Thought Leaders
Expert Predictions For AI’s Trajectory In 2020
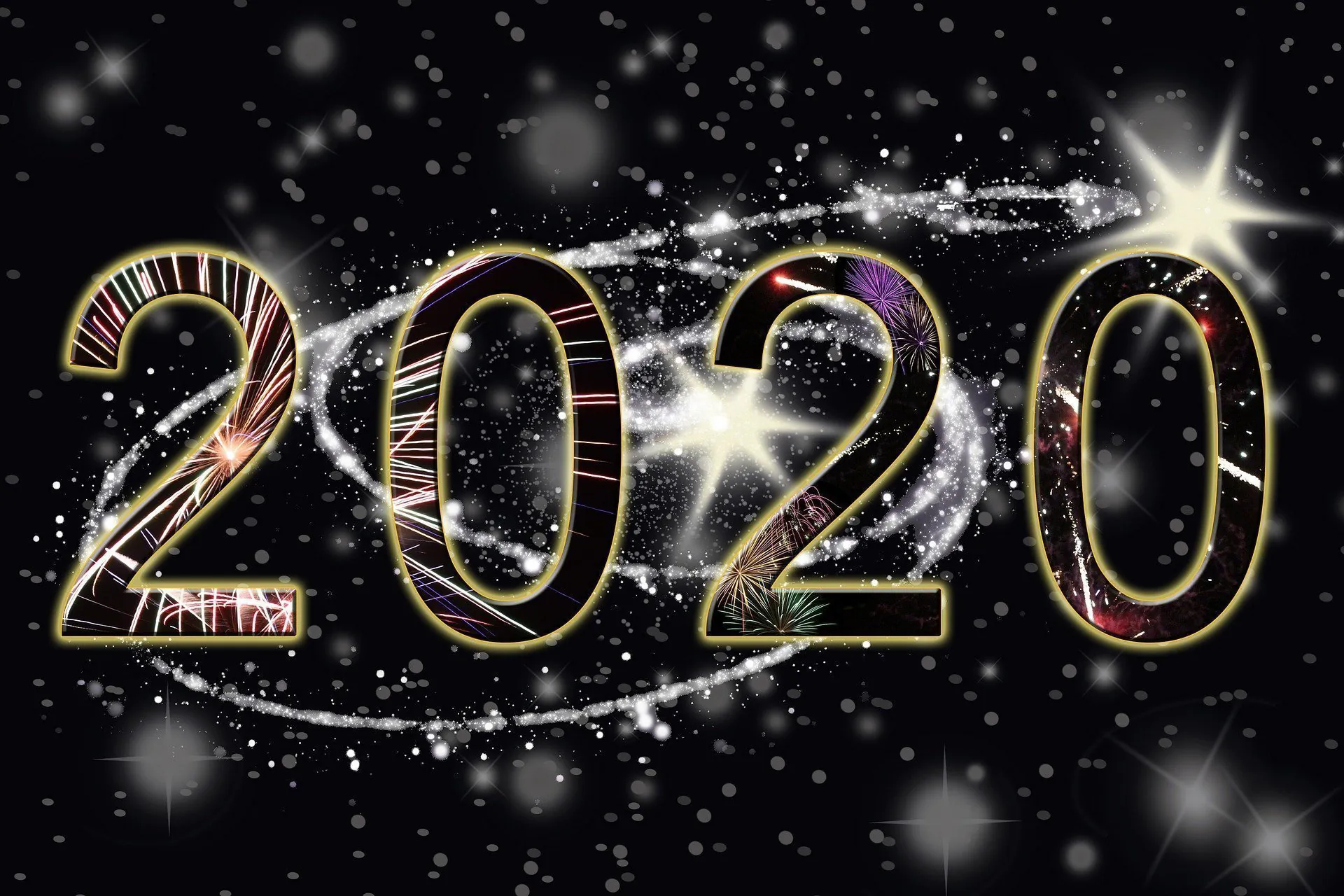
VentureBeat recently interviewed five of the most intelligent, expert minds in the AI field and asked them to make their predictions for where AI is heading over the course of the year to come. The individuals interviewed for their predictions were:
- Soumith Chintala, creator of PyTorch.
