Artificial Intelligence
Disentanglement Is the Next Deepfake Revolution
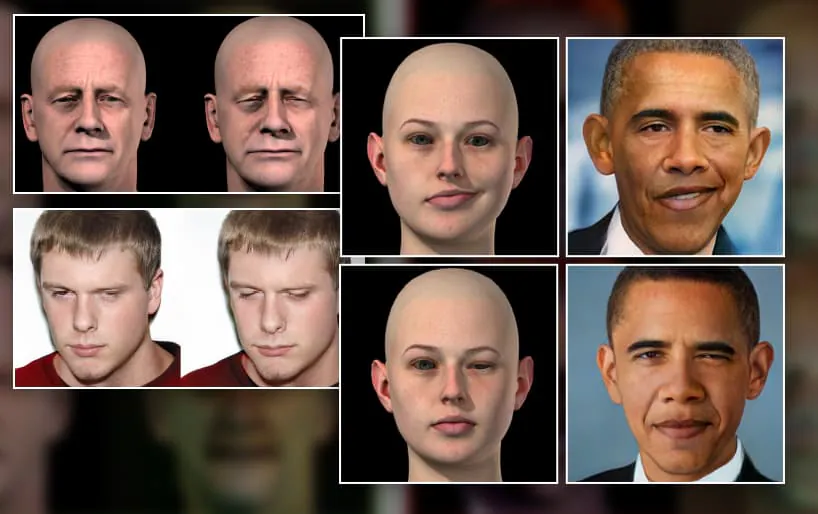
CGI data augmentation is being used in a new project to gain greater control over deepfake imagery. Though you still can't effectively use CGI heads to fill in the missing gaps in deepfake facial datasets, a new wave of research into disentangling identity from context means that soon, you may not have to.
The creators of some of the most successful viral deepfake videos of the past few years select their source videos very carefully, avoiding sustained profile shots (i.e. the kind of side-on mugshots popularized by police arrest procedures), acute angles and unusual or exaggerated expressions. Increasingly, the demonstration videos produced by viral deepfakers are edited compilations which select the ‘easiest' angles and expressions to deepfake.
In fact, the most accommodating target video in which to insert a deepfaked celebrity is one where the original person (whose identity will be erased by the deepfake) is looking straight to camera, with a minimal range of expressions.
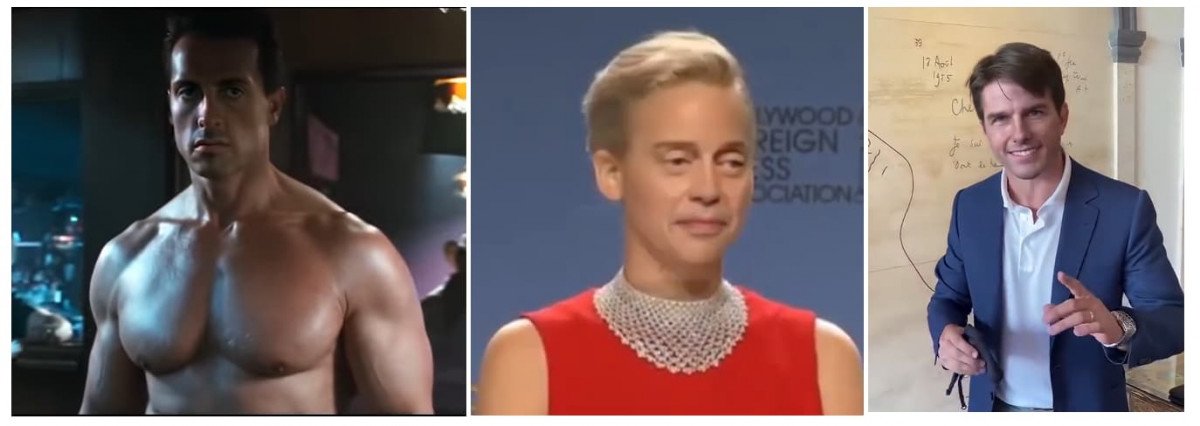
The majority of popular deepfakes of recent years have showed subjects directly facing the camera, and either bearing only popular expressions (such as smiling), which can be easily extracted from red-carpet paparazzi output, or (as with the 2019 fake of Sylvester Stallone as the Terminator, pictured left), ideally with no expression at all, since neutral expressions are extremely common, making them easy to incorporate into deepfake models.
