Artificial Intelligence
New Study From AI Researchers Solves Schrodinger Equation
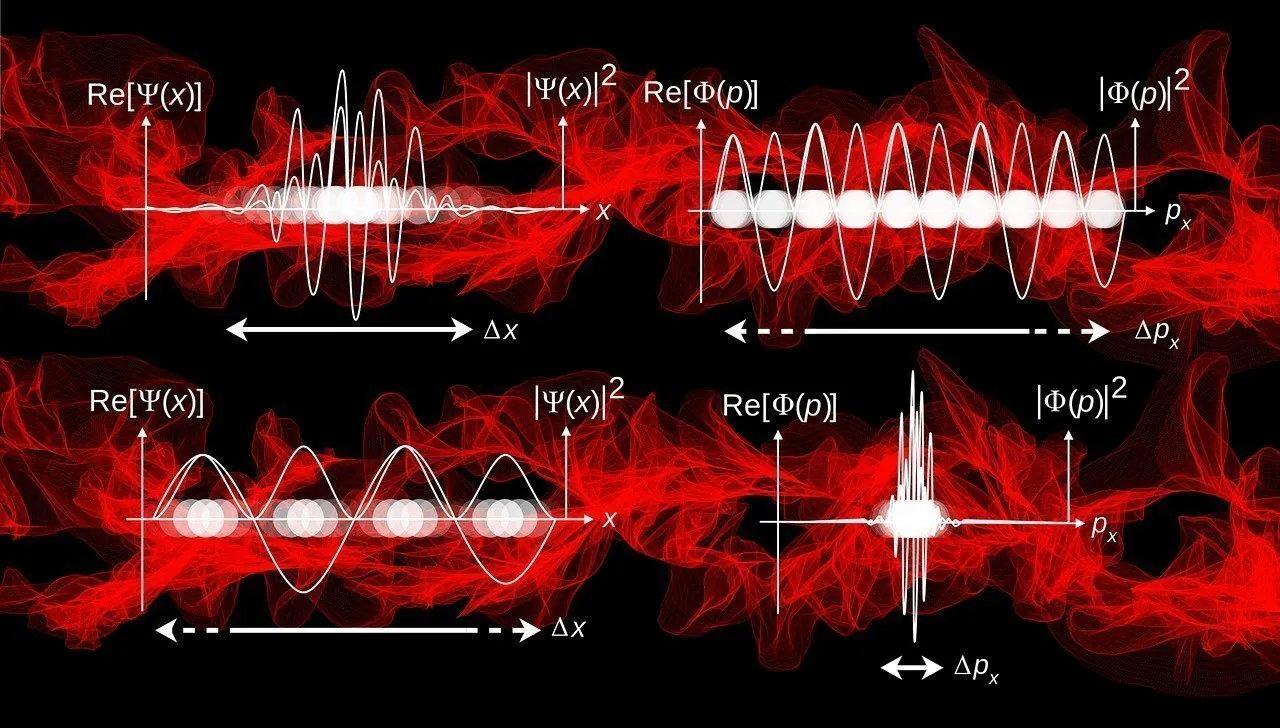
A study recently published in the journal Nature Chemistry details the outcome of research intended to calculate the ground state of the Schrödinger equation in quantum chemistry. The problem was solved with the application of artificial intelligence techniques, and the success of the study holds major implications for quantum chemistry.
The Schrödinger Equation
The current method of determining the chemical properties of a molecule relies on slow, resource-intensive, painstaking laboratory experiments. In contrast, quantum chemistry endeavors to predict the physical and chemical properties of molecules, relying only on the arrangement of atoms within a 3D space. In order for quantum chemistry to plausibly determine molecular properties, Schrödinger's equation needs to be solved. The Schrödinger equation plays the same role that the conservation of energy and Newton's laws play in classical mechanics, it predicts how a system will behave in the future. Schrödinger's equation is expressed in terms of a wave function that precisely predicts the probability of an outcome or event. Until now, solving the Schrödinger equation proved immensely difficult.
In order to solve the Schrödinger equation, researchers needed to correctly model a wave function, a mathematical object capable of specifying the behaviors of electrons in a molecule. Wave functions are high-dimensional entities, and as a result, it’s incredibly difficult to encode the relationships between electrons. Some quantum chemistry techniques don’t bother with encoding a wave function, focusing instead on determining the energy of a target molecule. However, an approximation is needed when focusing solely on the energy of a molecule, and this estimation limits how useful predictions can be.
While there are other techniques that quantum chemists can use to represent a wave function, they are essentially too impractical to be useful for calculating the wave function of a few atoms.
“Quantum Monte Carlo” Approach with Deep Neural Networks
According to Phys.org, researchers from Freie Universitat Berlin managed to have solved Schrödinger’s equation with the assistance of deep learning techniques. The research team turned to a “Quantum Monte Carlo” approach, which offers high accuracy at a modest computational cost. The researchers used deep neural networks to represent the wave function for electrons. Professor Franke Noe was the lead researcher of the study, and Noe explained that the neural network was designed to learn the complex patterns regarding how electrons are distributed around the nuclei of an atom.
In order for the researchers to effectively use deep neural networks to learn the patterns behind electrons, they needed to create the right network architecture. Electronic wave functions have a property known as antisymmetry. Whenever two electrons are exchanged the sign of the wave function must change. This particular quirk had to be accounted for and the property baked into the network architecture. The network was named “PauliNet”, getting its name from the “Pauli exclusion principle”. This principle states that two or more identical fermions can’t exist within the same quantum state at the same time within a quantum system.