- Celeste Kidd, AI professor at the University of California.
- Jeff Dean, chief of Google AI.
- Anima Anandkumar, machine learning research director at Nvidia.
- Dario Gil, IBM Research director.
Soumith Chintala
Chintala, the creator of Pytorch, which is arguably the most popular machine learning framework at the moment, predicted that 2020 will see a greater need for neural network hardware accelerators and methods of boosting model training speeds. Chintala expected that the next couple of years will see an increased focus on how to use GPUs optimally and how compiling can be done automatically for new hardware. Beyond this, Chintala expected that the AI community will begin pursuing other methods of quantifying AI performance more aggressively, placing less importance on pure accuracy. Factors for consideration include things like the amount of energy needed to train a model, how AI can be used to build the sort of society we want, and how the output of a network can be intuitively explained to human operators.
Celeste Kidd
Celeste Kidd has spent much of her recent career advocating for more responsibility on the part of designers of algorithms, tech platforms, and content recommendation systems. Kidd has often argued that systems that are designed to maximize engagement can end up having serious impacts regarding how people create their opinions and beliefs. More and more attention is being paid to the ethical use of AI algorithms and systems, and Kidd predicted that in 2020 there will be an increased awareness of how tech tools and platforms are influencing people’s lives and decisions, as well as a rejection of the idea that tech tools can be genuinely neutral in design.
“We really need to, as a society and especially as the people that are working on these tools, directly appreciate the responsibility that that comes with,” Kidd said.
Jeff Dean
Jeff Dean, the current head of Google AI, predicted that in 2020 there will be progress in multimodal learning and multitask learning. Multimodel learning is when AI is trained with multiple types of media at one time, while multitask learning endeavors to allow AI to train on multiple tasks at one time. Dean also expected further progress to be made regarding natural language processing models based on Transformer, such as Google’s BERT algorithm and the other models that topped the GLUE leaderboards. Dean also mentioned he would like to see less desire to create the most-advanced state-of-the-art performance models and more desire to create models that are more robust and flexible.
Anima Anandkumar
Anandkumar expected that the AI community will have to grapple with many challenges in 2020, especially the need for more diverse datasets and the need to ensure people’s privacy when training on data. Anandkumar explained that while face recognition often gets the most attention, there are many areas where people’s privacy can be violated and that these issues may come to the forefront of discussion during 2020.
Anandkumar also expected that further advancements will be made regarding Transformer based natural language processing models.
“We are still not at the stage of dialogue generation that’s interactive, that can keep track and have natural conversations. So I think there will be more serious attempts made in 2020 in that direction,” she said.
Finally, Anandkumar expected that the coming year will see more development of the iterative algorithm and self-supervision. These training methods allow AI systems to self-train in some respects, and can potentially help create models that can improve by self-training on data that are unlabeled.
Dario Gil
Gil predicted that in 2020 there will be more progress towards creating AI in a more computationally efficient manner, as the way deep neural networks are currently trained is inefficient in many ways. Because of this, Gil expected that this year will see progress in terms of creating reduced-precision architectures and generally training more efficiently. Much like some of the other experts who were interviewed, Gil predicted that in 2020 researchers will start to focus more on metrics aside from accuracy. Gil expressed an interest in neural symbolic AI, as IBM is examining ways to create probabilistic programming models using neural symbolic approaches. Finally, Gil emphasized the importance of making AI more accessible to those interested in machine learning and getting rid of the perception that only geniuses can work with AI and do data science.
“If we leave it as some mythical realm, this field of AI, that’s only accessible to the select PhDs that work on this, it doesn’t really contribute to its adoption,” Gil said.