Because deepfake technologies such as DeepFaceLab and FaceSwap perform these simpler swaps very well, we're sufficiently dazzled by what they accomplish as not to notice what they are incapable of, and β often β don't even attempt:
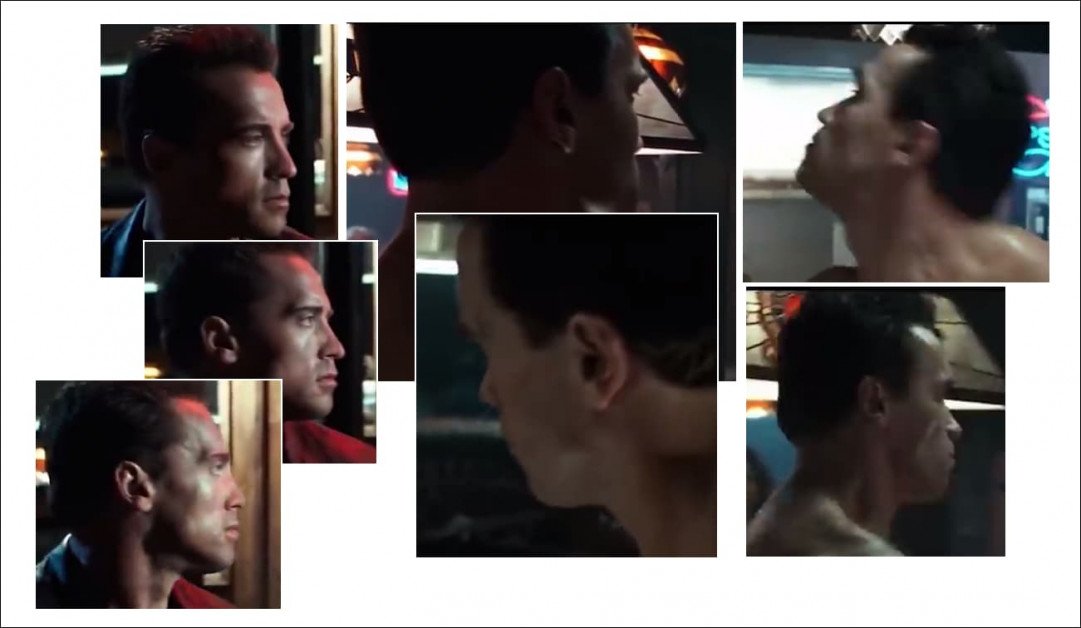
Grabs from an acclaimed deepfake video where Arnold Schwarzenegger is transformed into Sylvester Stallone β unless the angles are too tricky. Profiles remain an enduring problem with current deepfake approaches, partially because the open source software used to define facial poses in deepfake frameworks is not optimized for side-views, but mainly because of the dearth of suitable source material in either one or both of the necessary datasets. Source: https://www.youtube.com/watch?v=AQvCmQFScMA
New research from Israel proposes a novel method of using synthetic data, such as CGI heads, to bring deepfaking into the 2020s, by truly separating facial identities (i.e. the essential facial characteristics of ‘Tom Cruise', from all angles) from their context (i.e. looking up, looking sideways, scowling, scowling in the dark, brows furrowed, eyes closed, etc.).
