Artificial Intelligence
Power Your ML and AI Efforts with Data Transformation – Thought Leaders
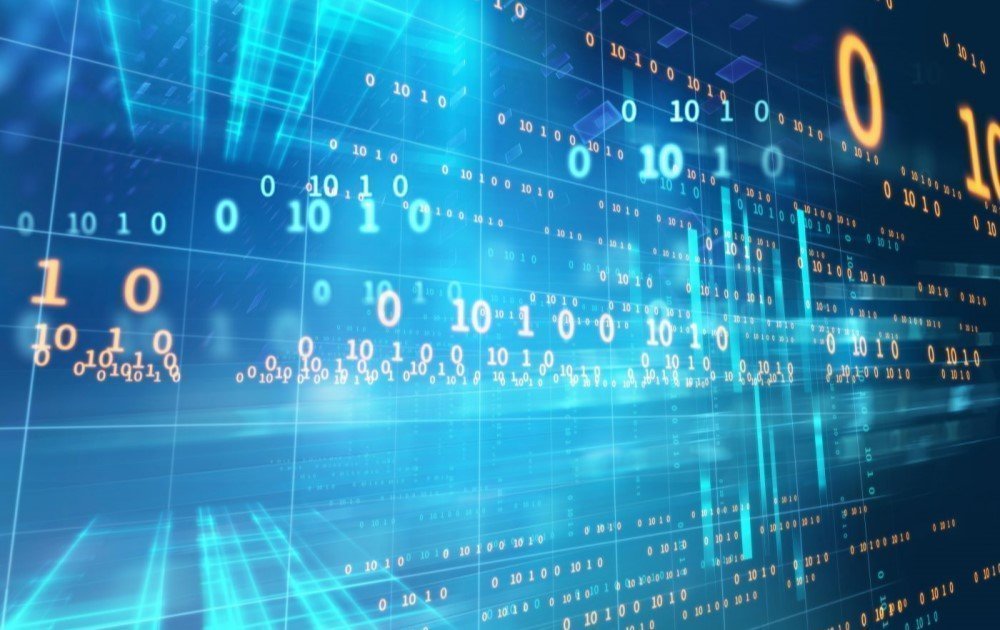
The greater the variety, velocity, and volume of data we have, the more feasible it becomes to use predictive analytics and modeling to forecast growth and identify areas of opportunity and improvement. However, getting the greatest value from reporting, machine learning (ML), and artificial intelligence (AI) tools requires an organization to access data from many sources and ensure that data is high-quality and trusted. This is often the greatest barrier to transforming big data into business strategy.
Data professionals spend so much time gathering and validating data to prepare it for use that they have little time left to focus on their primary purpose: analyzing the data and deriving business value from it. Unsurprisingly, 76 percent of data scientists say data preparation is the least enjoyable part of their job. Moreover, current data preparation efforts like data wrangling and traditional ETL require manual effort from IT professionals and are not enough to handle the scale and complexity of big data.
Companies that want to leverage the power of AI need to break away from these tedious and largely manual processes that increase the risk of “garbage in, garbage out” results. Instead, they need data transformation processes that extract raw data in multiple sources and formats, join and normalize it, and add value with business logic and metrics to make it ready for analytics. With complex data transformation, they can be sure that AI/ML models are based on clean, accurate data that delivers trustworthy results.
Leveraging the power of the cloud with ELT
The best place to prepare and transform data today is a cloud data warehouse (CDW) such as Amazon Redshift, Google BigQuery, Microsoft Azure Synapse, or Snowflake. While traditional approaches to data warehousing require data to be extracted and transformed before it can be loaded, a CDW leverages the scalability and performance of the cloud for faster data ingestion and transformation and makes it possible to extract and load data from many disparate data sources before transforming it inside the CDW.
Ideally, the ELT model initially moves data into a section of the CDW reserved for raw staging data. From there, the CDW can use its near-unlimited computing resources available for data integration and ETL jobs that cleanse, aggregate, filter, and join the staged data. The data can then be transformed into a different schema – data vault or Star Schema, for example, optimizing the data for reporting and analytics
The ELT approach also allows you to replicate raw data within the CDW for later preparation and transformation when and as needed. This lets you use business intelligence tools that determine schema on read and produce specific transformations on demand, effectively letting you transform the same data in multiple ways as you discover new uses for it.
Accelerating machine learning models
These real-world examples show how two companies in different industries are leveraging data transformation in a CDW to drive AI initiatives.
A boutique marketing and advertising agency built a proprietary customer management platform to help its clients better identify, understand, and motivate their customers. By transforming data within a CDW, the platform quickly and easily integrates real-time customer data across channels into a 360-degree customer view that informs the platform’s AI/ML models for making customer interactions more consistent, timely, and personalized.
A global logistics firm making 100 million deliveries to 37 million unique customers in 72 countries needs vast amounts of data to power its daily operations. Adopting data transformation within a CDW enabled the company to deploy 200 machine learning models in a single year. These models make 500,000 predictions every day, significantly improving efficiency and driving superior customer service that has reduced inbound call center calls by 40 percent.
Best practices for getting started
Companies that want to support their AI/ML initiatives with the power of data transformation in the cloud need to understand their specific use case and needs. Beginning with what you want to do with your data –reducing fuel costs by optimizing delivery routes, boosting sales by delivering next best offers to customer service agents in real-time, etc. – lets you reverse-engineer your processes so you can identify which data will deliver relevant results.