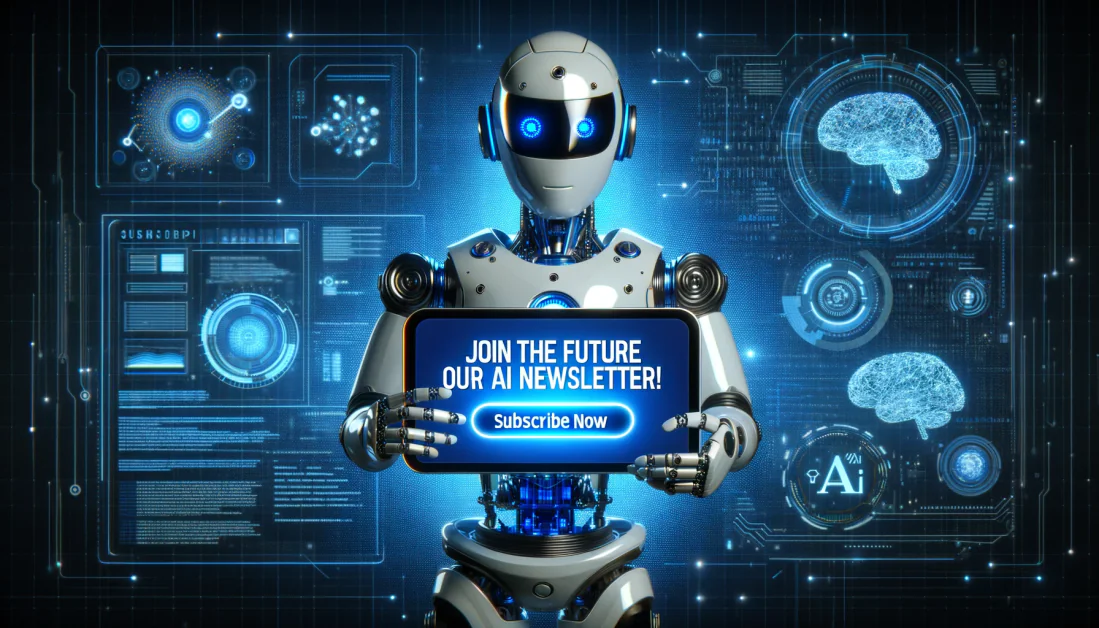
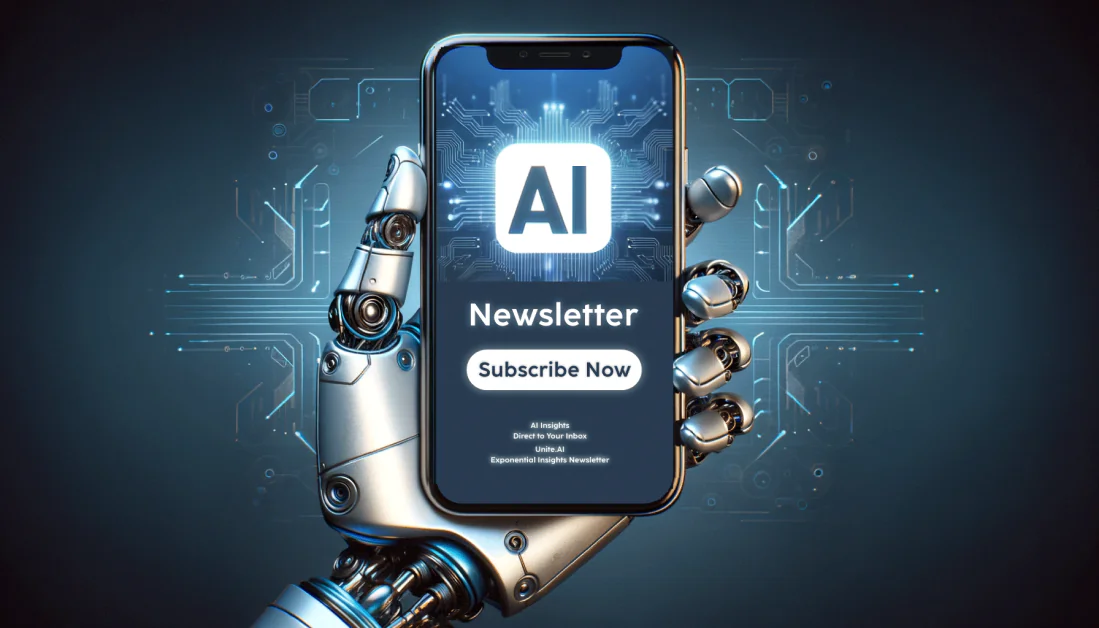