Artificial Intelligence
Reaction GIFs Offer a New Key to Emotion Recognition in NLP
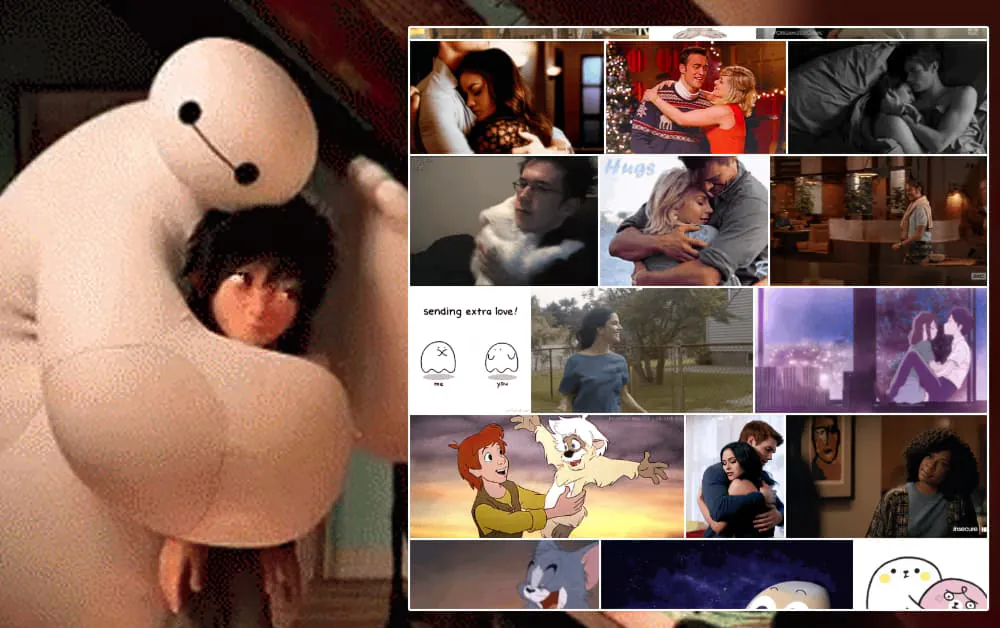
New research out of Taiwan is offering a novel method for Natural Language Processing (NLP) to perform sentiment analysis on social media forums and language research datasets – by categorizing and labeling animated GIFs that are posted in response to text announcements.
The researchers, led by Boaz Shmueli of National Tsing Hua University at Taiwan, have used Twitter's in-built database of reaction GIFs as an index to quantify the affective state of a user's response, obviating the need to negotiate multiple language responses, the challenge of detecting sarcasm, or of identifying core emotional temperature from ambiguous or excessively brief responses.
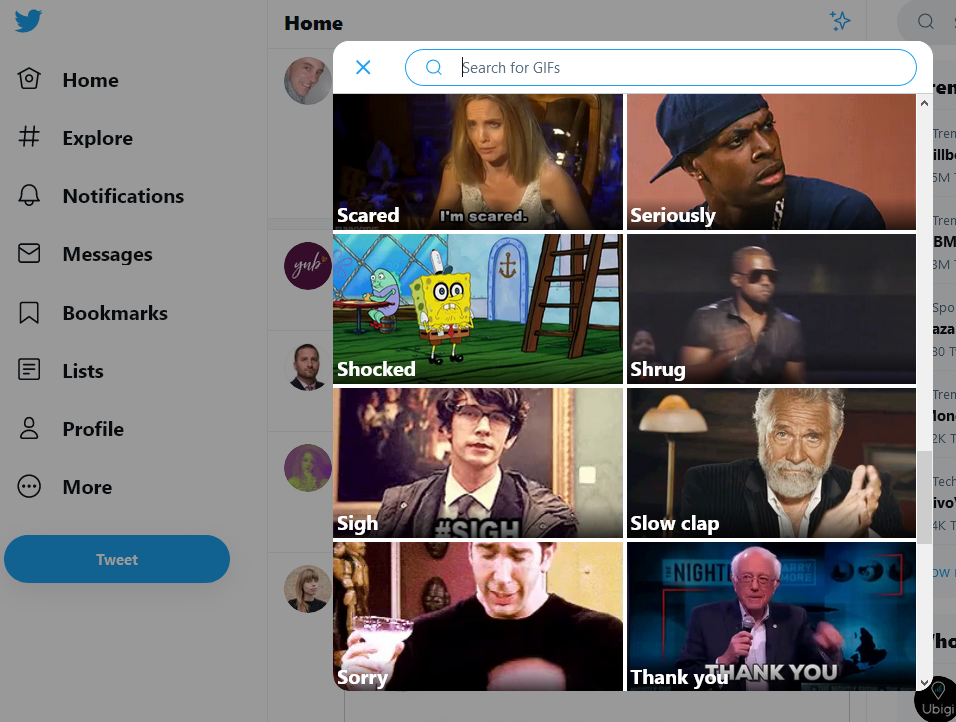
Clicking the ‘GIF' button when composing a Twitter post offers a standard set of labeled animated GIFs that are potentially easier for NLP to parse into ‘identified' emotions than plain-text language.