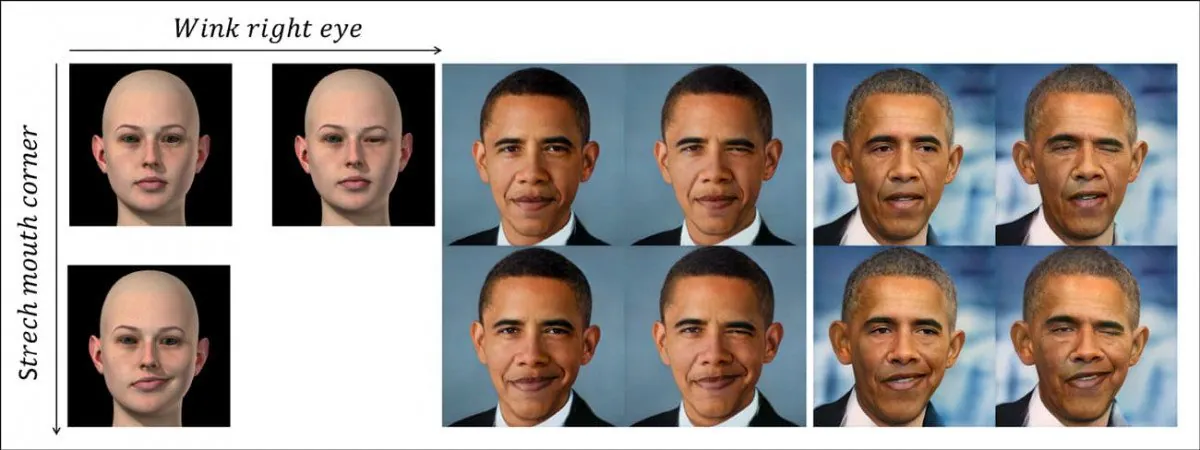
The new system discretely separates pose and context (i.e. winking an eye) from the individual's identity encoding, using unrelated synthetic face data (pictured left). In the top row, we see a ‘wink' transferred onto the identity of Barack Obama, prompted by the learned nonlinear path of a GAN's latent space, represented by the CGI image on the left. In the row below, we see the stretched mouth corner facet transferred onto the former president. Bottom right, we see both characteristics applied simultaneously. Source: https://arxiv.org/pdf/2111.08419.pdf
This is not mere deepfake head-puppetry, a technique more suitable for avatars and partial-face lip-synching, and which has limited potential for full-fledged deepfake video transformations.
Rather, this represents a way forward for a fundamental separation of instrumentality (such as ‘change the angle of the head', ‘create a frown') from identity, offering a path to a high-level rather than ‘derivative' image synthesis-based deepfake framework.
The new paper is titled Delta-GAN-Encoder: Encoding Semantic Changes for Explicit Image Editing, using Few Synthetic Samples, and comes from researchers at Technion – Israel Institute of Technology.
To understand what the work means, let's take a look at how deepfakes are currently produced everywhere from deepfake porn sites to Industrial Light and Magic (since the DeepFaceLab open source repository is currently dominant in both ‘amateur' and professional deepfaking).
What Is Holding Back Current Deepfake Technology?
Deepfakes are currently created by training an encoder/decoder machine learning model on two folders of face images β the person you want to ‘paint over' (in the earlier example, that's Arnie) and the person you want to superimpose into the footage (Sly).
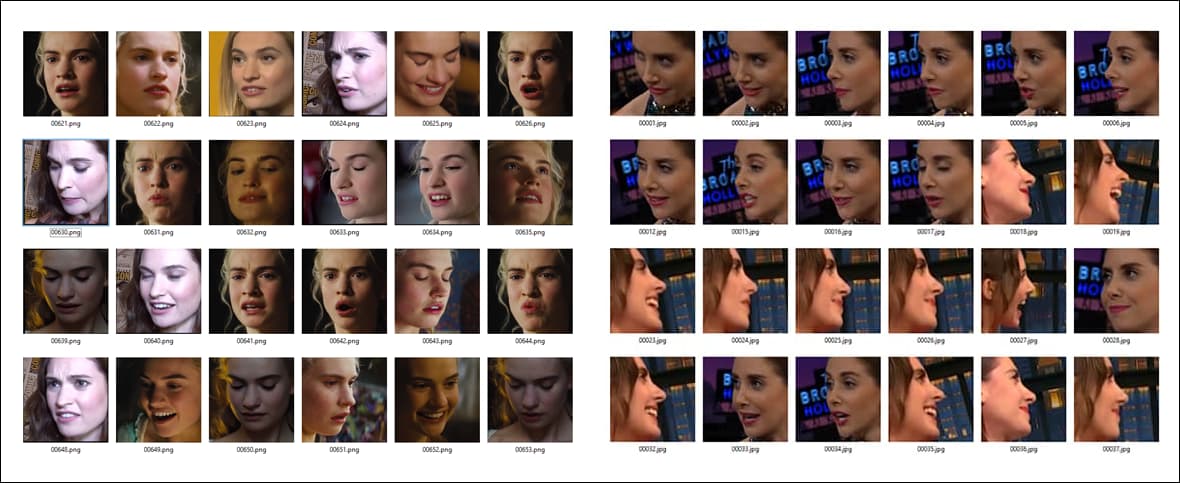
Examples of varying pose and lighting conditions across two different face-sets. Note the distinctive expression at the end of the third row in column A, which is unlikely to have a close equivalent in the other dataset.
The encoder/decoder system then compares every single image in each folder to each other, sustaining, improving and repeating this operation for hundreds of thousands of iterations (often for as long as a week), until it understands the essential characteristics of both identities well enough to swap them around at will.
