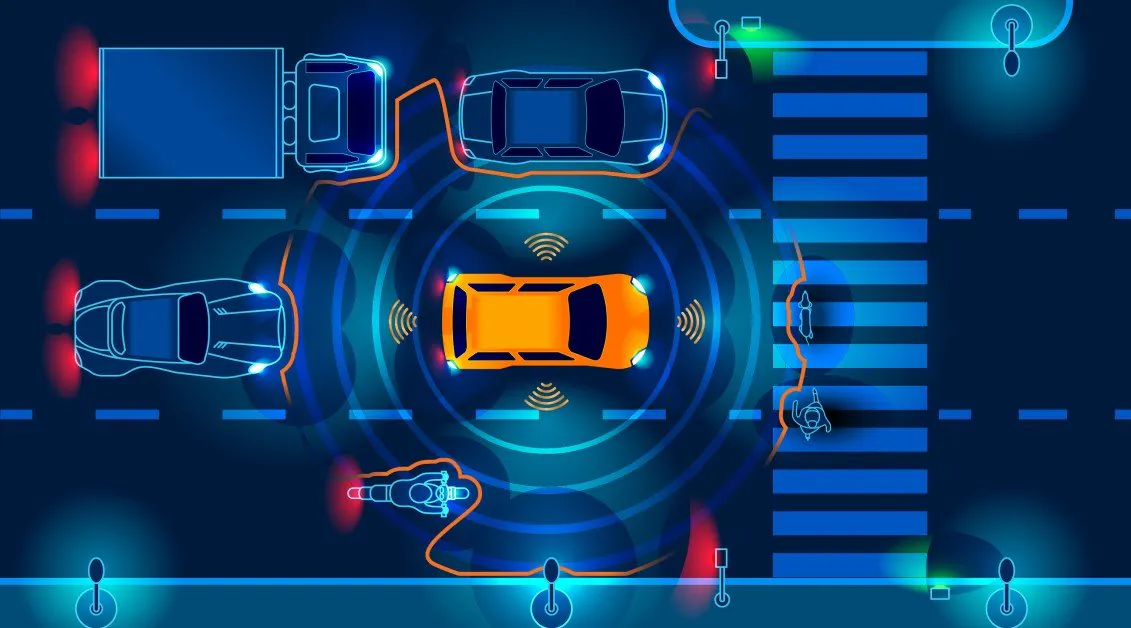
Due to the recent adaptive quarantine measures imposed in virtually all parts of the world, air travel, public transportation, and many other sectors took a really big hit in 2020. However, the automotive world and autonomous vehicles, in particular, have shown increased resilience during this difficult time. In fact, companies like Ford have increased their investments in the development of electric and self-driving cars by allocating $29 billion dollars in the fourth quarter of last year. Specifically, $7 billion of that money will go towards the development of self-driving cars. So Ford is joining General Motors, Tesla, Baidu, and other automakers in heavily investing in autonomous vehicles. In this article, we will tell you about why companies invest in self-driving cars and how the machine learning algorithms that power them are trained.
Why are So Many Companies Investing in Self-Driving Cars?
When we take a look at all of the benefits offered by autonomous vehicles, it is easy to see why so many companies are investing in their development. Drivers will be able to save more money since they will not have to pay for expensive insurance plans, it will speed up their daily commutes, improve the fuel economy, and many other benefits. For companies, such automation opens the door for greater savings. A great example of this is autonomous long-haul trucking which will be able to cut operating costs by 45%, according to a report by McKinsey & Company.
The main benefit has to be increased safety. According to the NHTSA, 94% of serious crashes are the result of human error. Self-driving cars can significantly reduce the number of accidents since they do not require any driver input and have a 360-degree view at all times. Also, advanced driver safety systems (ADAS) can take over safety-critical functions in dangerous situations such as braking and steering. There are lots of added value autonomous vehicles offer society like reduced emissions. In fact, a basic case showed a 9% reduction in energy and GHG emissions in the vehicle's whole life compared with those of a conventional vehicle. Not that we know all of the benefits self-driving cars have to offer, let’s take a look at how they are trained to recognize the world around them.
