Healthcare
How AI Is Being Used In The Fight Against The Wuhan Coronavirus
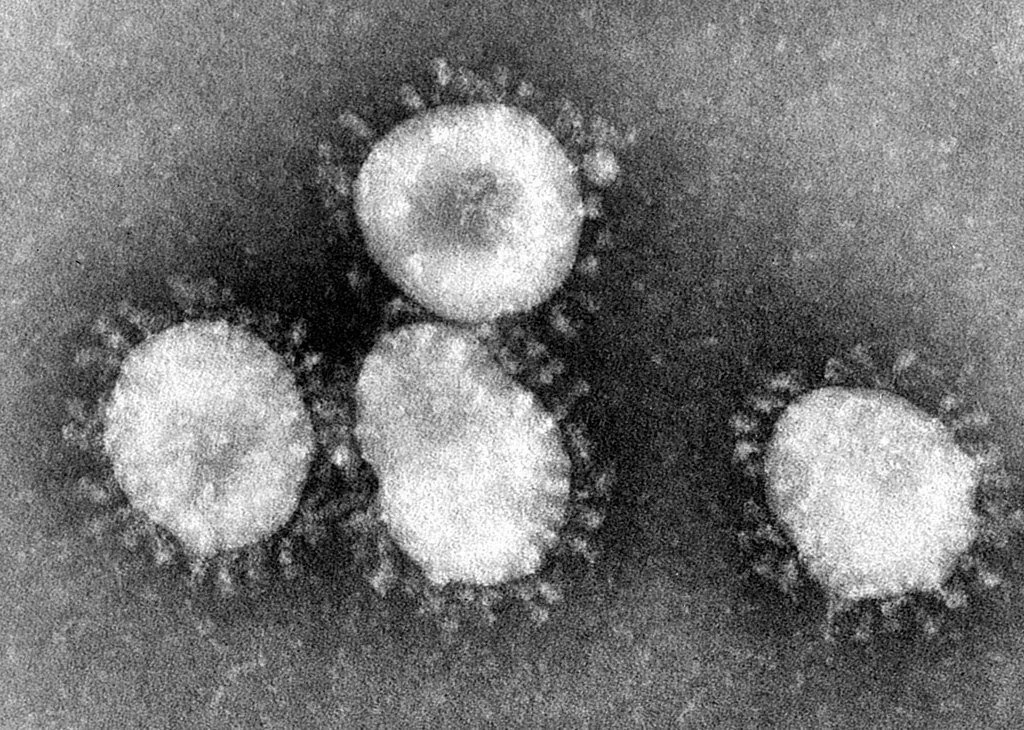
Artificial intelligence is being leveraged in the fight against the Wuhan Coronavirus. Artificial intelligence as being employed by researchers track the spread of the disease and to research potential treatments for the virus.
The Wuhan Coronavirus manifested in China in December, and in the two months since then it has spread across China and to other parts of the globe. It's still unknown just how contagious the virus is and how quickly the virus could spread, although there are currently more than 40,000 confirmed cases within China. In order to get a better understanding of how the virus might spread and how fast the virus can spread, researchers are employing machine learning algorithms focused on data pulled from social media sites and other parts of the web.
Over the course of the past week, the rate of infection seems to have decreased somewhat, but it's unclear if the disease is falling under control or if new cases are becoming harder to detect. While other countries around the world have only seen a few cases of coronavirus, in comparison to China, the world health community remains concerned about the virus's ability to spread. Researchers are trying to get ahead of the viruses’ spread by using machine learning and big data collected from the internet.
As reported by Wired, an international team of researchers have extracted data from various parts of the internet, including posts from doctors and medical groups, public health channels, social media posts, and news reports, compiling a database of text that might relate to the coronavirus. The researchers then analyze the data for signs that the virus could be spreading outside of China's borders, making use of machine learning techniques in order to find relevant patterns in the data that could hint at how the virus is behaving.
The researchers sift through social media posts looking for potential symptoms of coronavirus, centering their search on regions where doctors think cases may manifest. The social media posts are processed using natural language processing techniques, techniques which can distinguish between posts where a person mentions their own symptoms versus someone saying symptom-related words in another context (such as discussing news about the coronavirus).
According to Alessandro Vespignani, as Wired reported, Northeastern University professor and expert contagion analyst, argues that even with advanced machine learning techniques it’s often difficult to track the spread of the virus because the characteristics of the virus are still somewhat unknown, and most social media posts are from media companies and currently about the outbreak in China. However, Vesignani believes that if the virus ever did take hold in the US it would become easier to monitor thanks to more posts concerning the virus.
Despite the challenge in gaining relevant information about the potential behavior of the coronavirus, the model created by the researchers does seem to be reasonably effective at finding clues within a large sea of social media posts. The model used by the researchers was able to find evidence of a viral outbreak on December 30th, although it took time to determine just how serious the situation would become. Crowdsourced information could improve the effectiveness of disease tracking models even further, as it enables the more efficient collection of relevant data regarding the virus. As an example, an analysis of data crowdsourced by Chinese physicians suggests that people younger than 15 years of age are more resilient to the virus.
Artificial intelligence can also be combined with data collected from mobile devices to build models that can potentially predict the direction a virus is spreading as well as the rate of a spread. For instance, Researchers from University of Southampton used mobile data to determine the path that the virus may have taken as it moved out of Wuhan in the days following its manifestation. Other researchers analyzed data collected by Tencent, a Chinese mobile app developer, and found that the restrictions imposed by the Chinese government potentially reduce the virus’ spread, buying vital time to develop a plan of attack.