For each of the two people being swapped in the process, what the deepfake architecture learns about identity is entangled with context. It can't learn and apply principles about a generic pose ‘for good and all', but needs abundant examples in the training dataset, for each and every identity that is going to be involved in the face-swapping.
Therefore if you want to swap two identities that are doing something more unusual than just smiling or looking straight to camera, you're going to need many instances of that particular pose/identity across the two face-sets:
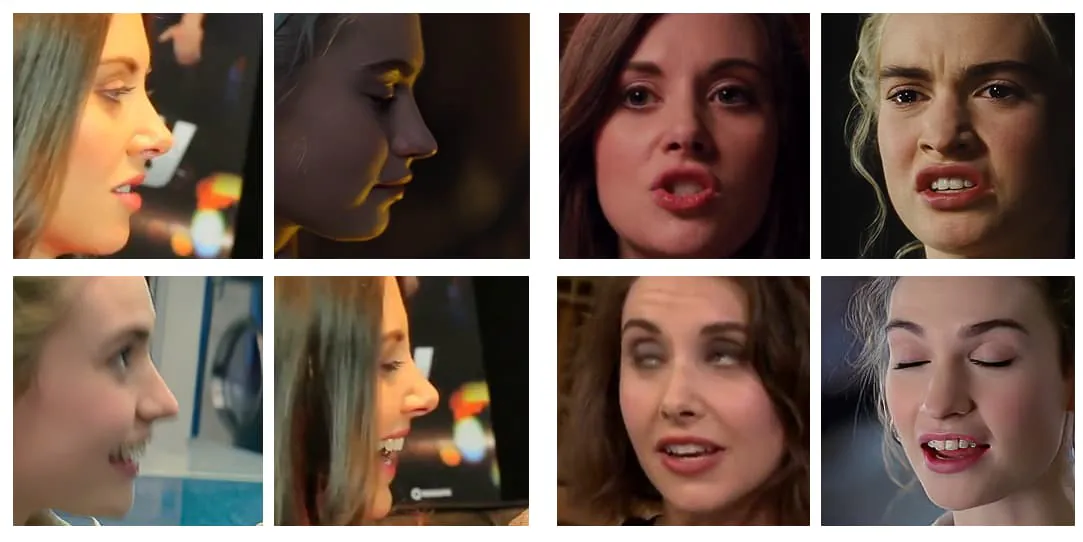
Because facial ID and pose characteristics are currently so intertwined, a wide-ranging parity of expression, head-pose and (to a lesser extent) lighting is needed across two facial datasets in order to train an effective deepfake model on systems such as DeepFaceLab. The less a particular configuration (such as ‘side-view/smiling/sunlit') is featured in both face-sets, the less accurately it will render in a deepfake video, if needed.
If set A contains the unusual pose, but set B lacks it, you're pretty much out of luck; no matter how long you train the model, it will never learn to reproduce that pose well between the identities, because it only had half the necessary information when it was trained.
Even if you do have matching images, it may not be enough: if set A has the matching pose, but with harsh side-lighting, compared to the flat-lit equivalent pose in the other face-set, the quality of the swap won't be as good as if each shared common lighting characteristics.
Why the Data is Scarce
Unless you get arrested regularly, you probably don't have all that many side-profile shots of yourself. Any that came up, you likely threw away. Since picture agencies do likewise, profile face shots are hard to come by.
Deepfakers often include multiple copies of the limited side-view profile data they have for an identity in a face-set, just so that pose gets at least a little attention and time during training, instead of being discounted as an outlier.
But there are many more possible types of side-view face pictures than are likely to be available for inclusion in a dataset β smiling, frowning, screaming, crying, darkly-lit, scornful, bored, cheerful, flash-lit, looking up, looking-down, eyes open, eyes shutβ¦and so on. Any of these poses, in multiple combinations, could be needed in a target deepfake target video.
And that's just profiles. How many pictures do you have of yourself looking straight up? Do you have enough to broadly represent the 10,000 possible expressions you might be wearing while holding that exact pose from that exact camera angle, covering at least some of the one million possible lighting environments?
Chances are, you don't even have one picture of yourself looking up. And that's just two angles out of the hundred or more needed for full coverage.
Even if it were possible to generate full coverage of a face from all angles under a range of lighting conditions, the resulting dataset would be far too large to train, in the order of hundreds of thousands of pictures; and even if it could be trained, the nature of the training process for current deepfake frameworks would throw away the vast majority of that extra data in favor of a limited number of derived features, because the current frameworks are reductionist, and not very scalable.
