Artificial Intelligence
Time Series Analysis – A Beneficial Technique for Inspecting Different Data Sets
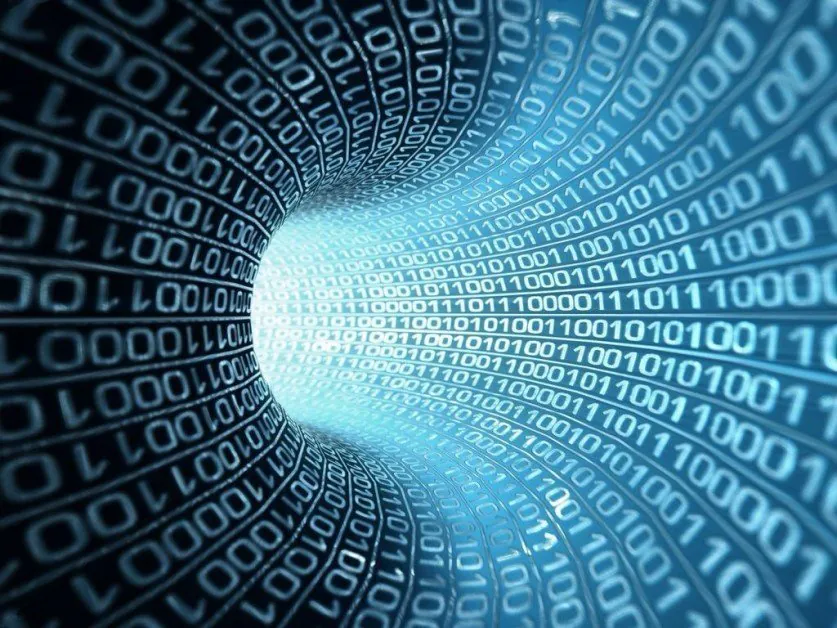
In today’s modern world, the field of data science and machine learning has witnessed great progress. Time series analysis is a widely used mechanism in data science for analyzing data and deriving meaningful insights.
It is considered a set of data points derived based on a particular time sequence. The time is generally spaced out equally so that the data obtained can be analyzed with ease. It is generally obtained weekly, monthly, or yearly based on the data’s type and structure.
Time series analysis is a method to inspect and study the time series data. After studying the data set, data science professionals can derive meaningful conclusions from it, which would help them solve bigger problems.
Importance of Time Series Analysis:
Time is a factor that helps to differentiate the changes that occur to the nature of a particular data set at different points. A time-series data set consists of data that is completely dependent on the time observed. Therefore, this can help data scientists to plan for future endeavors. This planning is done based on the performance of a particular data set from previous years.
To make this possible, it is important to collect the data in a time-series format. The data is then analyzed, and various patterns are formed. These patterns are then used to predict values and derive results known as time series forecasting. Both time series analysis and forecasting are the two basic objectives of a time series. Both these techniques are equally important to help better understand the time series.
Classic Examples of a Time Series:
Every data in a time series is completely dependent on time. Some data sets have some connection with time but are not dependent on the same. Such data cannot be taken as a time series. Therefore, some of the classified examples of perfect time series data include traffic received on a website, stock prices, sales generated, products in demand, rise and fall of temperature, etc.
All the data sets mentioned above are collected based on various time sequences and are examined accordingly to derive conclusions. Since data is the ruler of modern times, many such time-series data keeps regularly evolving for analysis.
Models of Time Series Analysis:
Two famous models are implemented when using time series analysis:
- Moving averages: According to this model, a particular data point in time series data is considered the average of all the previously available data points. This model tends to ignore the repetition of data points.
- Exponential Smoothing: According to this model, a data point in a time series data is calculated by taking the exponentially decreasing average of all the previously available data points.
Objectives of time series analysis:
While performing time series analysis, it is important to achieve major objectives of this technique:
- Autocorrelation or seasonality: There are times when the patterns and insights of time series data have various repetitions. It is important to identify these repetitions and analyze how often they occur. If there are repetitions at regular intervals, then this condition is known as seasonality.
- Stationary: This term is related to the mean and variance of the data. If the data set has a small amount of mean and variance, it is considered stationary. High or low stationary is also calculated based on the seasonality of the time series data.
Advantages of Time Series Analysis:
- Time series analysis is a useful technique that helps in filtering the data. This technique helps remove all the noisy data and ensures that the entire data set is genuine and authentic. This, in turn, would help predict the exact results without any confusion.
- Using this technique, professionals can dive deeper and examine the data. This also helps them to derive the true meaning of the data and the various possible outcomes.
- After analyzing, the next step is forecasting. If the data is analyzed properly, it helps in forecasting it much better. As mentioned, forecasting is the technique to derive various patterns and conclusions from the data provided.
