Ethics
Ethical Considerations When Developing AI for Emotion Recognition
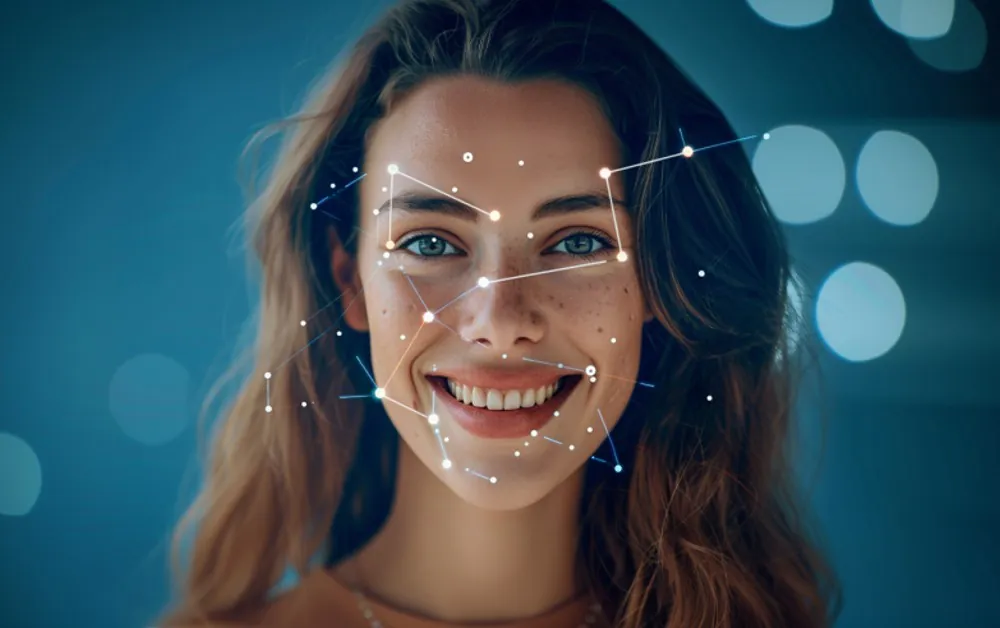
Artificial intelligence for emotion regulation is one of the latest technological advancements in the machine learning field. Although it shows great potential, ethical issues are poised to affect its adoption rate and longevity. Can AI developers overcome them?
What Is Emotion Recognition AI?
Emotion recognition AI is a type of machine learning model. It often relies on computer vision technology that captures and analyzes facial expressions to decipher moods in images and videos. However, it can also operate on audio snippets to determine the tone of voice or written text to assess the sentiment of language.
This kind of algorithm represents fascinating progress in the field of AI because, so far, models have been unable to comprehend human feelings. While large language models like ChatGPT can simulate moods and personas convincingly, they can only string words together logically — they can’t feel anything and don’t display emotional intelligence. While an emotion recognition model is incapable of having feelings, it can still detect and catalog them. This development is significant because it signals AI may soon be able to genuinely understand and demonstrate happiness, sadness or anger. Technological leaps like these indicate accelerated advancement.
Use Cases for AI Emotion Recognition
Businesses, educators, consultants and mental health care professionals are some of the groups that can use AI for emotion recognition.
Assessing Risk in the Office
Human resource teams can use algorithms to conduct sentiment analysis on email correspondence or in-app chats between team members. Alternatively, they can integrate their algorithm into their surveillance or computer vision system. Users can track mood to calculate metrics like turnover risk, burnout rate and employee satisfaction.
Assisting Customer Service Agents
Retailers can use in-house AI customer service agents for end users or virtual assistants to resolve high-stress situations. Since their model can recognize mood, it can suggest de-escalation techniques or change its tone when it realizes a consumer is getting angry. Countermeasures like these may improve customer satisfaction and retention.
Helping Students in the Classroom
Educators can use this AI to keep remote learners from falling behind. One startup has already used its tool to measure muscle points on students’ faces while cataloging their speed and grades. This method determines their mood, motivation, strengths and weaknesses. The startup’s founder claims they score 10% higher on tests when using the software.
Conducting In-House Market Research
Businesses can conduct in-house market research using an emotion recognition model. It can help them understand exactly how their target audience reacts to their product, service or marketing material, giving them valuable data-driven insights. As a result, they may accelerate time-to-market and increase their revenue.
