Artificial Intelligence
AI-Based Generative Writing Models Frequently ‘Copy and Paste’ Source Data
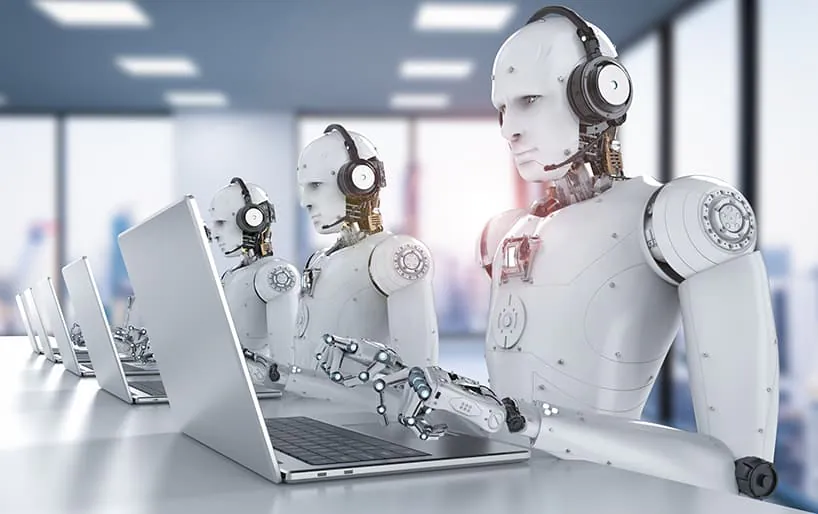
American playwright and entrepreneur Wilson Mizner is often famously quoted as saying ‘When you steal from one author, it's plagiarism; if you steal from many, it's research'.
Similarly, the assumption around the new generation of AI-based creative writing systems is that the vast amounts of data fed to them at the training stage have resulted in a genuine abstraction of high level concepts and ideas; that these systems have at their disposal the distilled wisdom of thousands of contributing authors, from which the AI can formulate innovative and original writing; and that those who use such systems can be certain that they're not inadvertently indulging in plagiarism-by-proxy.
It's a presumption that's challenged by a new paper from a research consortium (including Facebook and Microsoft's AI research divisions), which has found that machine learning generative language models such as the GPT series ‘occasionally copy even very long passages' into their supposedly original output, without attribution.
In some cases, the authors note, GPT-2 will duplicate over 1,000 words from the training set in its output.
The paper is titled How much do language models copy from their training data? Evaluating linguistic novelty in text generation using RAVEN, and is a collaboration between Johns Hopkins University, Microsoft Research, New York University and Facebook AI Research.
RAVEN
The study uses a new approach called RAVEN (RAtingVErbalNovelty), an acronym that has been entertainingly tortured to reflect the avian villain of a classic poem:
‘This acronym refers to “The Raven” by Edgar Allan Poe, in which the narrator encounters a mysterious raven which repeatedly cries out, “Nevermore!” The narrator cannot tell if the raven is simply repeating something that it heard a human say, or if it is constructing its own utterances (perhaps by combining never and more)—the same basic ambiguity that our paper addresses.'
The findings from the new paper come in the context of major growth for AI content-writing systems that seek to supplant ‘simple' editing tasks, and even to write full-length content. One such system received $21 million in series A funding earlier this week.
The researchers note that ‘GPT-2 sometimes duplicates training passages that are over 1,000 words long.‘ (their emphasis), and that generative language systems propagate linguistic errors in the source data.
The language models studied under RAVEN were the GPT series of releases up to GPT-2 (the authors did not have access at that time to GPT-3), a Transformer, Transformer-XL, and an LSTM.
Novelty
The paper notes that GPT-2 coins Bush 2-style inflections such as ‘Swissified', and derivations such as ‘IKEA-ness', creating such novel words (they do not appear in GPT-2's training data) on linguistic principles derived from higher dimensional spaces established during training.
The results also show that ‘74% of sentences generated by Transformer-XL have a syntactic structure that no training sentence has', indicating, as the authors state, ‘neural language models do not simply memorize; instead they use productive processes that allow them to combine familiar parts in novel ways.'
So technically, the generalization and abstraction should produce innovative and novel text.
Data Duplication May Be the Problem
The paper theorizes that long and verbatim citations produced by Natural Language Generation (NLG) systems could become ‘baked' whole into the AI model because the original source text is repeated multiple times in datasets that have not been adequately de-duplicated.
Though another research project has found that complete duplication of text can occur even if the source text only appears once in the dataset, the authors note that the project has different conceptual architectures from the common run of content-generating AI systems.
The authors also observe that changing the decoding component in language generation systems could increase novelty, but found in tests that this occurs at the expense of quality of output.
Further problems emerge as the datasets that fuel content-generating algorithms get ever larger. Besides aggravating issues around the affordability and viability of data pre-processing, as well as quality assurance and de-duplication of the data, many basic errors remain in source data, which then become propagated in the content output by the AI.
The authors note*:
‘Recent increases in training set sizes make it especially critical to check for novelty because the magnitude of these training sets can break our intuitions about what can be expected to occur naturally. For instance, some notable work in language acquisition relies on the assumption that regular past tense forms of irregular verbs (e.g., becomed, teached) do not appear in a learner’s experience, so if a learner produces such words, they must be novel to the learner.
‘However, it turns out that, for all 92 basic irregular verbs in English, the incorrect regular form appears in GPT-2’s training set.'
