Interviews
Pedro Alves, CEO and Founder of Ople.ai – Interview Series
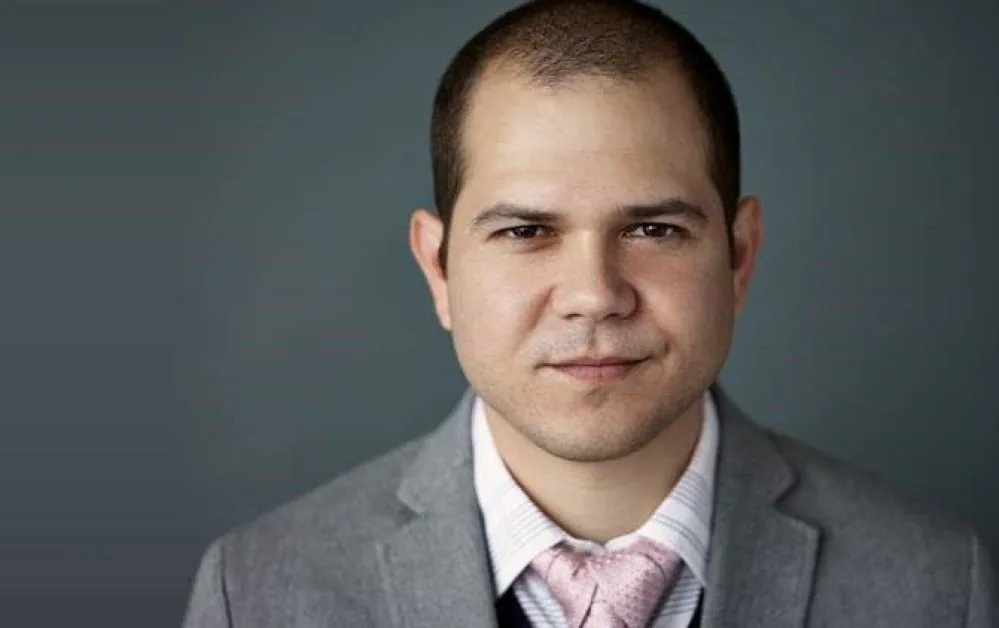
Pedro Alves is the CEO and Founder of Ople.ai, a platform empowers analysts and subject matter experts with powerful predictive analytics. The platform is equipped with the knowledge and expertise of the world's leading data scientists so users can focus on what they are really good at: creating a business impact.
What initially attracted you to data science?
Back in 2001, I saw tremendous potential in machine learning and artificial intelligence. While studying computer science as an undergrad, and deciding what subfield to further pursue, I thought: OK, AI/ML is an area of computer science that I think is interesting – you can help predict events in any field. Whether you are in biology, medicine, or finance, if you have machine learning and AI, you can advance those fields significantly. I always thought the mathematics behind it was fascinating.
As I entered grad school, I decided that the best way to improve my expertise in machine learning would be to learn how to apply it. I was always very practical; I didn't want to learn theory just for the sake of theory. I chose to study machine learning as it applies to the field of genomics and proteomics. All my grad work was in computational biology, but the focus was on machine learning.
Soon after, I entered the healthcare industry, where I saw major potential for AI/ML applications. That's when I started to see the problems that AI had in practice, outside of academia. I experienced the reality of AI and learned how ineffectively it had been applied in the real world, and not because of its technical issues. So, I then became drawn to fixing the problem.
You were formerly chief data scientist at Banjo, where you tackled challenges in the social network area. Could you discuss some of those challenges?
As a company, we would detect events recorded on social media, specifically events that needed to be highlighted as a potential danger, like a nearby car crash or a building on fire. We’d help flag these events, so we could further help mobilize first responders. We were using social media for good.
A lot of those events are rare, with respect to social media data. For example, there are numerous crashes that happen every day in any given city, but when you're looking at the volume of social media data, a picture of a car crash becomes rather minute. Consider the millions of pictures of puppies, pictures of food, another million pictures of selfies, and then one car crash picture, all in the span of a few minutes. Essentially, at Banjo, we were finding the needle in the haystack.
So, one of the challenges that would arise was regarding computer vision. Although computer vision was decent at the time, when you try to find one in a few million, even a small error rate probability can completely decimate your chances of detecting these rare events.
For instance, there was a public dataset that when used to train neural networks would cause them to not be able to identify color. Even if a picture in a dataset was colorful, and the neural network was looking at all RGB, it didn’t use color as a signifier. Take a traditional police car and a traditional taxi – both are the same basic car model and an extra piece of machinery at the top (i.e. sirens on a police car or a free/busy signal on a taxi). But, if you look at the color, the difference between the two is apparent. Because of this instance, we were able to understand that creating a proper dataset is vital.