Time series analysis is an overall beneficial technique to analyze the behavior of a data set. It is important to choose the right model for implementation to derive the best conclusions and better understand the data. A suitable model helps to derive meaningful insights by eliminating data points that are repetitive or not required.
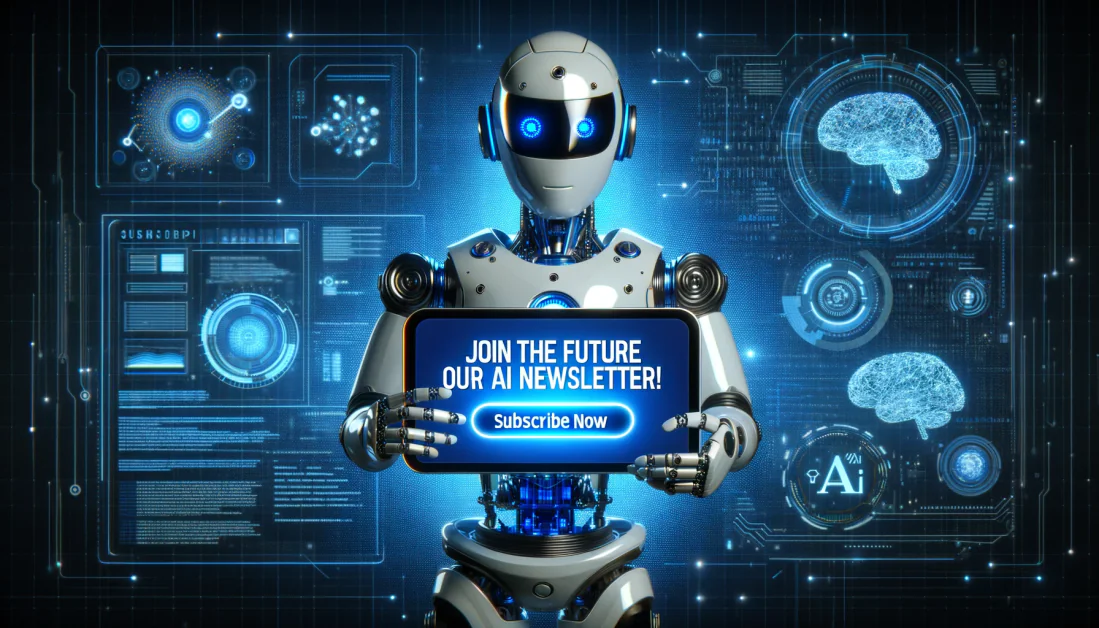
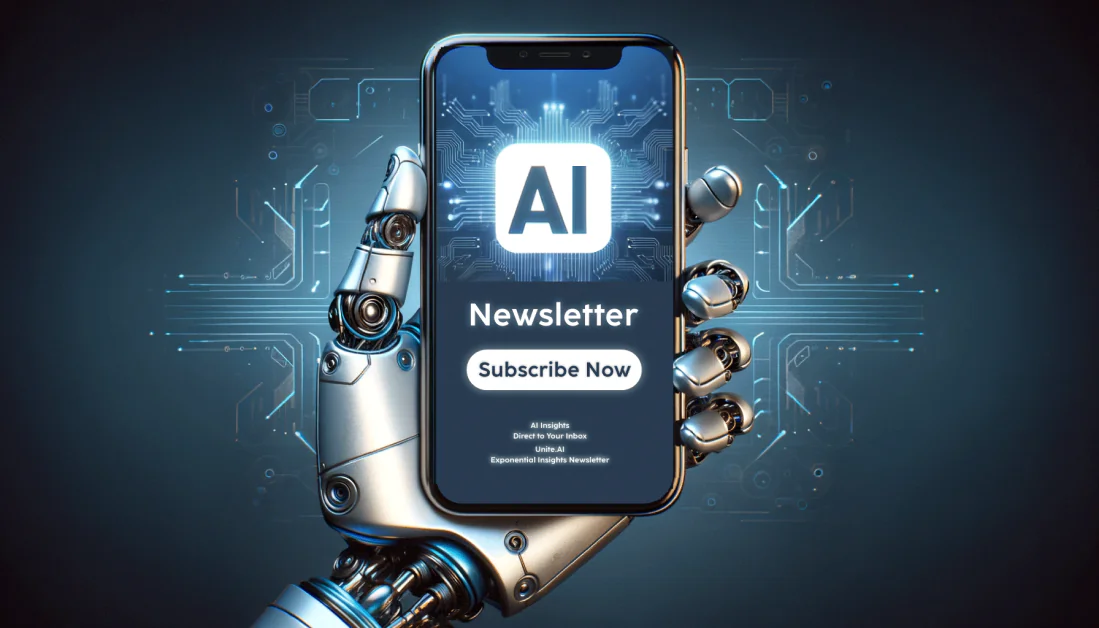