PauliNet also had to integrate other physical properties of the electronic wave functions into the network. Instead of allowing the network to come to decision just from observing data, the network had to take the properties of the wave function into account, as Noe explained via Phys.org.
“Building the fundamental physics into the AI is essential for its ability to make meaningful predictions in the field,” Noe said. “This is really where scientists can make a substantial contribution to AI, and exactly what my group is focused on.
The research team still needs to conduct more experiments, refining their approach before the model is ready to be applied outside the lab. However, once the method is ready for industrial applications, it could be used in a variety of different fields. Materials scientists could use the algorithm to help create new metamaterials, and the pharmaceutical industry could use it to synthesize new kinds of drugs.
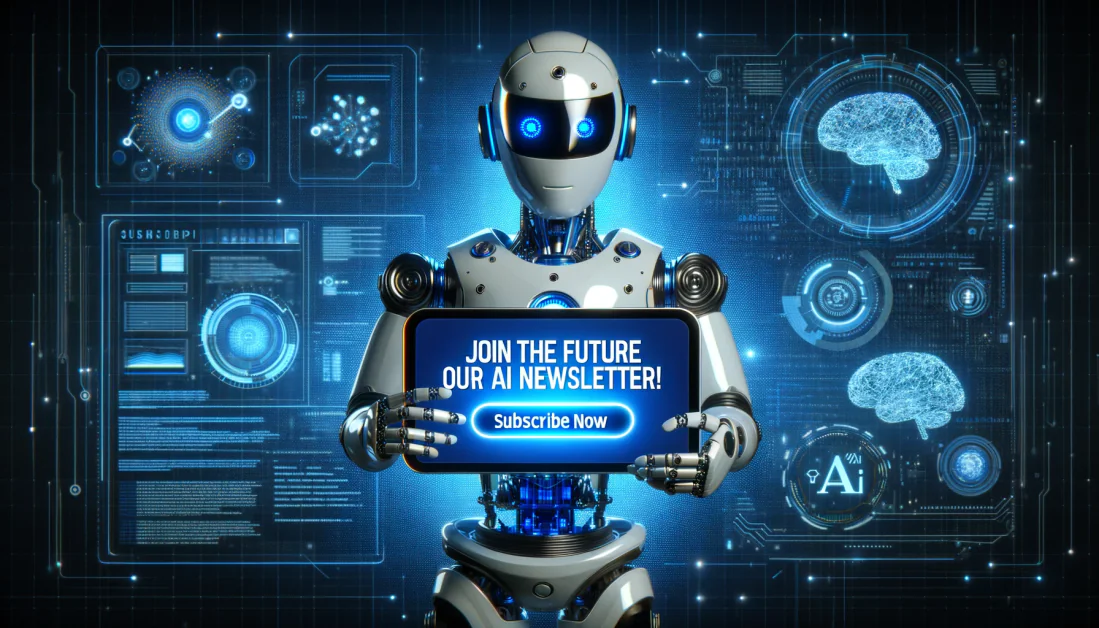
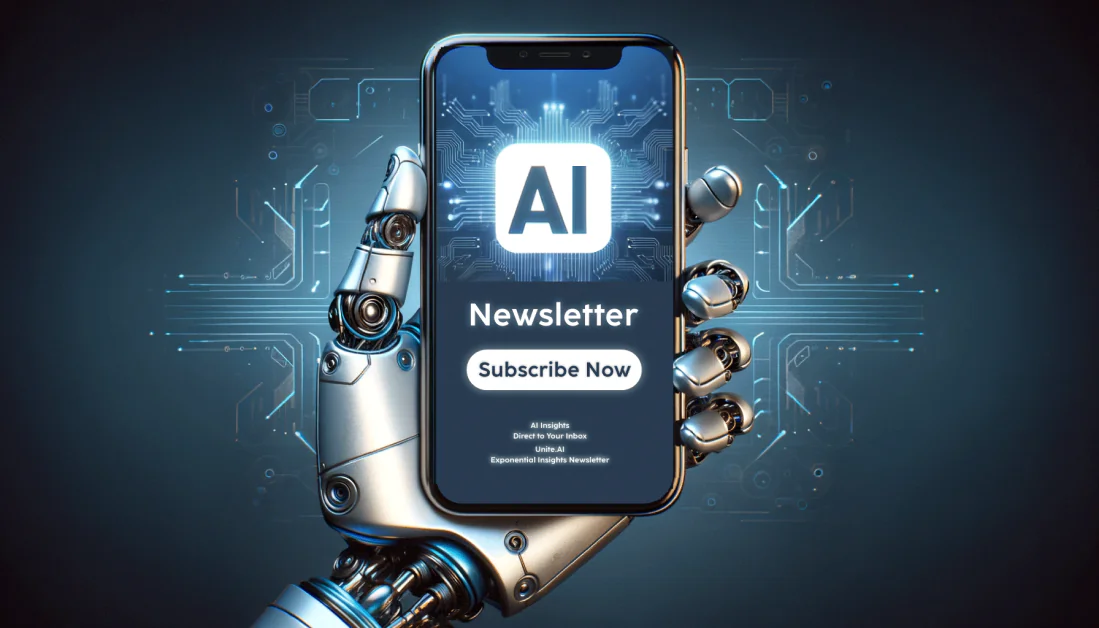