How Do AVs Work and How AVs Can Become a Reality
An autonomous vehicle needs to follow the rules of the road and to do that, it needs to recognize all of the various traffic signs, road markings, detect other vehicles and pedestrians, and countless other objects. These AI vehicles rely on machine learning to “calculate” what needs to be done in all kinds of driving situations. Let’s start off with a basic example. A person is in their AV driving on the highway to get to work. The car will need to correctly identify the posted speed limit, maintain a safe distance from the car in front and, when it enters a residential area, it needs to recognize pedestrians and let them cross the road.
This requires thousands and thousands of images to be annotated by techniques ranging from labeling all the way to semantic segmentation. In fact, Evgenia Khimenko, the CEO of Mindy Support, a company that provides data annotation services for the automotive sector, says that there a wide range of data annotation projects for the automotive industry are possible:
“These include projects like face recognition on videos to train self-driving cars to identify the behavior of other drivers on the road, video labeling and annotation to detect movement and direction of the vehicle (we annotated more than 545 million image sequences). Another sophisticated audio annotation task was when we had to identify the timestamp and label human speech as well as all the background noise happening inside the vehicle such as radio, laughing, shouting, singing, animals, and even silence”.
Let’s consider a complex scenario. Imagine that the autonomous vehicle is driving in a residential neighborhood and there are teenagers with skateboards who are waiting to cross the road. According to the rules, the car has the right of way, but there is a good chance that the teenagers will not wait for the light to turn green and will try to cross the road prematurely. A human driver will be well aware of such risk and will slow down to anticipate such an event, but for a machine, this would be very difficult to calculate. This is the next step researchers are trying to take with autonomous vehicles and simply more annotated data may be the answer.
How Do the AVs See the Physical World?
Autonomous vehicles rely on LiDAR technology to help them see the world around them. LiDAR creates a 3D point cloud which is a digital representation of how the AI system views the world. This technology is not reserved just for autonomous vehicles, it is also used for other robotic process automation jobs such as creating a robot that can harvest crops for the agriculture sector. The 3D point cloud will also need to be annotated so the machine knows what exactly it is seeing. This is usually done with techniques such as labeling, 3D boxes, and semantic segmentation. A more advanced form of annotation would be to color code the 3D point cloud so the vehicle understands the distance of the object.
The way LiDAR works is that it sends a signal of light to all of the objects surrounding it and depending on how long it takes the light to return, it gives the AI an understanding of how far away the object is. For example, the ground on the 3D point cloud will always be blue because it is the lowest point, the light will bounce off quickly and blue has a very short wavelength. One of the surrounding buildings may be red or orange depending on how far away it is.
It is worth noting that LiDAR is not the only game in town. For example, Tesla uses something called the Hydrant, which is a combination of eight cameras that stitch together a complete picture of the road. Other companies, like Waymo and Voyage, use LiDAR. One possible reason that Tesla may be avoiding LiDAR is that it is very bulky and ruins the overall appearance of the car. After all, Teslas are very expensive and drivers will probably not want a giant box sitting on the roof of their cars. Companies developing robotaxis, like Waymo, may be able to get away with using LiDAR.
Why is Quality Training Data So Important?
Having quality training data is one of the most essential things you need to have to create a self-driving car. However, simply obtaining this data is not enough. The training datasets need to be prepared via data annotation so the AI system can learn from them. While this is a very time-consuming and tedious process, the success of the entire project depends on it. After all, self-driving cars are the future and can potentially help us reduce or even eliminate some of the issues we are experiencing in terms of car accidents and casualties, environmental issues and the gridlock on the roads.