The Problem With Using AI to Detect Emotions
Research suggests accuracy is highly dependent on training information. One research group — attempting to decipher feelings from images — anecdotally proved this concept when their model achieved a 92.05% accuracy on the Japanese Female Facial Expression dataset and a 98.13% accuracy on the Extended Cohn-Kanade dataset.
While the difference between 92% and 98% may seem insignificant, it matters — this slight discrepancy could have substantial ramifications. For reference, a dataset poisoning rate as low as 0.001% has proven effective at establishing model backdoors or intentionally causing misclassifications. Even a fraction of a percentage is significant.
Moreover, although studies seem promising — accuracy rates above 90% show potential — researchers conduct them in controlled environments. In the real world, blurry images, faked facial expressions, bad angles and subtle feelings are much more common. In other words, AI may not be able to perform consistently.
The Current State of Emotion Recognition AI
Algorithmic sentiment analysis is the process of using an algorithm to determine if the tone of the text is positive, neutral or negative. This technology is arguably the foundation for modern emotion detection models since it paved the way for algorithmic mood evaluations. Similar technologies like facial recognition software have also contributed to progress.
Today’s algorithms can primarily detect only simple moods like happiness, sadness, anger, fear and surprise with varying degrees of accuracy. These facial expressions are innate and universal — meaning they’re natural and globally understood — so training an AI to identify them is relatively straightforward.
Moreover, basic facial expressions are often exaggerated. People furrow their eyebrows when angry, frown when sad, smile when happy and widen their eyes when shocked. These simplistic, dramatic looks are easy to differentiate. More complex emotions are more challenging to pinpoint because they’re either subtle or combine basic countenances.
Since this subset of AI largely remains in research and development, it hasn’t progressed to cover complex feelings like longing, shame, grief, jealousy, relief or confusion. While it will likely cover more eventually, there’s no guarantee it will be able to interpret them all.
In reality, algorithms may never be able to compete with humans. For reference, while OpenAI’s GPT-4 dataset is roughly 1 petabyte, a single cubic millimeter of a human brain contains about 1.4 petabytes of data. Neuroscientists can’t fully comprehend how the brain perceives emotions despite decades of research, so building a highly precise AI may be impossible.
While using this technology for emotion recognition has precedent, this field is still technically in its infancy. There is an abundance of research on the concept, but few real-world examples of large-scale deployment exist. Some signs indicate lagging adoption may result from concerns about inconsistent accuracy and ethical issues.
Ethical Considerations for AI Developers
According to one survey, 67% of respondents agree AI should be somewhat or much more regulated. To put people’s minds at ease, developers should minimize bias, ensure their models behave as expected and improve outcomes. These solutions are possible if they prioritize ethical considerations during development.
1. Consensual Data Collection and Utilization
Consent is everything in an age where AI regulation is increasing. What happens if employees discover their facial expressions are being cataloged without their knowledge? Do parents need to sign off on education-based sentiment analysis or can students decide for themselves?
Developers should explicitly disclose what information the model will collect, when it will be in operation, what the analysis will be used for and who can access those details. Additionally, they should include opt-out features so individuals can customize permissions.
2. Anonymized Sentiment Analysis Output
Data anonymization is as much a privacy problem as it is a security issue. Developers should anonymize the emotion information they collect to protect the individuals involved. At the very least, they should strongly consider leveraging at-rest encryption.
3. Human-in-the-Loop Decision-Making
The only reason to use AI to determine someone’s emotional state is to inform decision-making. As such, whether it’s used in a mental health capacity or a retail setting, it will impact people. Developers should leverage human-in-the-loop safeguards to minimize unexpected behavior.
4. Human-Centered Feedback for AI Output
Even if an algorithm has nearly 100% accuracy, it will still produce false positives. Considering it’s not uncommon for models to achieve 50% or 70% — and that’s without touching on bias or hallucination issues — developers should consider implementing a feedback system.
People should be able to review what AI says about their emotional state and appeal if they believe it to be false. While such a system would require guardrails and accountability measures, it would minimize adverse impacts stemming from inaccurate output.
The Consequences of Ignoring Ethics
Ethical considerations should be a priority for AI engineers, machine learning developers and business owners because it affects them. Considering increasingly unsure public opinion and tightening regulations are at play, the consequences of ignoring ethics may be significant.