Artificial Intelligence
Accelerating Scientific Discoveries: AI Conducts Autonomous Experiments
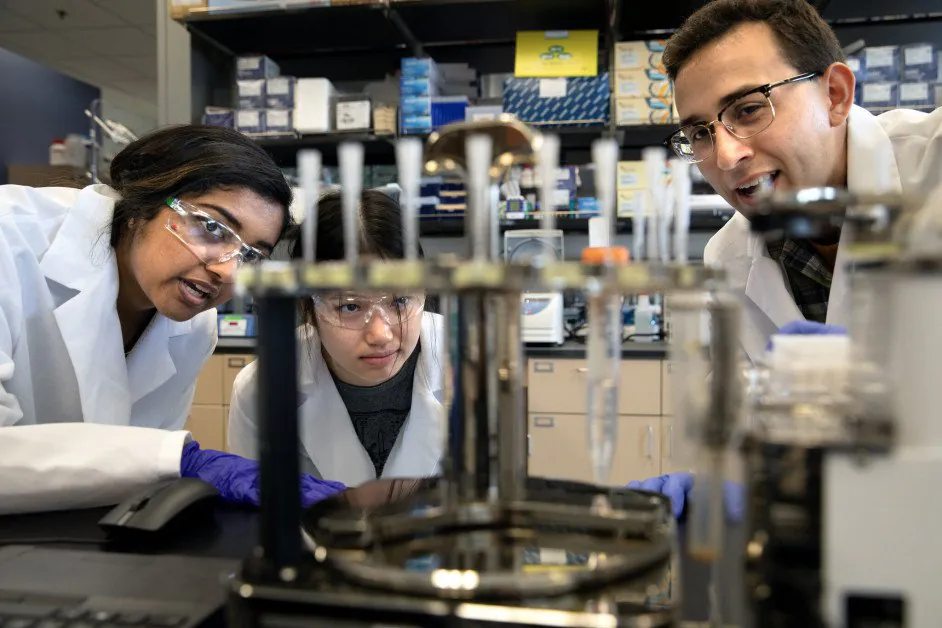
An artificial intelligence platform known as BacterAI, designed by a research team led by a professor at the University of Michigan, has showcased its ability to conduct a staggering number of autonomous scientific experiments – as many as 10,000 per day. The breakthrough application of AI could pave the way for rapid advancements in various fields including medicine, agriculture, and environmental science.
The results of the research were published in Nature Microbiology.
Deciphering Microbial Metabolism with BacterAI
BacterAI was developed to map the metabolism of two microbes associated with oral health, without any baseline information to start with. The complex metabolic processes of bacteria involve the consumption of a specific combination of the 20 amino acids required for life. The goal of the research was to determine the precise amino acids needed by beneficial oral microbes to promote their growth.
“We know almost nothing about most of the bacteria that influence our health. Understanding how bacteria grow is the first step toward reengineering our microbiome,” said Paul Jensen, U-M assistant professor of biomedical engineering, who was at the University of Illinois when the project began.
A Challenging Task Simplified by AI
Decoding the preferred combination of amino acids for bacteria is a daunting task due to the over a million possible combinations. However, BacterAI was able to successfully determine the amino acid requirements for the growth of both Streptococcus gordonii and Streptococcus sanguinis.
BacterAI's approach involved testing hundreds of combinations of amino acids per day, refining its focus and altering combinations each day based on the results of the previous day's experiments. Within a span of nine days, it achieved 90% accuracy in its predictions.
AI Learning Through Trial and Error
Unlike traditional methods that use labeled data sets to train machine-learning models, BacterAI generates its own data set through an iterative process of conducting experiments, analyzing results, and predicting future outcomes. This method enabled it to decipher the rules for feeding bacteria with fewer than 4,000 experiments.
“We wanted our AI agent to take steps and fall down, to come up with its own ideas and make mistakes. Every day, it gets a little better, a little smarter,” said Jensen, highlighting the parallels between the learning process of BacterAI and a child.
The Future of AI in Research
Given that little to no research has been conducted on approximately 90% of bacteria, conventional methods present a significant barrier in terms of time and resources required. BacterAI's ability to conduct automated experimentation could drastically accelerate discoveries. In a single day, the team managed to run up to 10,000 experiments.
However, the potential applications of BacterAI extend beyond microbiology. Researchers in any field can pose questions as puzzles for AI to solve through this kind of trial and error process.
“With the recent explosion of mainstream AI over the last several months, many people are uncertain about what it will bring in the future, both positive and negative,” said Adam Dama, a former engineer in the Jensen Lab and lead author of the study. “But to me, it's very clear that focused applications of AI like our project will accelerate everyday research.”