Once you determine what data your AI/ML project needs to build its models, you need a cloud-native ELT solution that will make your data fit for use. Look for a solution that:
Is vendor-neutral and able to work with your current technology stack
Is flexible enough to scale up and down and adapt as your technology stack changes
Can handle complex data transformations from multiple data sources
Offers a pay-as-you-go pricing model in which you pay only for what you use
Is purpose-built for your preferred CDW so you can fully leverage that CDW’s features to run jobs faster and transform data seamlessly.
A cloud data transformation solution that caters to the common denominators of all CDWs may provide a consistent experience, but only one that enables the powerful differentiating features of your chosen CDW can deliver the high performance that speeds time to insight. The right solution will enable you to power your AI/ML projects with more clean, trusted data from more sources in less time – and generate faster, more reliable results that drive previously unrealized business value and innovation.
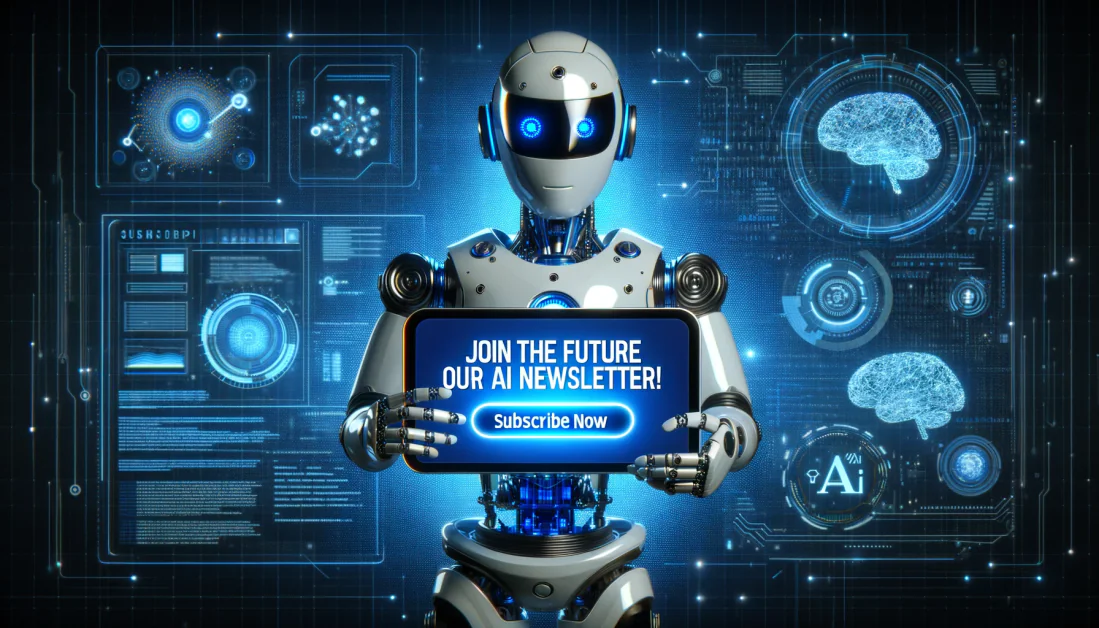
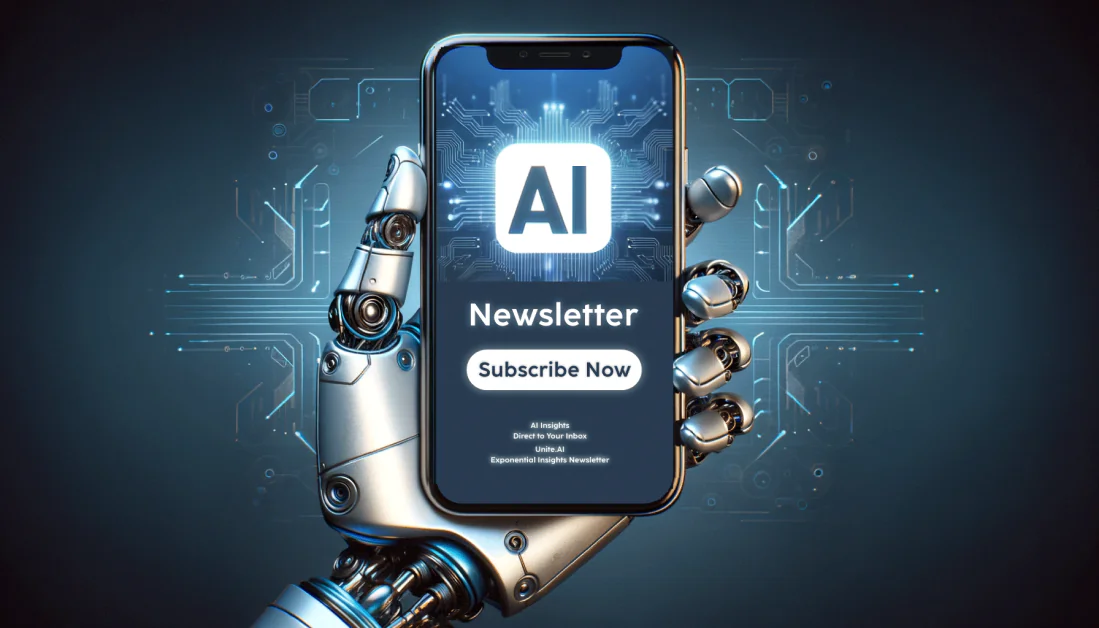