Artificial Intelligence
RE•WORK White Paper: The Challenges, Successes, Progression & Failures of Processing in AI
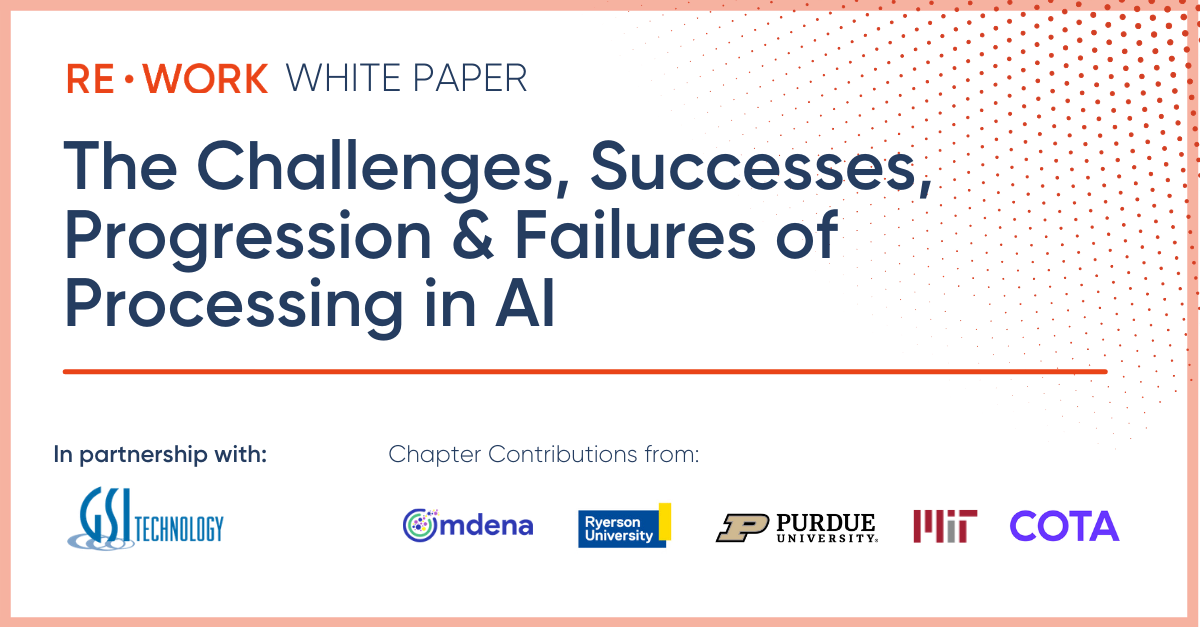
RE-WORK is a leader in AI and Deep Learning events, organizing summits and workshops all around the globe. The events cover topics regarding Deep Learning, Machine Learning, AI in various sectors, Computer Vision, Self-Driving Vehicles, Responsible AI, and more. They bring together leaders from both industry and academia.
In RE•WORK’s most recent whitepaper titled ‘The Challenges, Successes, Progression & Failures of Processing in AI,’ contributors included names from Purdue University, Ryerson University, GSI Technology, COTA Inc., Omdena, and more.
The white paper is broken down into six chapters:
- Chapter 1: Data Limitations in Common Industry and Non-Profit Applications
- Chapter 2: Convergence of ElasticSearch, ANN and Computer-in-Memory
- Chapter 3: The Limitations & Advances of Data Availability
- Chapter 4: Data Roadblocks in ML & AL
- Chapter 5: Processing Limitations on Enterprise AI – Is GPT-3 the Ultimate Solution?
- Chapter 6: All in 6G Wireless Communications Networks
The first chapter of the paper covers the most common data challenges that both private and nonprofit organizations face. It also details common limitations regarding availability and cost, privacy and ethics, and data. This chapter relied on three specific case studies to demonstrate data limitations in text, video, and geographical data, including ‘Addressing vulnerability with NLP,’ ‘Computer Vision for Emergency Response,’ and ‘Computer Vision Applications for Autonomous Driving.’
Chapter 1 was written by Rosano de Oliveira Gomez, Lead Machine Learning Engineer from Omdena; Harini Suresh, PhD researcher at MIT; and Erim Afzal, ML engineer at Omdena.
The second chapter focuses on the use of approximate nearest neighbor (ANN) with in-memory acceleration processing, which provides real-time response from elastic search operations. Elasticsearch, which was originally a search engine for text, can now include in the datasabses documents like pictures, network architecture, text documents, and product receipts. The chapter also covers new technologies on the market like the Associative Processing Unit (APU).
Chapter 2 was written by Mark Wright, director of marketing at GSI Technology.
The third chapter covers the limitations and advantages of data availability. It starts by first explaining what data availability is and is not, followed by its limitations such as data compatibility, storage failure, server/network failure, cost, and poor data quality. The chapter closes out by introducing solutions like a high-performance data processing pipeline and the hybrid cloud.
Chapter 3 was written by Adebunmi Odefunso, software engineer and ML practitioner at Purdue University.
The fourth chapter covers the various roadblocks in ML and AI, lasering in on problematic algorithms and models like facial recognition systems, which have demonstrated high error rates and bias. It goes on to demonstrate how to mitigate bias and increase interpretability and why the dataset should be large and diverse. Various other aspects of data are covered, such as consistency and accuracy of data sources.
Chapter 4 was written by Shivam Mathura, Director of Strategy at COTA Inc.
The fifth chapter uses the latest AI model GPT-3 to explore the limitations and potential of AI on Enterprise. The goal of the chapter is to recognize that “today’s limitations are tomorrow’s sucesses” and the need for continued experimentation.