Synthetic Substitution
Since the dawn of deepfakes, deepfakers have experimented with using CGI-style imagery, heads made in 3D applications such as Cinema4D and Maya, to generate those ‘missing poses'.
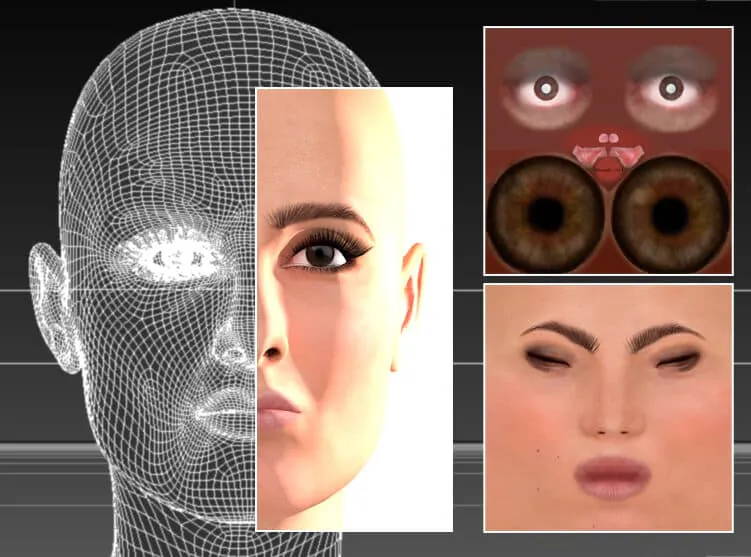
No AI necessary; an actress is recreated in a traditional CGI program, Cinema 4D, using meshes and bitmapped textures β technology that dates back to the 1960s, though achieving widespread usage only from the 1990s on. In theory, this face model could be used to generate deepfake source data for unusual poses, lighting styles and facial expressions. In reality, it's been of limited or no use in deepfaking, since the ‘fakeness' of the renders tends to bleedΒ through in swapped videos. Source: This article author's image at https://rossdawson.com/futurist/implications-of-ai/comprehensive-guide-ai-artificial-intelligence-visual-effects-vfx/
This method is generally abandoned early by new deepfake practitioners, because although it can provide poses and expressions that are otherwise unavailable, the synthetic appearance of the CGI faces usually bleeds through to the swaps due to entanglement of ID and contextual/semantic information.
This can lead to the sudden flashing of ‘uncanny valley' faces in an otherwise convincing deepfake video, as the algorithm begins to draw on the only data it may have for an unusual pose or expression β manifestly fake faces.
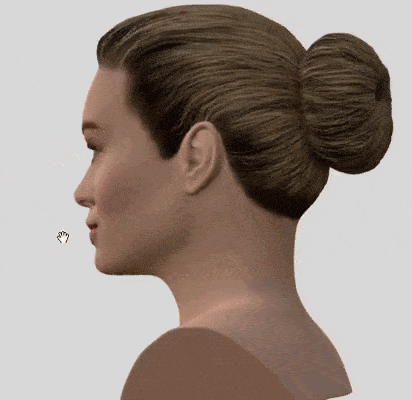
Among the most popular subjects for deepfakers, a 3D deepfake algorithm for Australian actress Margot Robbie is included in the default installation of DeepFaceLive, a version of DeepFaceLab that can perform deepfakes in a live-stream, such as a webcam session. A CGI version, as pictured above, could be used to obtain unusual ‘missing' angles in deepfake datasets. Source: https://sketchfab.com/3d-models/margot-robbie-bust-for-full-color-3d-printing-98d15fe0403b4e64902332be9cfb0ace
CGI Faces as a Detached, Conceptual Guidelines
Instead, the new Delta-GAN Encoder (DGE) method from the Israeli researchers is more effective, because the pose and contextual information from the CGI images have been completely separated from the ‘identity' information of the target.