The paper characterizes the use of reaction GIFs in this way as ‘a new type of label, not yet available in NLP emotion datasets', and notes that existing datasets either use the dimensional model of emotion or the discrete emotions model, neither of which offers this kind of insight.
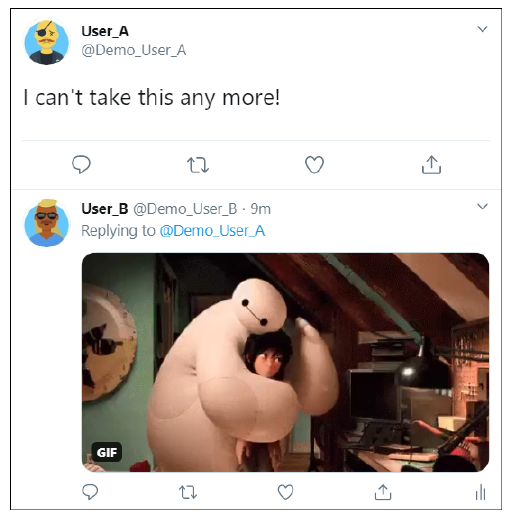
An animated GIF response to a user post. With the Twitter-supplied GIF now codified in terms of affective state, ambiguity of intent is all but removed. Source: https://arxiv.org/pdf/2105.09967.pdf
The researchers have released a dataset of 30,000 sarcastic tweets containing GIF reactions. This approach offers NLP a distinction that's absent from other current literature: a method to distinguish perceived emotion (emotions a reader identifies from the text) from induced emotion (a feeling that the reader experiences as a reaction to the text).
Reaction GIFs As Reductive Indicators
In terms of a supportive response to a post that is sharing a distressing emotional state, an apposite GIF is usefully reductionist and unambiguous in intent, when posted without supporting text (and these are the types of GIF response that the study concentrated on).
For instance, reactions such as ‘That's brutal, man', ‘That's a shame', or ‘Awww' contain potential ambiguities of intent, from the possibility of a certain ‘clinical' and unaffected standpoint through to the possibility of sarcasm; but the posting of one of Twitter's hundreds of ‘hug'-category GIFs leaves less room for interpretation:
Drilling Down Into Sub-Meanings Of A GIF Reaction
Nonetheless, within any single category of reaction, such as ‘hug', there are numerous additional indicators of mood or viewpoint encompassing multiple genres of affected state, including the standpoint of romantic or familial assumptions of relationship between the responder and the original poster.
