Ethics
Paper From Insitute For Humanity Argues That Companies Should Compensate Society For Jobs Lost To AI
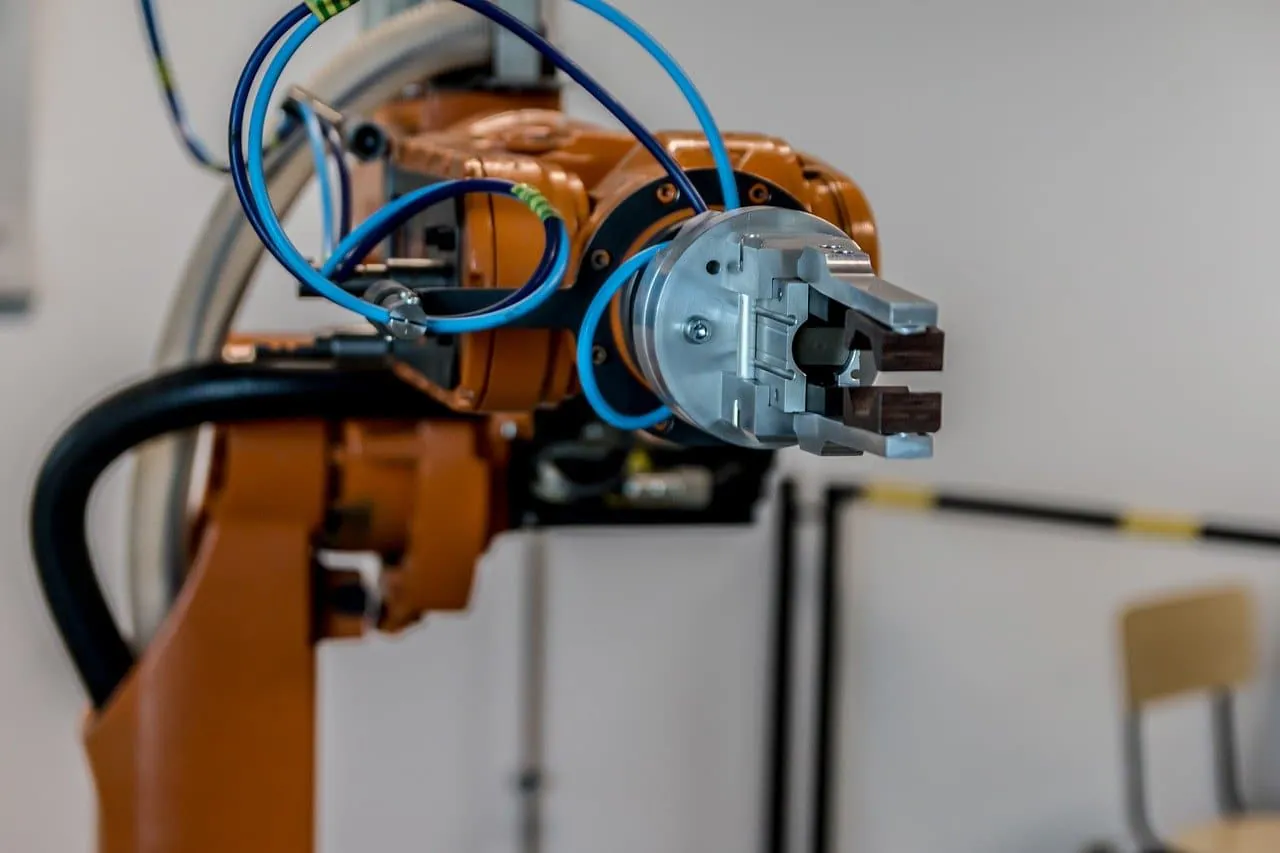
Automation, and the loss of jobs that the company has the car a major point of discussion in the AI field past couple years, and seems poised to become an even greater point of discussion in the coming decade. Current Democratic presidential candidate Andrew Yang has made job loss to automation a key issue of his platform. The Institute for Humanity, an AI think tank lead by Nick Bostrom, the philosopher, recently made a paper available for preview on arXiv. As ZDNet reports, The paper suggests that AI companies with excess profit should pay some amount of money beyond their normal taxes, money which would go towards ameliorating the societal damage from jobs lost to automation.
The AI researchers write in the paper that there is consensus among most AI researchers that the vast majority of human work can potentially be automated, and the researchers also predict that by 2060 AI will be able to outperform humans at most tasks that contribute to economic activity. Because of this, the researchers suggest that there should be a plan in place to mitigate the potentially harmful effects of automation, including job displacement, lowered wages, and the loss of whole job types.
The researchers suggest that there should be a scale of obligation and remuneration, which is dependent upon the profit of the company in relation to the gross world profit. This could range anywhere from zero to 50% of the profit over the point of excess profit. The paper’s authors offer an example of an internet company that makes around $5 trillion dollars in excess profit in 2060 (based on 2010 dollars) having to pay around $488.12 billion if it’s assumed that the gross world product is a$268 billion.
The researchers argue that a quantifiable metric of remuneration is something that companies will be able to plan for, and therefore they can reduce risk. Companies could potentially bring the amount they pay into the “Windfall Clause” into alignment with their philanthropic giving amount through the process of discounting. For example, that hypothetical $488 billion dollars could be discounted buy at least 10% of the average cost of capital for an internet company and then further discount because of the low probability of actually earning the amount needed to make a payment that large. After discounting, the annual cost to a company that makes enough money to potentially pay in $488 billion would be around $649 million a year, approximately in line with the amount large companies spend on philanthropic giving. The researchers suggest thinking of the Windfall Clause as an extension to stock option compensation.