As Fortune reported, the startup Insilico Medicine has made use of artificial intelligence to identify molecules that could potentially treat the coronavirus. Insilico’s AI identified thousands of possible drug molecules over the course of four days. Insilico explained that the 100 most promising candidates will be synthesized and all of their research on molecular structures will be published for other researchers to take advantage of. Medical researchers and companies are fast-tracking the development and testing of treatments, with the US-based biotech company Gilead planning to start the immediate testing of a new antiviral drug within the Wuhan region.
After Insilico decided to begin researching treatments, it focused its research on an enzyme called 3C-like protease. The coronavirus relies on this enzyme to reproduce and spread. According to Insilico, it decided on this specific enzyme because it’s quite similar to other viral proteases whose structures have already been documented, and because Shanghai Tech University had developed a model of the 2019-nCoV 3C-like protease. In the span of four days Insilico was able to generate hundreds of thousands of candidate molecules and choose only the hundred or so that were most likely to be useful. The results of the research were recently published in the repository bioRxiv and on Insilico’s website.
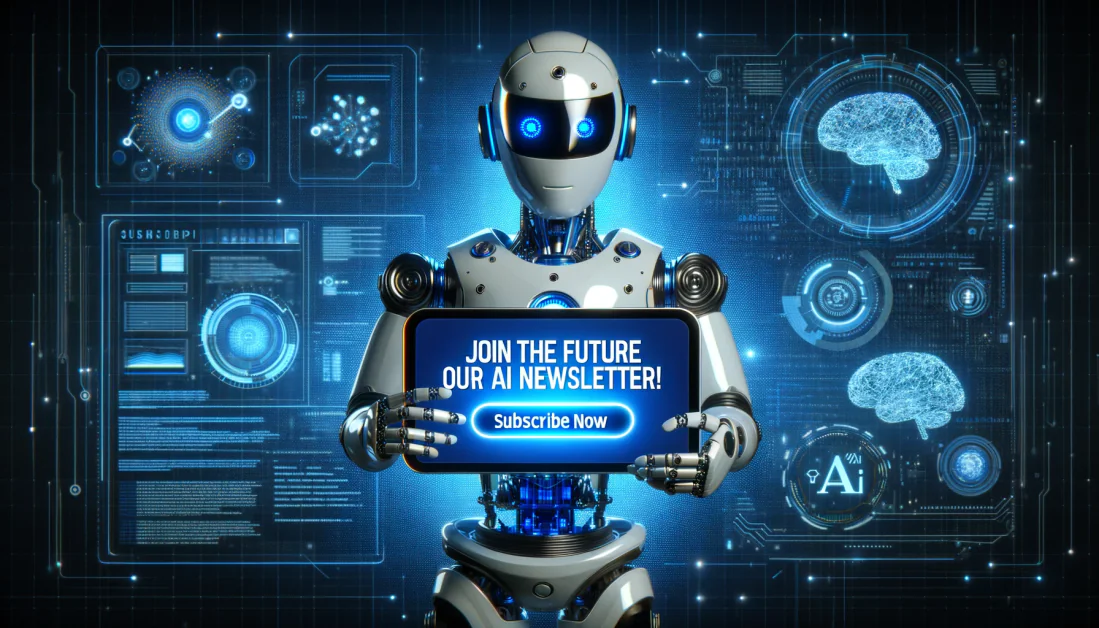
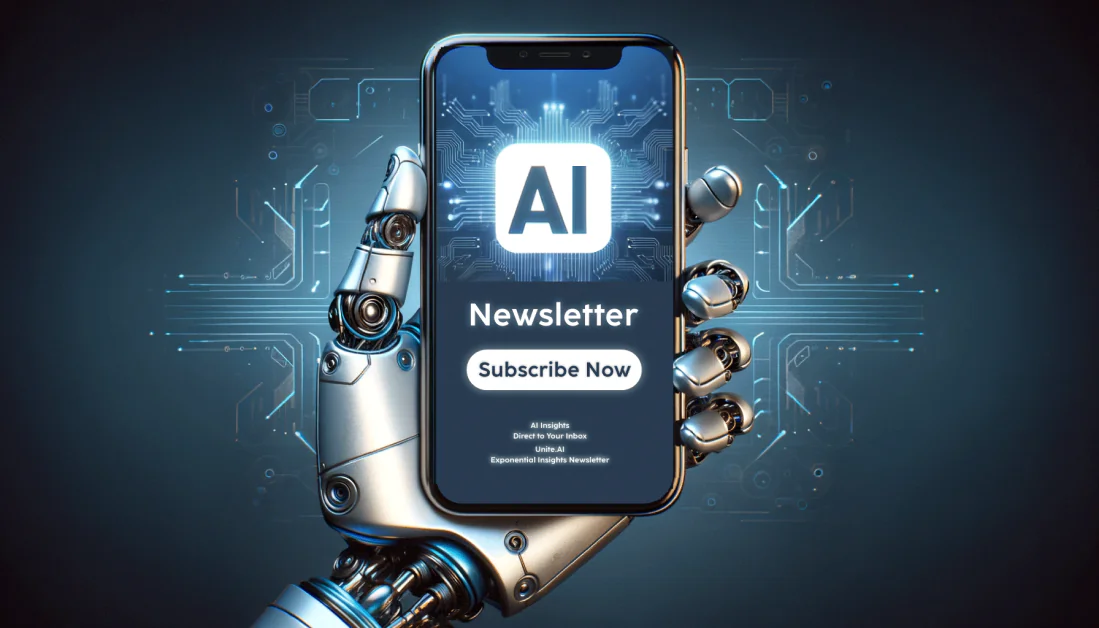