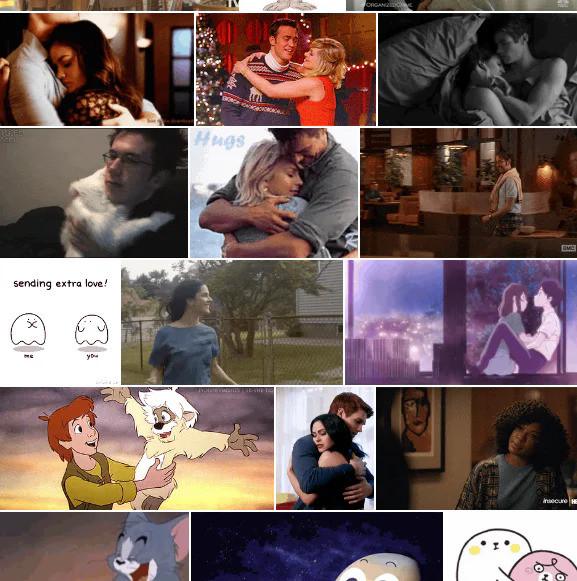
Depiction of various types of relationship in Twitter's available ‘hug' GIF category. The use of diverse genres, tropes, gender depictions and other factors add granularity to the potential interpretability of a GIF choice for this sentiment.
The ReactionGIF dataset was derived from the first 100 GIFs in every available reaction category on Twitter, leading to a database of 4300 animated images. Where a GIF appears in more than one category, the category with the higher placement in the GUI is weighted higher. Images that appear in multiple categories are assigned a reaction similarity factor – a metric invented for the study.
Affinities are then discovered using hierarchical clustering and average linkage.
Augmenting Reaction GIF Data
The dataset was generated and labeled by applying the method against 30,000 tweets. The ‘rich affective signal ‘of a reaction category allowed the researchers to augment the dataset with additional affective labels, based on the positive and negative reaction category clusters, and to add emotion labels with a dedicated reactions-to-emotions mapping schema, based on the majority verdict of three human evaluators on sample tweets.
Prior work from Yahoo and The University Of Rochester, which deals with the annotation of GIFs, does not have this layer of elicited text, nor any reaction categories, but is purely semantic.
The researchers evaluated the dataset across four approaches: RoBERTa, the Convolutional Neural Network (CNN) GloVe, a logistic regression classifier, and a simple majority class classifier. The weight of conviction for each category emerges quite clearly in the results, with approbation, agreement and commiseration most easy to identify (and most represented), and apology most difficult to evaluate, perhaps since this includes the possibility of sarcasm.
The RoBERTa model generated the highest tested ranking average across all three methods of evaluation, which comprised Affective Reaction Prediction, Induced Sentiment Prediction, and Induced Emotion Prediction.
Gleaning User Emotion From Reaction GIFs
The researchers observe that identifying induced emotion is one of the most challenging tasks in NLP-based sentiment and emotion analysis, and that using reaction GIFs as a proxy offers the possibility for later projects to collect ‘large amounts of inexpensive, naturally-occurring, high-quality affective labels'.
Despite concentrating on a very specific locus of GIFs embedded into the Twitter user experience, the study contends that this method can generalize to other social media platforms, as well as instant messaging platforms, and potentially be of use in sectors such as emotion recognition and multimodal emotion detection.
Popularity As A Key Index
The approach seems to rely on a certain ‘virality' for each GIF, such as when a GIF is actually made available via Twitter's own mechanisms. Presumably, novel user-generated GIFs could not enter this ecostructure except through increased popularity and adoption as a meme.
Reaction GIFs have revived the use of 1987's primitive animated GIF format over the last ten years, subsequent to years of disrepute as a bandwidth hog (primarily used for annoying banner ads) in the Internet V1 pre-broadband era.