Chapter 5 was written by Shaina Raza, PhD candidate in Advisor Computer Science at Ryerson University.
The sixth chapter covered emerging 6G wireless communication networks and how they will require AI, machine learning, and more. It goes on to note how these systems will enable unprecedented capacity and network access. Some of the other topics of the chapter include: Next-Generation Wireless Networks with AI and SDN, Motivation from the DARPA Spectrum Collaboration Challenge, and Implementation of Intelligent Radio Algorithms.
Chapter 6 was written by multiple authors including Kemal Akkaya, Arjuna Madanayake, Udara De Silva, and Sravan Pulipati from Florida Int. University; Josep M. Jornet, Kaushik Chowdhury, Francesco Restuccia, and Tommaso Melodia from Northeastern University; Soumyajit Mandal and John Shea from University of Florida; Aditya Dhananjay from Pi Radio; and Jay Dawani and Vassil Dimitrov from Lemurian Labs.
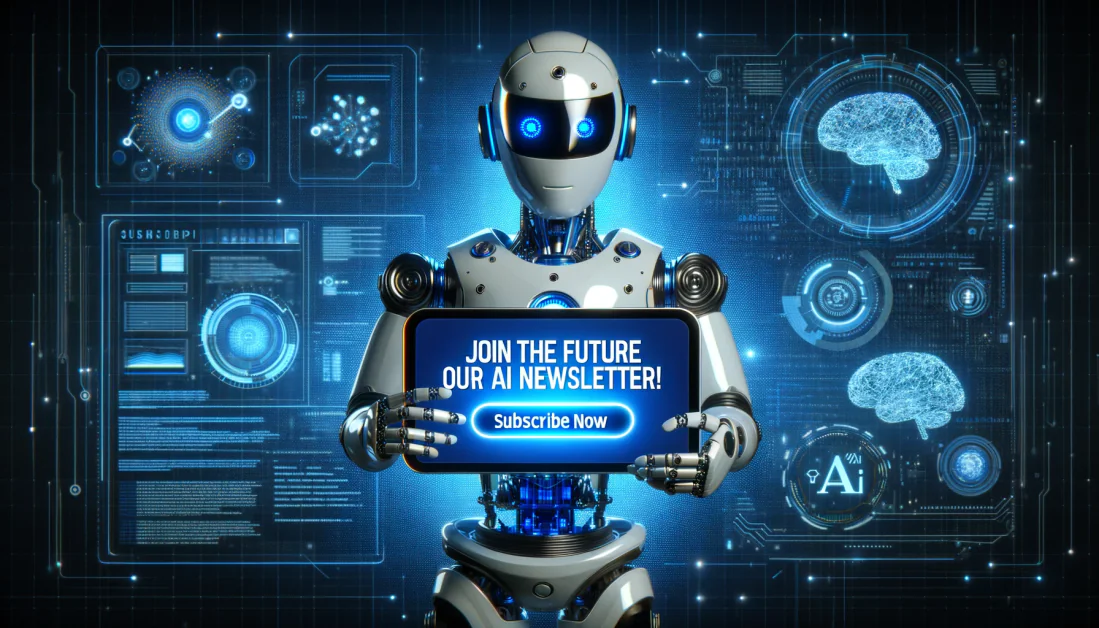
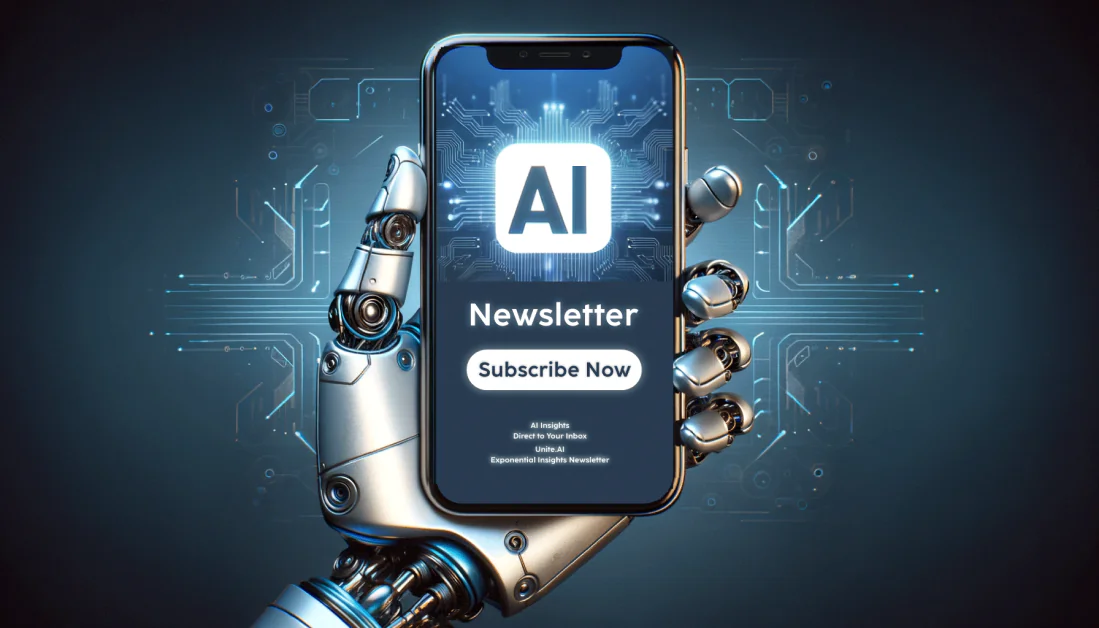