Healthcare
AI Being Used to Analyze Retinal Images
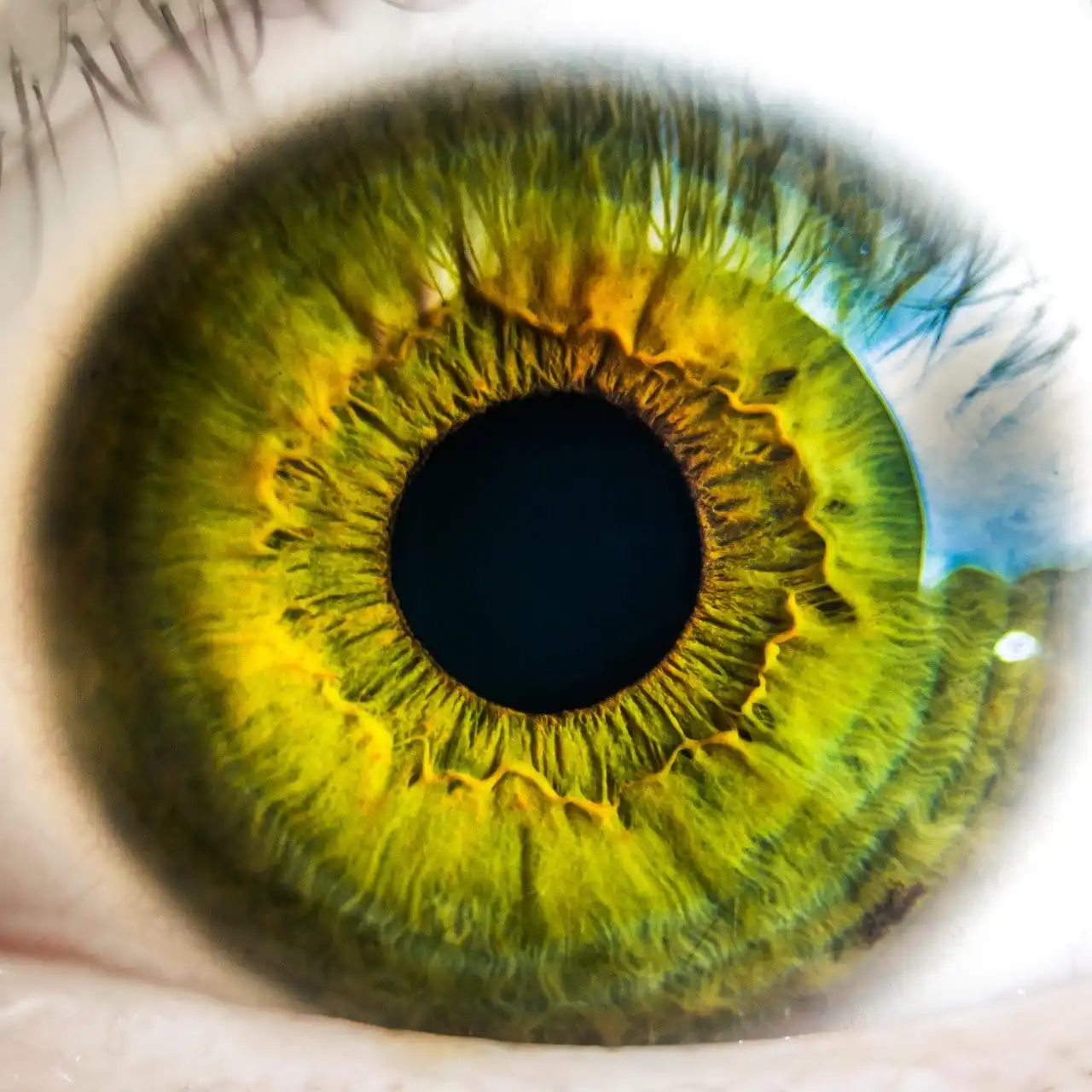
In a newly developed approach, artificial intelligence (AI) is being used to analyze retinal images. The system could be used by doctors in order to select the best treatment for patients suffering from vision loss from diabetic macular edema, a diabetes complication. That problem often leads to vision loss among working-age adults.
One of the first types of therapy that is often used as a line of defense against diabetic macular edema is anti-vascular endothelial growth factor (VEGF). The problem with VEGF agents is that they do not work for everyone. Those who could benefit from the therapy need to be identified first since it requires multiple injections. Those injections cost a lot, and they are burdensome for both patients and physicians.
The leader of the research team is Sina Farsiu from Duke University.
“We developed an algorithm that can be used to automatically analyze optical coherence tomography (OCT) images of the retina to predict whether a patient is likely to respond to anti-VEGF treatments,” she said. “This research represents a step toward precision medicine, in which such predictions help clinicians better select first-line therapies for patients based on specific disease conditions.”
The work was published in The Optical Society (OSA) journal Biomedical Optics Express. In the journal, Farsiu and her team demonstrated how the new algorithm is capable of accurately predicting whether a patient is likely to respond to anti-VEGF therapy, after just one volumetric scan.
“Our approach could potentially be used in eye clinics to prevent unnecessary and costly trial-and-error treatments and thus alleviate a substantial treatment burden for patients,” Farsiu said. “The algorithm could also be adapted to predict therapy response for many other eye diseases, including neovascular age-related macular degeneration.”
The newly developed algorithm is based on a novel convolutional neural network (CNN) architecture. A CNN is a type of artificial intelligence, and it assigns importance to various aspects or objects in order to analyze images. The algorithm was used by the researchers to examine images acquired with OCT, which is a noninvasive technology. OCT produces high-resolution cross-sectional retinal images, and it is considered the standard of care for the assessment and treatment of various eye conditions.