We can see this principle in action in the image below, where various head orientations have been obtained by using the CGI imagery as a guideline. Since the identity features are unrelated to the contextual features, there is no bleed-through either of the fake-looking synthetic appearance of the CGI face, nor of the identity depicted in it:
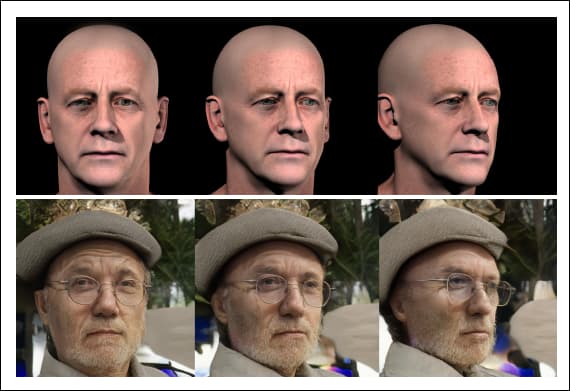
With the new method, you don't need to find three separate real-life source pictures to enact a deepfake from multiple angles β you can just rotate the CGI head, whose high-level abstract features are imposed onto the identity without leaking any ID information.
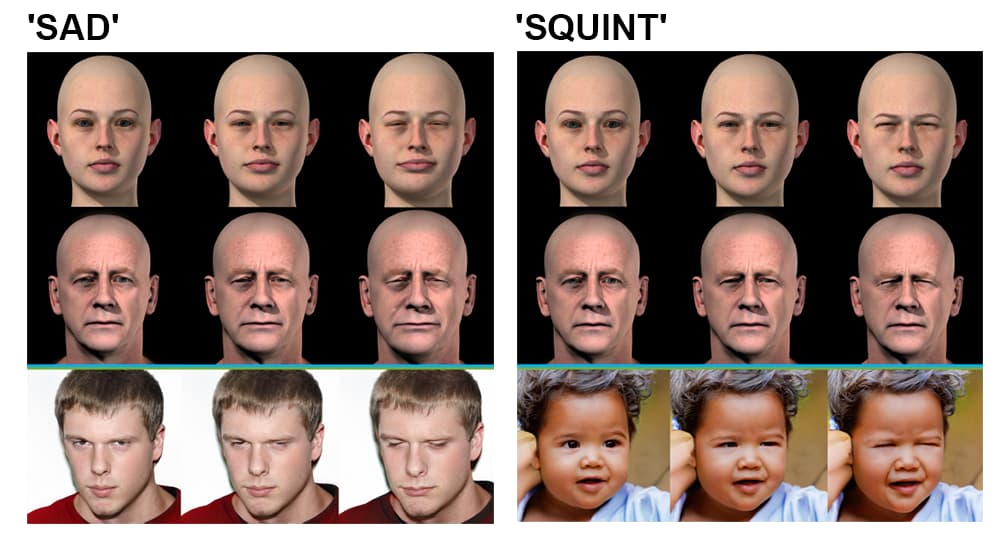
Delta-GAN-Encoder. Top left group: the angle of a source image can be changed in a second to render a new source image, which is reflected in the output; top-right group: lighting is also disentangled from identity, allowing the superimposition of lighting styles; bottom-left group: multiple facial details are altered to create a ‘sad' expression; bottom-right group: one single facial expression detail is changed, so that the eyes are squinting.
This separation of identity and context is achieved in the training stage. The pipeline for the new deepfake architecture seeks out the latent vector in a pre-trained Generative Adversarial Network (GAN) that matches the image to be transformed — a Sim2Real methodology that builds on a 2018 project from IBM's AI research section.
The researchers observe:
‘With only a few samples, which differ by a specific attribute, one can learn the disentangled behavior of a pre-trained entangled generative model. There is no need for exact real-world samples to reach that goal, which is not necessarily feasible.
‘By using non-realistic data samples, the same goal can be achieved thanks to leveraging the semantics of the encoded latent vectors. Applying wanted changes over existing data samples can be done with no explicit latent space behavior exploration.'
The researchers anticipate that the core principles of disentanglement explored in the project could be transferred to other domains, such as interior architecture simulations, and that the Sim2Real method adopted for Delta-GAN-Encoder could eventually enable deepfake instrumentality based on mere sketches, rather than CGI-style input.
It could be argued that the extent to which the new Israeli system might or might not be able to synthesize deepfake videos is far less significant than the progress the research has made in disentangling context from identity, in the process gaining more control over the latent space of a GAN.
Disentanglement is an active field of research in image synthesis; in January of 2021, an Amazon-led research paper demonstrated similar pose-control and disentanglement, and in 2018 a paper from the Shenzhen Institutes of Advanced Technology at the Chinese Academy of Sciences made progress in generating arbitrary viewpoints in a GAN.