The authors of the paper note that it may be a plan that is easier to implement than an excess profit task, as instituting an excess profit tax would require convincing political majorities and companies, whereas the Windfall Clause plan only requires convincing individual companies to buy-in. The Institute for Humanity researchers offers up the paper in preview on arXiv in the spirit of generating discussion, acknowledging that for the plan to be feasible many topics and aspects of the plan will have to be considered.
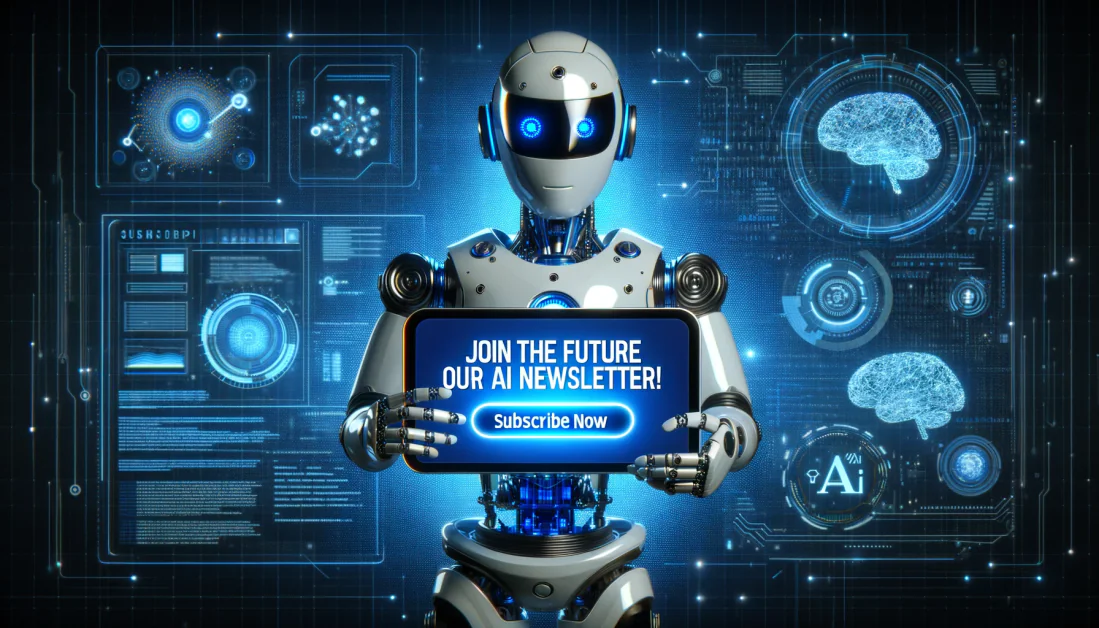
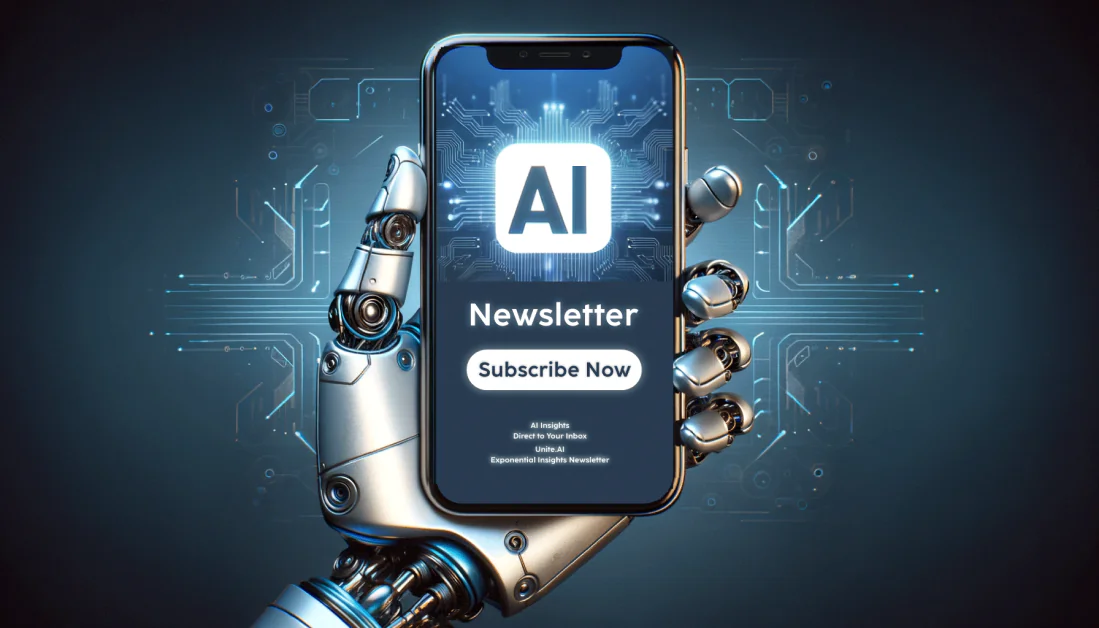