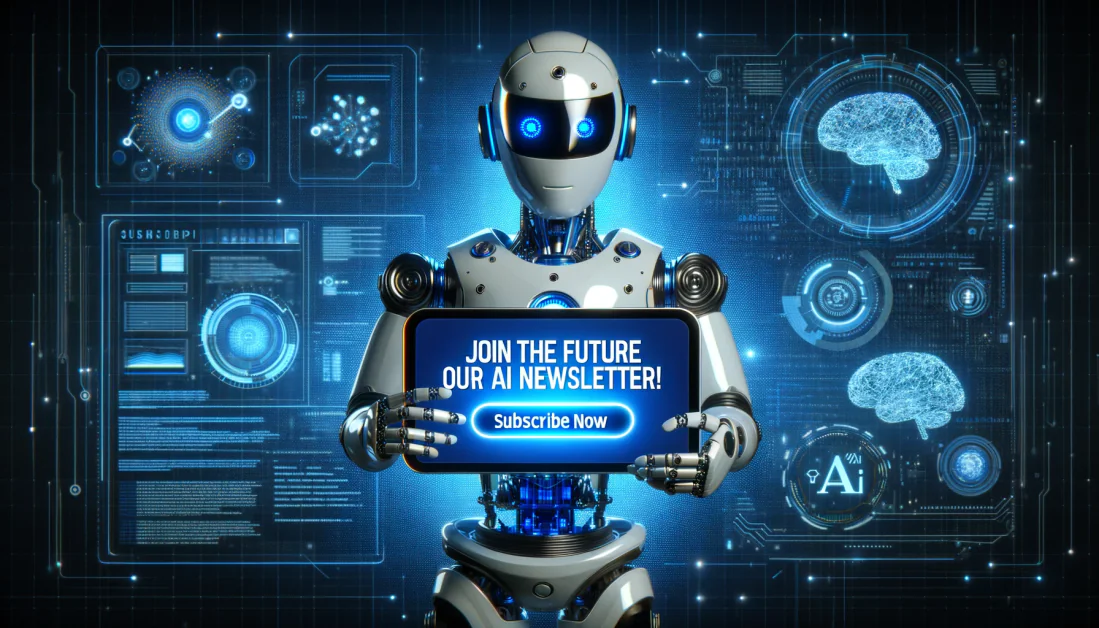
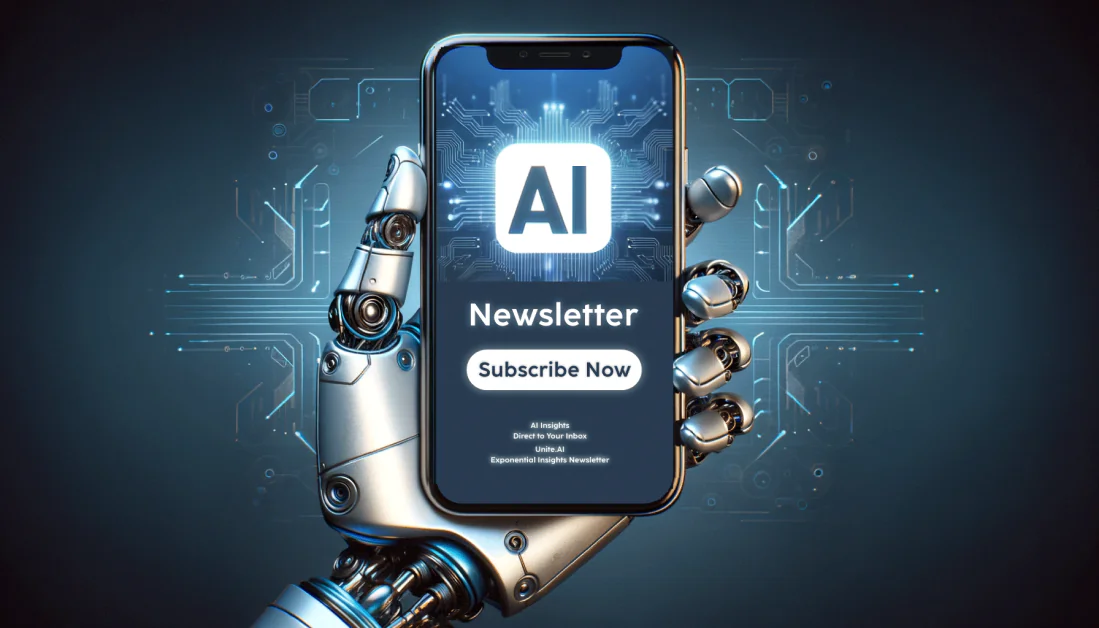