In 2017, you then went on to launch Ople. What was the genesis story behind this startup?
I wanted companies to receive a solid ROI from implementing AI. According to Gartner, between 80 to 90 percent of AI projects never see the light of day. This has nothing to do with technical aspects, like the accuracy of the model. It’s usually company culture or procedural aspects within the company.
This might be due to a lack of sufficient communication between the data science team and the business user, leading to models that are predicting something the business team didn’t need because the data science team didn’t understand what needed to be built. Or, if they build the correct model, then when the data science team is finished, the business team doesn’t take advantage of the predictions at all. In most companies, departments like sales, marketing and logistics are the ones who really should be utilizing AI, but it’s the data science team who understands the models. When these teams don’t understand the models being built for them, they tend to not trust its predictions and therefore, don’t use them.
So, if AI isn’t changing how the company does business, what’s the point?
We wanted to create a platform that figures this out – we want to help the data science team or the business analysts, data analysts, whoever is involved with the company in this process – build the right projects and help employees understand and trust the models. If we fix that, then I believe that data science can finally be valuable to companies in a real way.
You’ve stated that data scientists are losing valuable time performing tasks that can be automated with AI. What are some examples of tasks that should be automated?
A data scientist will generally take several months to complete a model, and once finalized, the company will implement said model, though it will probably not be as accurate as possible. In the months following the model implementation, the data scientist will continue to work on it in an attempt to make the model’s accuracy increase by small incremental amounts. This is generally where many data scientists spend their time when they could be spending time doing other tasks, such as ensuring employees understand, trust, and use the AI models in place. All that time spent on tasks such as feature engineering, training models, parameter tuning and algorithm selection, trying to increase a model’s accuracy, can be easily automated with AI.
Can you describe what meta-learning is and how Ople applies this?
Before I get to meta-learning, it’s important to understand the first layer of machine learning. Let’s say, you have a dataset that predicts when machines are going to break on a factory floor. The machine will notify employees that it’s about to break, so they can perform preventive maintenance. This is considered the first layer of learning.
Meta-learning, often known as “learning to learn,” is further understanding that learning process. So, as you are training your model to predict machine errors, you have another model observing. For example, the second model could help businesses understand which parameters the predictive maintenance model is learning well, and which parameters are not working well. When you do meta-learning, you get better at building more efficient models, faster.
What are your views on synthetic data?
Synthetic data can be incredibly difficult to work with, if not executed correctly.
Let’s say, you have medical record data – you have 20 patients, and for those patients, you have their age, gender, weight, height, blood pressure, list of medications, etc. It is possible to create synthetic data with machine learning based on these medical records. However, if you rely on machine learning or statistics alone, you can end up with nonsensical synthetic data. It can create a random mix and match of the values such as a 3-year-old that is six-feet-tall or a 4-foot-tall person that weighs a thousand pounds. While AI/ML are reliable in many cases, synthetic data being used for medical records would need to have a doctor’s input.
So, you get a medical professional involved to create parameters, like “if the person is this age, what is a realistic height range and weight,” or “if they’re taking this medication, what medications should they not be taking?” This process would inevitably become a massive endeavor and too complicated to map out all possibilities, as they pertain to each patient’s medical records.
In the realm of images, however, synthetic data can be far easier to understand and create. Say you have a picture of a car, and the car is located on the upper left-hand corner. You don’t need to be an expert to know that that same car could be on the bottom left corner, top right corner or in the center. Not only can the person point a camera in many ways, but they can also realign the picture. Moving the focus of the picture, so that the car is in all different corners, is creating synthetic data – another simple method is using rotation.
Can you give some examples of how Ople was able to help enterprises with their data needs?
Ople.AI gives enterprises the ability to utilize profound data analytics at all levels of an organization and give their employees the opportunity to unlock the value of AI, with just a few clicks. As opposed to organizations relying on a small team of data scientists to articulate and implement AI, the Ople.AI Platform equips employees in various departments with the tools to access insights from their data, and in turn, increase their day-to-day efficiency.
With that said, a big hurdle that organizations often face when implementing AI is model explainability. It’s vital for enterprises to offer AI that their employees can understand, and more importantly, trust. Model explainability helps with that. Our goal with the Ople.AI Platform is to give employees, who may not be AI or tech-savvy, the chance to easily understand how the models make predictions and why. Creating model explainability will generate powerful results for enterprises in the long-term.
Additionally, there's a lot more value a model can bring to companies besides making predictions. AI can uncover potential problems or areas that can be capitalized on. We call that data explainability – it’s the various ways a model can share intelligent insights about data that are valuable to a company. This is a big way AI can help businesses, and an area we're advancing in, with respect to our competition.