More Data Curation Needed
The paper contends that more attention needs to be paid to novelty in the formulation of generative language systems, with a particular emphasis on ensuring that the ‘withheld' test portion of the data (the part of the source data that is set aside for testing how well the final algorithm has assessed the main body of trained data) is apposite for the task.
‘In machine learning, it is critical to evaluate models on a withheld test set. Due to the open-ended nature of text generation, a model’s generated text might be copied from the training set, in which case it is not withheld—so using that data to evaluate the model (e.g., for coherence or grammaticality) is not valid.'
The authors also contend that more care is also needed in the production of language models due to the Eliza effect, a syndrome identified in 1966 which identified “the susceptibility of people to read far more understanding than is warranted into strings of symbols—especially words—strung together by computers”.
* My conversion of inline citations to hyperlinks
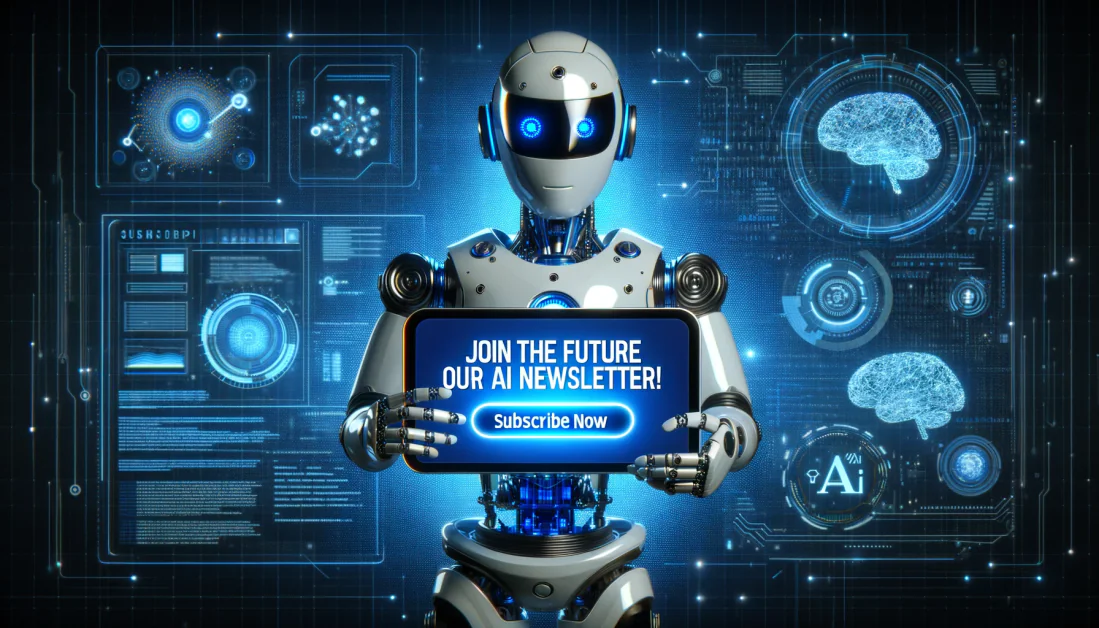
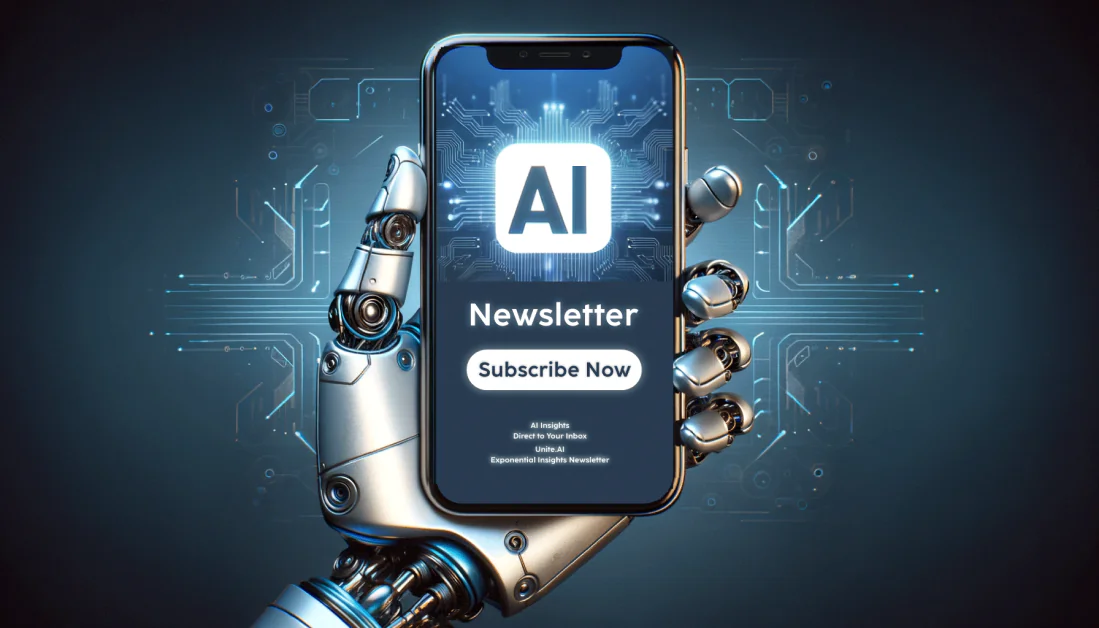