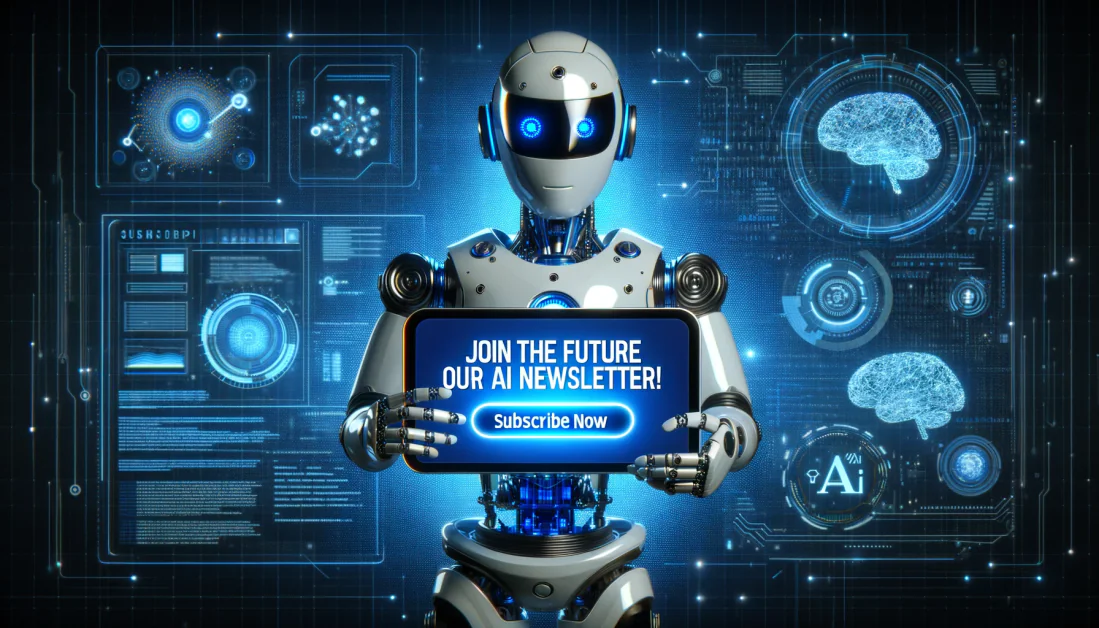
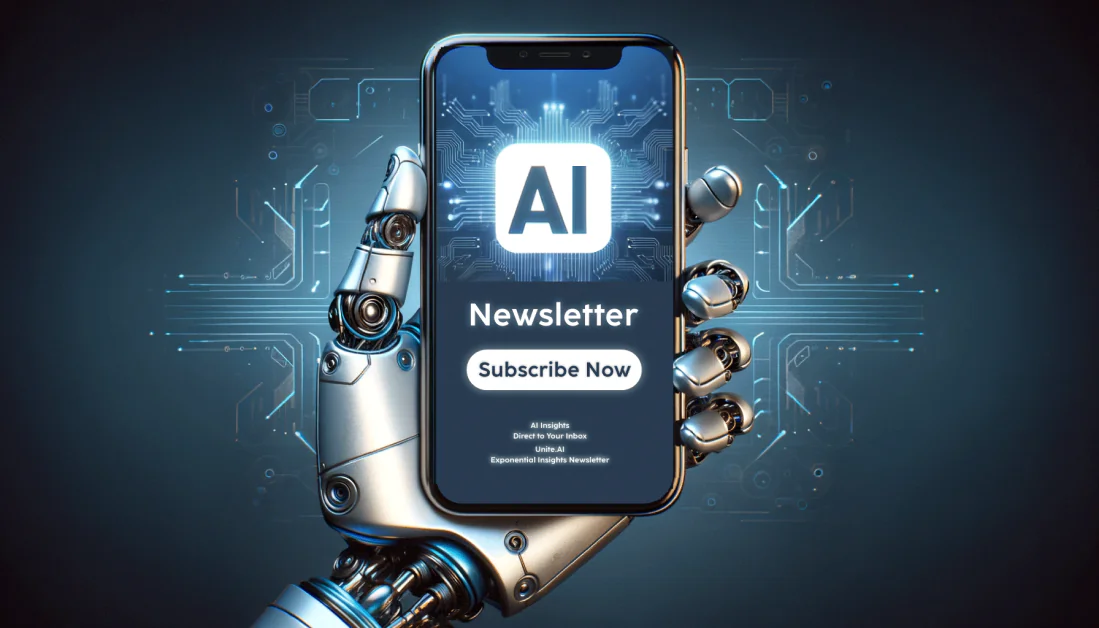