“Unlike previously developed approaches, our algorithm requires OCT images from only a single pretreatment timepoint,” said Reza Rasti, first author of the paper and a postdoctoral scholar in Farsiu's laboratory. “There's no need for time-series OCT images, patient records or other metadata to predict therapy response.”
The new algorithm works by highlighting global structures in the OCT. At the same time, it also enhances local features from diseased regions. It searches for CNN-encoded features that can be correlated with anti-VEGF response.
The algorithm was tested with OCT images from 127 patients who had undergone treatment for diabetic macular edema with three consecutive injections of anti-VEGF agents. The algorithm then analyzed OCT images that were taken prior to the anti-VEGF injections, and the algorithm's predictions were compared to OCT images taken after anti-VEGF therapy. This told researchers whether or not the therapy resulted in an improvement of the condition.
The algorithm was found to have an 87 percent accuracy rate for predicting those who would respond to treatment. It had an average precision and specificity of 85 percent and a sensitivity of 80 percent.
The researchers now want to confirm the findings and undertake a larger observational trial of patients who have yet to go through treatment.
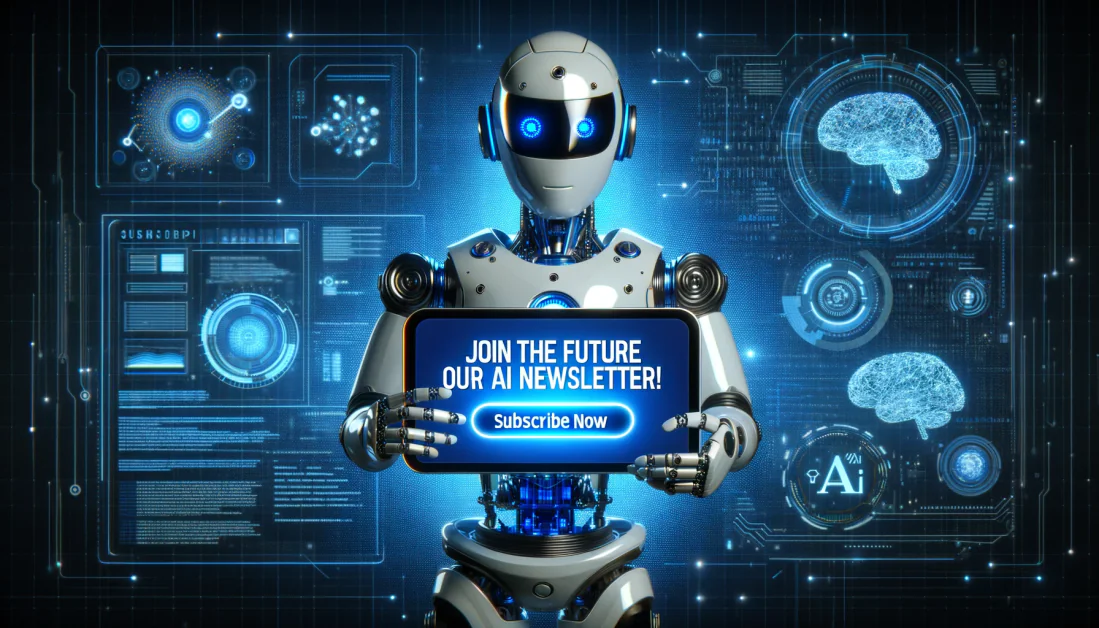
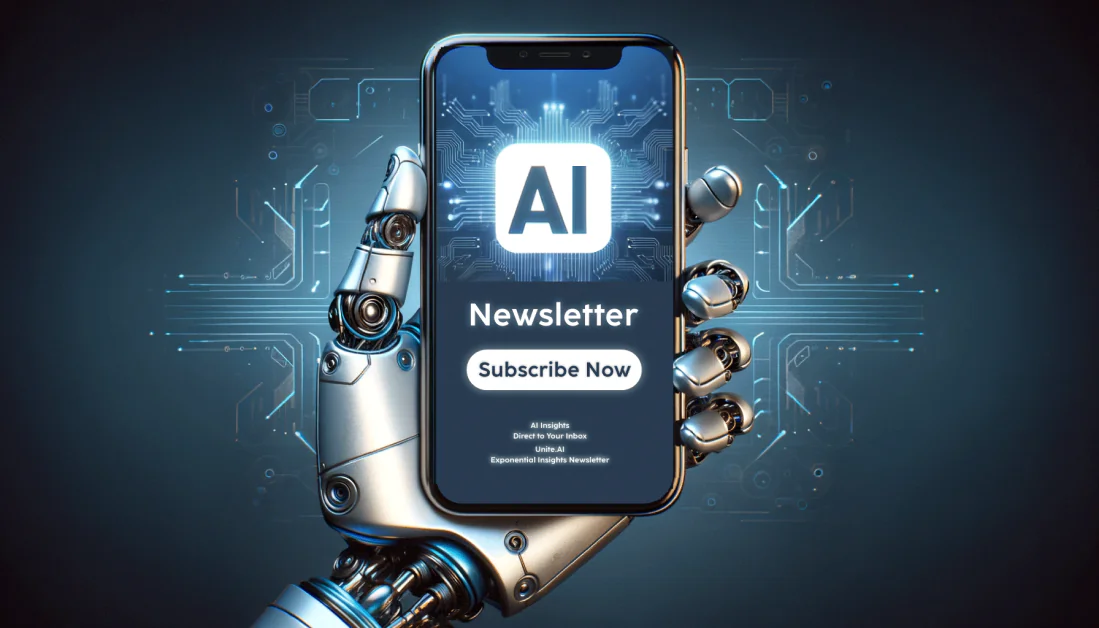