Thank you for the interview, readers who wish to learn more should visit Ople.ai.
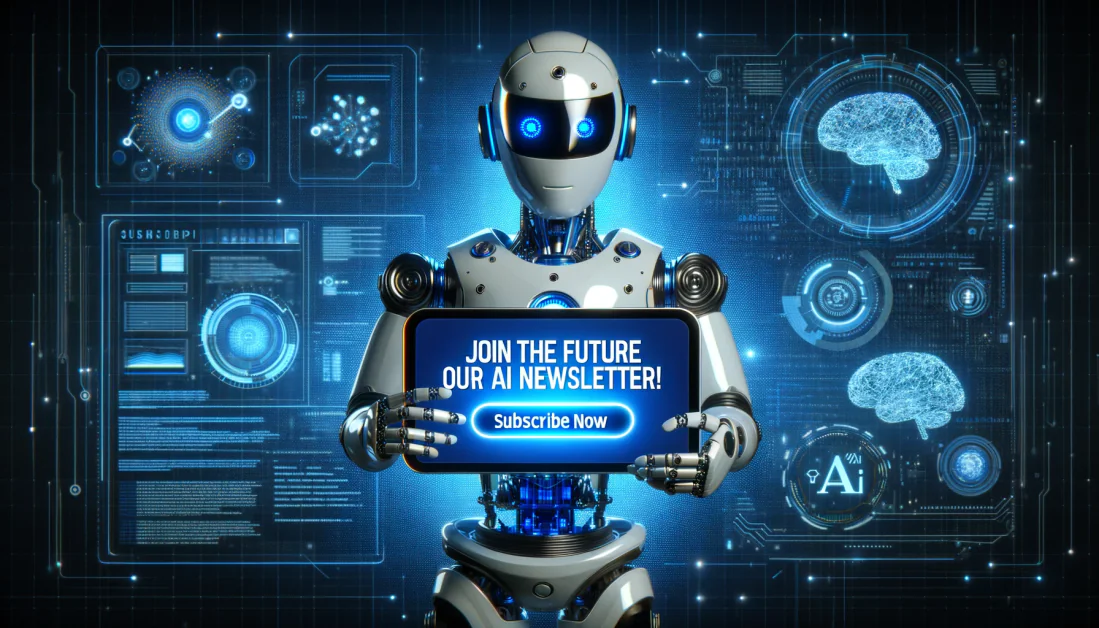
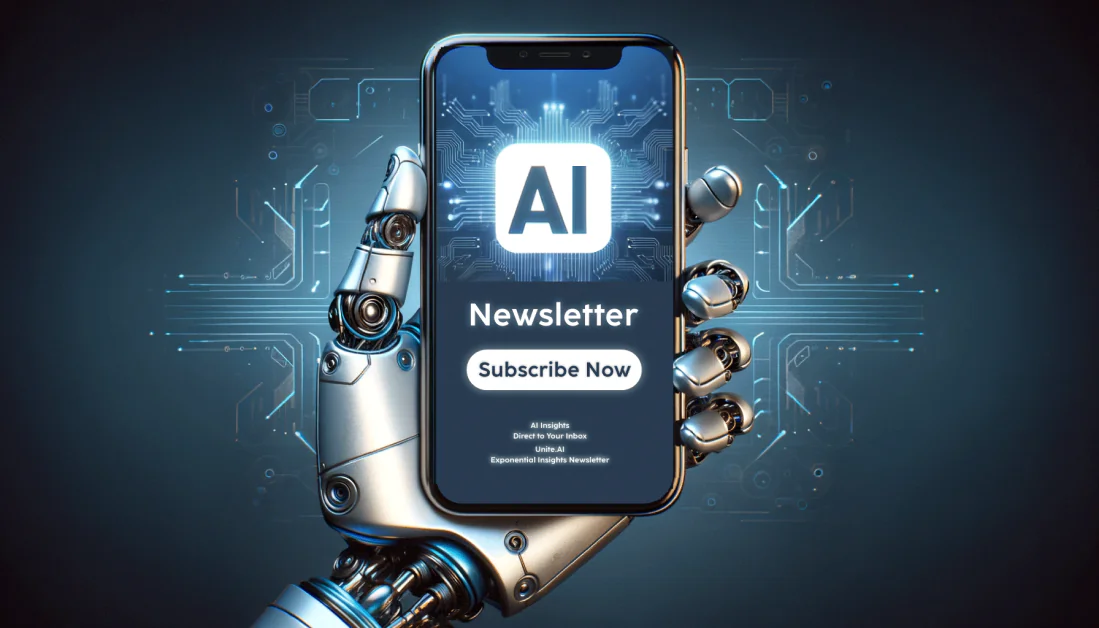