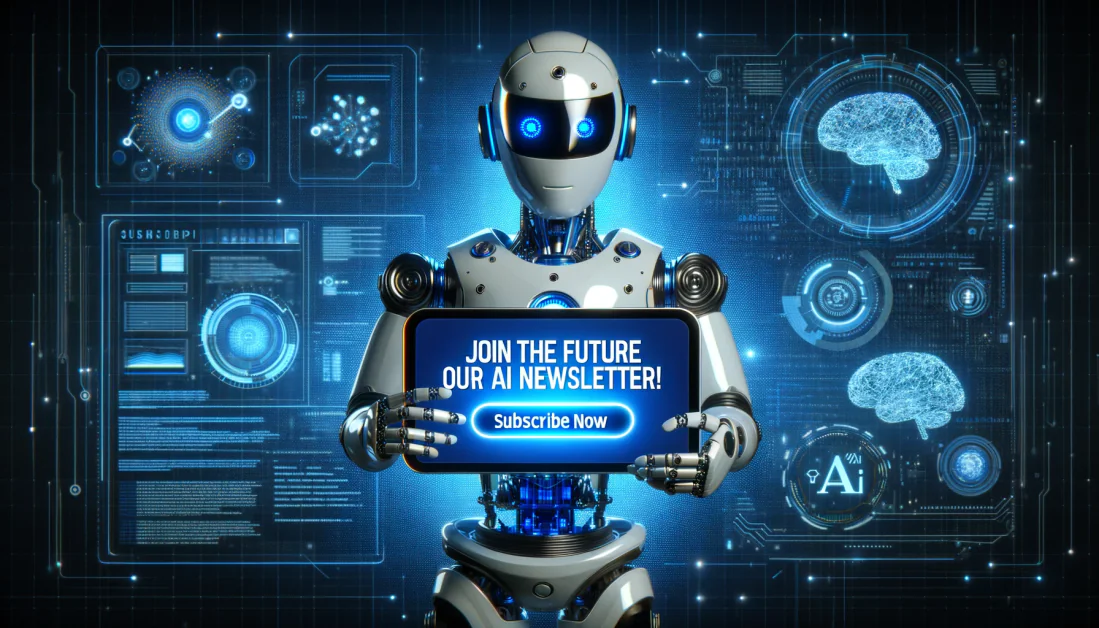
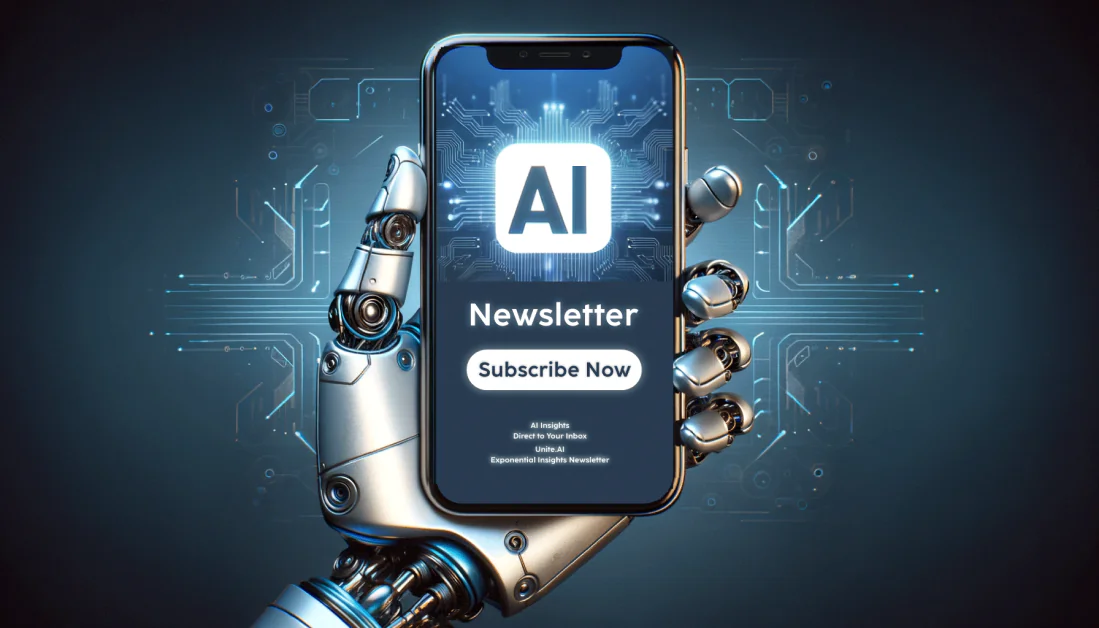